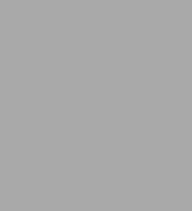
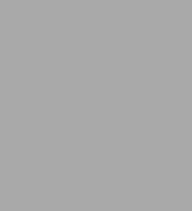
Paperback(5th ed.)
-
SHIP THIS ITEMTemporarily Out of Stock Online
-
PICK UP IN STORE
Your local store may have stock of this item.
Available within 2 business hours
Related collections and offers
Overview
Product Details
ISBN-13: | 9781119401155 |
---|---|
Publisher: | Wiley |
Publication date: | 07/31/2017 |
Edition description: | 5th ed. |
Pages: | 520 |
Product dimensions: | 7.00(w) x 10.00(h) x 0.80(d) |
About the Author
Read an Excerpt
A Guide to Modern Econometrics
By Marno Verbeek
John Wiley & Sons
ISBN: 0-470-85773-0Chapter One
Introduction
1.1 About Econometrics
Economists are frequently interested in relationships between different quantities, for example between individual wages and the level of schooling. The most important job of econometrics is to quantify these relationships on the basis of available data and using statistical techniques, and to interpret, use or exploit the resulting outcomes appropriately. Consequently, econometrics is the interaction of economic theory, observed data and statistical methods. It is the interaction of these three that makes econometrics interesting, challenging and, perhaps, difficult. In the words of a seminar speaker, several years ago: 'Econometrics is much easier without data'.
Traditionally econometrics has focused upon aggregate economic relationships. Macro-economic models consisting of several up to many hundreds equations were specified, estimated and used for policy evaluation and forecasting. The recent theoretical developments in this area, most importantly the concept of cointegration, have generated increased attention to the modelling of macro-economic relationships and their dynamics, although typically focusing on particular aspects of the economy. Since the 1970s econometric methods are increasingly employed in micro-economic models describing individual, household or firm behaviour, stimulated by the development of appropriateeconometric models and estimators which take into account problems like discrete dependent variables and sample selection, by the availability of large survey data sets, and by the increasing computational possibilities. More recently, the empirical analysis of financial markets has required and stimulated many theoretical developments in econometrics. Currently econometrics plays a major role in empirical work in all fields of economics, almost without exception, and in most cases it is no longer sufficient to be able to run a few regressions and interpret the results. As a result, introductory econometrics textbooks usually provide insufficient coverage for applied researchers. On the other hand, the more advanced econometrics textbooks are often too technical or too detailed for the average economist to grasp the essential ideas and to extract the information that is needed. Thus there is a need for an accessible textbook that discusses the recent and relatively more advanced developments.
The relationships that economists are interested in are formally specified in mathematical terms, which lead to econometric or statistical models. In such models there is room for deviations from the strict theoretical relationships due to, for example, measurement errors, unpredictable behaviour, optimization errors or unexpected events. Broadly, econometric models can be classified in a number of categories.
A first class of models describes relationships between present and past. For example, how does the short-term interest rate depend on its own history? This type of model, typically referred to as a time series model, usually lacks any economic theory and is mainly built to get forecasts for future values and the corresponding uncertainty or volatility.
A second type of model considers relationships between economic quantities over a certain time period. These relationships give us information on how (aggregate) economic quantities fluctuate over time in relation to other quantities. For example, what happens to the long-term interest rate if the monetary authority adjusts the short-term one? These models often give insight into the economic processes that are operating.
Third, there are models that describe relationships between different variables measured at a given point in time for different units (for example households or firms). Most of the time, this type of relationship is meant to explain why these units are different or behave differently. For example, one can analyse to what extent differences in household savings can be attributed to differences in household income. Under particular conditions, these cross-sectional relationships can be used to analyse 'what if' questions. For example, how much more would a given household, or the average household, save if income would increase by 1%?
Finally, one can consider relationships between different variables measured for different units over a longer time span (at least two periods). These relationships simultaneously describe differences between different individuals (why does person 1 save much more than person 2?), and differences in behaviour of a given individual over time (why does person 1 save more in 1992 than in 1990?). This type of model usually requires panel data, repeated observations over the same units. They are ideally suited for analysing policy changes on an individual level, provided that it can be assumed that the structure of the model is constant into the (near) future.
The job of econometrics is to specify and quantify these relationships. That is, econometricians formulate a statistical model, usually based on economic theory, confront it with the data, and try to come up with a specification that meets the required goals. The unknown elements in the specification, the parameters, are estimated from a sample of available data. Another job of the econometrician is to judge whether the resulting model is 'appropriate'. That is, check whether the assumptions made to motivate the estimators (and their properties) are correct, and check whether the model can be used for what it is made for. For example, can it be used for prediction or analysing policy changes? Often, economic theory implies that certain restrictions apply to the model that is estimated. For example, (one version of) the efficient market hypothesis implies that stock market returns are not predictable from their own past. An important goal of econometrics is to formulate such hypotheses in terms of the parameters in the model and to test their validity.
The number of econometric techniques that can be used is numerous and their validity often depends crucially upon the validity of the underlying assumptions. This book attempts to guide the reader through this forest of estimation and testing procedures, not by describing the beauty of all possible trees, but by walking through this forest in a structured way, skipping unnecessary side-paths, stressing the similarity of the different species that are encountered, and by pointing out dangerous pitfalls. The resulting walk is hopefully enjoyable and prevents the reader from getting lost in the econometric forest.
1.2 The Structure of this Book
The first part of this book consists of Chapters 2, 3 and 4. Like most textbooks, it starts with discussing the linear regression model and the OLS estimation method. Chapter 2 presents the basics of this important estimation method, with some emphasis on its validity under fairly weak conditions, while Chapter 3 focuses on the interpretation of the models and the comparison of alternative specifications. Chapter 4 considers two particular deviations from the standard assumptions of the linear model: autocorrelation and heteroskedasticity of the error terms. It is discussed how one can test for these phenomena, how they affect the validity of the OLS estimator and how this can be corrected. This includes a critical inspection of the model specification, the use of adjusted standard errors for the OLS estimator and the use of alternative (GLS) estimators. These three chapters are essential for the remaining part of this book and should be the starting point in any course.
In Chapter 5 another deviation from the standard assumptions of the linear model is discussed which is, however, fatal for the OLS estimator. As soon as the error term in the model is correlated with one or more of the explanatory variables all good properties of the OLS estimator disappear and we necessarily have to use alternative estimators. The chapter discusses instrumental variables (IV) estimators and, more generally, the generalized method of moments (GMM). This chapter, at least its earlier sections, is also recommended as an essential part of any econometrics course.
Chapter 6 is mainly theoretical and discusses maximum likelihood (ML) estimation. Because in empirical work maximum likelihood is often criticized for its dependence upon distributional assumptions, it is not discussed in the earlier chapters where alternatives are readily available that are either more robust than maximum likelihood or (asymptotically) equivalent to it. Particular emphasis in Chapter 6 is on misspecification tests based upon the Lagrange multiplier principle. While many empirical studies tend to take the distributional assumptions for granted, their validity is crucial for consistency of the estimators that are employed and should therefore be tested. Often these tests are relatively easy to perform, although most software does not routinely provide them (yet). Chapter 6 is crucial for understanding Chapter 7 on limited dependent variable models and for a small number of sections in Chapters 8 to 10.
The last part of this book contains four chapters. Chapter 7 presents models that are typically (though not exclusively) used in micro-economics, where the dependent variable is discrete (e.g. zero or one), partly discrete (e.g. zero or positive) or a duration. It also includes discussions of the sample selection problem and the estimation of treatment effects that go further than their typical textbook treatment.
Chapters 8 and 9 discuss time series modelling including unit roots, cointegration and error-correction models. These chapters can be read immediately after Chapter 4 or 5, with the exception of a few parts that relate to maximum likelihood estimation. The theoretical developments in this area over the last 20 years have been substantial and many recent textbooks seem to focus upon it almost exclusively. Univariate time series models are covered in Chapter 8. In this case models are developed that explain an economic variable from its own past. This includes ARIMA models, as well as GARCH models for the conditional variance of a series. Multivariate time series models that consider several variables simultaneously are discussed in Chapter 9. This includes vector autoregressive models, cointegration and error-correction models.
Finally, Chapter 10 covers models based on panel data. Panel data are available if we have repeated observations of the same units (for example households, firms or countries). The last decade the use of panel data has become important in many areas of economics. Micro-economic panels of households and firms are readily available and, given the increase in computing resources, more manageable than in the past. In addition, it is more and more common to pool time series of several countries. One of the reasons for this may be that researchers believe that a cross-sectional comparison of countries provides interesting information, in addition to a historical comparison of a country with its own past. This chapter also discusses the recent developments on unit roots and cointegration in a panel data setting.
At the end of the book the reader will find two short appendices discussing mathematical and statistical results that are used at several places in the book. This includes a discussion of some relevant matrix algebra and distribution theory. In particular, a discussion of properties of the (bivariate) normal distribution, including conditional expectations, variances and truncation is provided.
In my experience the material in this book is too much to be covered in a single course. Different courses can be scheduled on the basis of the chapters that follow. For example, a typical graduate course in applied econometrics would cover Chapters 2, 3, 4, parts of Chapter 5, and then continue with selected parts of Chapters 8 and 9 if the focus is on time series analysis, or continue with Section 6.1 and Chapter 7 if the focus is on cross-sectional models. A more advanced undergraduate or graduate course may focus attention to the time series chapters (Chapters 8 and 9), the micro-econometric chapters (Chapters 6 and 7) or panel data (Chapter 10 with some selected parts from Chapters 6 and 7).
Given the focus and length of this book, I had to make many choices of which material to present or not. As a general rule I did not want to bother the reader with details that I considered not essential or do not have empirical relevance. The main goal was to give a general and comprehensive overview of the different methodologies and approaches, focusing on what is relevant for doing and understanding empirical work. Some topics are only very briefly mentioned and no attempt is made to discuss them at any length. To compensate for this I have tried to give references at appropriate places to other, often more advanced, textbooks that do cover these issues.
1.3 Illustrations and Exercises
In most chapters a variety of empirical illustrations is provided in separate sections or subsections. While it is possible to skip these illustrations essentially without losing continuity, these sections do provide important aspects concerning the implementation of the methodology discussed in the preceding text. In addition, I have attempted to provide illustrations that are of economic interest in themselves, using data that are typical for current empirical work and covering a wide range of different areas. This means that most data sets are used in recently published empirical work and are fairly large, both in terms of number of observations and number of variables. Given the current state of computing facilities, it is usually not a problem to handle such large data sets empirically.
Learning econometrics is not just a matter of studying a textbook. Hands-on experience is crucial in the process of understanding the different methods and how and when to implement them. Therefore, readers are strongly encouraged to get their hands dirty and to estimate a number of models using appropriate or inappropriate methods, and to perform a number of alternative specification tests. With modern software becoming more and more user-friendly, the actual computation of even the more complicated estimators and test statistics is often surprisingly simple, sometimes dangerously simple. That is, even with the wrong data, the wrong model and the wrong methodology, programs may come up with results that are seemingly all right. At least some expertise is required to prevent the practitioner from such situations and this book plays an important role in this.
To stimulate the reader to use actual data and estimate some models, almost all data sets used in this text are available through the web site.
Continues...
Excerpted from A Guide to Modern Econometrics by Marno Verbeek Excerpted by permission.
All rights reserved. No part of this excerpt may be reproduced or reprinted without permission in writing from the publisher.
Excerpts are provided by Dial-A-Book Inc. solely for the personal use of visitors to this web site.
Table of Contents
Preface xi1 Introduction 1
1.1 About Econometrics 1
1.2 The Structure of This Book 3
1.3 Illustrations and Exercises 4
2 An Introduction to Linear Regression 6
2.1 Ordinary Least Squares as an Algebraic Tool 7
2.1.1 Ordinary Least Squares 7
2.1.2 Simple Linear Regression 9
2.1.3 Example: Individual Wages 11
2.1.4 Matrix Notation 11
2.2 The Linear Regression Model 12
2.3 Small Sample Properties of the OLS Estimator 15
2.3.1 The Gauss–Markov Assumptions 15
2.3.2 Properties of the OLS Estimator 16
2.3.3 Example: Individual Wages (Continued) 20
2.4 Goodness-of-Fit 20
2.5 Hypothesis Testing 23
2.5.1 A Simple t-Test 23
2.5.2 Example: Individual Wages (Continued) 25
2.5.3 Testing One Linear Restriction 25
2.5.4 A Joint Test of Significance of Regression Coefficients 26
2.5.5 Example: Individual Wages (Continued) 28
2.5.6 The General Case 29
2.5.7 Size, Power and p-Values 30
2.5.8 Reporting Regression Results 32
2.6 Asymptotic Properties of the OLS Estimator 33
2.6.1 Consistency 33
2.6.2 Asymptotic Normality 35
2.6.3 Small Samples and Asymptotic Theory 37
2.7 Illustration: The Capital Asset Pricing Model 39
2.7.1 The CAPM as a Regression Model 40
2.7.2 Estimating and Testing the CAPM 41
2.7.3 The World’s Largest Hedge Fund 43
2.8 Multicollinearity 44
2.8.1 Example: Individual Wages (Continued) 47
2.9 Missing Data, Outliers and Influential Observations 48
2.9.1 Outliers and Influential Observations 48
2.9.2 Robust Estimation Methods 50
2.9.3 Missing Observations 51
2.10 Prediction 53
Wrap-up 54
Exercises 55
3 Interpreting and Comparing Regression Models 60
3.1 Interpreting the Linear Model 60
3.2 Selecting the Set of Regressors 65
3.2.1 Misspecifying the Set of Regressors 65
3.2.2 Selecting Regressors 66
3.2.3 Comparing Non-nested Models 71
3.3 Misspecifying the Functional Form 73
3.3.1 Nonlinear Models 73
3.3.2 Testing the Functional Form 74
3.3.3 Testing for a Structural Break 74
3.4 Illustration: Explaining House Prices 76
3.5 Illustration: Predicting Stock Index Returns 79
3.5.1 Model Selection 80
3.5.2 Forecast Evaluation 82
3.6 Illustration: Explaining Individual Wages 85
3.6.1 Linear Models 85
3.6.2 Loglinear Models 88
3.6.3 The Effects of Gender 91
3.6.4 Some Words of Warning 92
Wrap-up 93
Exercises 94
4 Heteroskedasticity and Autocorrelation 97
4.1 Consequences for the OLS Estimator 98
4.2 Deriving an Alternative Estimator 99
4.3 Heteroskedasticity 100
4.3.1 Introduction 100
4.3.2 Estimator Properties and Hypothesis Testing 103
4.3.3 When the Variances Are Unknown 104
4.3.4 Heteroskedasticity-consistent Standard Errors for OLS 105
4.3.5 Multiplicative Heteroskedasticity 106
4.3.6 Weighted Least Squares with Arbitrary Weights 107
4.4 Testing for Heteroskedasticity 108
4.4.1 Testing for Multiplicative Heteroskedasticity 108
4.4.2 The Breusch–Pagan Test 109
4.4.3 The White Test 109
4.4.4 Which Test? 110
4.5 Illustration: Explaining Labour Demand 110
4.6 Autocorrelation 114
4.6.1 First-order Autocorrelation 116
4.6.2 Unknown ρ 118
4.7 Testing for First-order Autocorrelation 119
4.7.1 Asymptotic Tests 119
4.7.2 The Durbin–Watson Test 120
4.8 Illustration: The Demand for Ice Cream 121
4.9 Alternative Autocorrelation Patterns 124
4.9.1 Higher-order Autocorrelation 124
4.9.2 Moving Average Errors 125
4.10 What to Do When You Find Autocorrelation? 126
4.10.1 Misspecification 126
4.10.2 Heteroskedasticity-and-autocorrelation-consistent Standard Errors for OLS 128
4.11 Illustration: Risk Premia in Foreign Exchange Markets 129
4.11.1 Notation 129
4.11.2 Tests for Risk Premia in the 1-Month Market 131
4.11.3 Tests for Risk Premia Using Overlapping Samples 134
Wrap-up 136
Exercises 136
5 Endogenous Regressors, Instrumental Variables and GMM 139
5.1 A Review of the Properties of the OLS Estimator 140
5.2 Cases Where the OLS Estimator Cannot Be Saved 143
5.2.1 Autocorrelation with a Lagged Dependent Variable 143
5.2.2 Measurement Error in an Explanatory Variable 144
5.2.3 Endogeneity and Omitted Variable Bias 146
5.2.4 Simultaneity and Reverse Causality 148
5.3 The Instrumental Variables Estimator 150
5.3.1 Estimation with a Single Endogenous Regressor and a Single Instrument 150
5.3.2 Back to the Keynesian Model 155
5.3.3 Back to the Measurement Error Problem 156
5.3.4 Multiple Endogenous Regressors 156
5.4 Illustration: Estimating the Returns to Schooling 157
5.5 Alternative Approaches to Estimate Causal Effects 162
5.6 The Generalized Instrumental Variables Estimator 163
5.6.1 Multiple Endogenous Regressors with an Arbitrary Number of Instruments 163
5.6.2 Two-stage Least Squares and the Keynesian Model Again 167
5.6.3 Specification Tests 168
5.6.4 Weak Instruments 169
5.6.5 Implementing and Reporting Instrumental Variables Estimators 170
5.7 Institutions and Economic Development 171
5.8 The Generalized Method of Moments 175
5.8.1 Example 175
5.8.2 The Generalized Method of Moments 177
5.8.3 Some Simple Examples 179
5.8.4 Weak Identification 180
5.9 Illustration: Estimating Intertemporal Asset Pricing Models 181
Wrap-up 184
Exercises 185
6 Maximum Likelihood Estimation and Specification Tests 187
6.1 An Introduction to Maximum Likelihood 188
6.1.1 Some Examples 188
6.1.2 General Properties 191
6.1.3 An Example (Continued) 194
6.1.4 The Normal Linear Regression Model 195
6.1.5 The Stochastic Frontier Model 197
6.2 Specification Tests 198
6.2.1 Three Test Principles 198
6.2.2 Lagrange Multiplier Tests 200
6.2.3 An Example (Continued) 203
6.3 Tests in the Normal Linear Regression Model 204
6.3.1 Testing for Omitted Variables 204
6.3.2 Testing for Heteroskedasticity 206
6.3.3 Testing for Autocorrelation 207
6.4 Quasi-maximum Likelihood and Moment Conditions Tests 208
6.4.1 Quasi-maximum Likelihood 208
6.4.2 Conditional Moment Tests 210
6.4.3 Testing for Normality 211
Wrap-up 212
Exercises 212
7 Models with Limited Dependent Variables 215
7.1 Binary Choice Models 216
7.1.1 Using Linear Regression? 216
7.1.2 Introducing Binary Choice Models 216
7.1.3 An Underlying Latent Model 219
7.1.4 Estimation 219
7.1.5 Goodness-of-Fit 221
7.1.6 Illustration: The Impact of Unemployment Benefits on Recipiency 223
7.1.7 Specification Tests in Binary Choice Models 226
7.1.8 Relaxing Some Assumptions in Binary Choice Models 228
7.2 Multiresponse Models 229
7.2.1 Ordered Response Models 230
7.2.2 About Normalization 231
7.2.3 Illustration: Explaining Firms’ Credit Ratings 231
7.2.4 Illustration: Willingness to Pay for Natural Areas 234
7.2.5 Multinomial Models 237
7.3 Models for Count Data 240
7.3.1 The Poisson and Negative Binomial Models 240
7.3.2 Illustration: Patents and R&D Expenditures 244
7.4 Tobit Models 246
7.4.1 The Standard Tobit Model 247
7.4.2 Estimation 249
7.4.3 Illustration: Expenditures on Alcohol and Tobacco (Part 1) 250
7.4.4 Specification Tests in the Tobit Model 253
7.5 Extensions of Tobit Models 256
7.5.1 The Tobit II Model 256
7.5.2 Estimation 259
7.5.3 Further Extensions 261
7.5.4 Illustration: Expenditures on Alcohol and Tobacco (Part 2) 262
7.6 Sample Selection Bias 265
7.6.1 The Nature of the Selection Problem 266
7.6.2 Semi-parametric Estimation of the Sample Selection Model 268
7.7 Estimating Treatment Effects 269
7.7.1 Regression-based Estimators 271
7.7.2 Regression Discontinuity Design 274
7.7.3 Weighting and Matching 276
7.8 Duration Models 278
7.8.1 Hazard Rates and Survival Functions 278
7.8.2 Samples and Model Estimation 281
7.8.3 Illustration: Duration of Bank Relationships 283
Wrap-up 284
Exercises 285
8 Univariate Time Series Models 288
8.1 Introduction 289
8.1.1 Some Examples 289
8.1.2 Stationarity and the Autocorrelation Function 291
8.2 General ARMA Processes 294
8.2.1 Formulating ARMA Processes 294
8.2.2 Invertibility of Lag Polynomials 297
8.2.3 Common Roots 298
8.3 Stationarity and Unit Roots 299
8.4 Testing for Unit Roots 301
8.4.1 Testing for Unit Roots in a First-order Autoregressive Model 301
8.4.2 Testing for Unit Roots in Higher-Order Autoregressive Models 304
8.4.3 Extensions 306
8.4.4 Illustration: Stock Prices and Earnings 307
8.5 Illustration: Long-run Purchasing Power Parity (Part 1) 309
8.6 Estimation of ARMA Models 313
8.6.1 Least Squares 314
8.6.2 Maximum Likelihood 315
8.7 Choosing a Model 316
8.7.1 The Autocorrelation Function 316
8.7.2 The Partial Autocorrelation Function 318
8.7.3 Diagnostic Checking 319
8.7.4 Criteria for Model Selection 319
8.8 Illustration: The Persistence of Inflation 320
8.9 Forecasting with ARMA Models 324
8.9.1 The Optimal Forecast 324
8.9.2 Forecast Accuracy 327
8.9.3 Evaluating Forecasts 329
8.10 Illustration: The Expectations Theory of the Term Structure 330
8.11 Autoregressive Conditional Heteroskedasticity 335
8.11.1 ARCH and GARCH Models 335
8.11.2 Estimation and Prediction 338
8.11.3 Illustration: Volatility in Daily Exchange Rates 340
8.12 What about Multivariate Models? 342
Wrap-up 343
Exercises 344
9 Multivariate Time Series Models 348
9.1 Dynamic Models with Stationary Variables 349
9.2 Models with Nonstationary Variables 352
9.2.1 Spurious Regressions 352
9.2.2 Cointegration 353
9.2.3 Cointegration and Error-correction Mechanisms 356
9.3 Illustration: Long-run Purchasing Power Parity (Part 2) 358
9.4 Vector Autoregressive Models 360
9.5 Cointegration: the Multivariate Case 364
9.5.1 Cointegration in a VAR 364
9.5.2 Example: Cointegration in a Bivariate VAR 366
9.5.3 Testing for Cointegration 367
9.5.4 Illustration: Long-run Purchasing Power Parity (Part 3) 370
9.6 Illustration: Money Demand and Inflation 372
Wrap-up 378
Exercises 379
10 Models Based on Panel Data 382
10.1 Introduction to Panel Data Modelling 383
10.1.1 Efficiency of Parameter Estimators 384
10.1.2 Identification of Parameters 385
10.2 The Static Linear Model 386
10.2.1 The Fixed Effects Model 386
10.2.2 The First-difference Estimator 388
10.2.3 The Random Effects Model 390
10.2.4 Fixed Effects or Random Effects? 394
10.2.5 Goodness-of-Fit 395
10.2.6 Alternative Instrumental Variables Estimators 396
10.2.7 Robust Inference 398
10.2.8 Testing for Heteroskedasticity and Autocorrelation 400
10.2.9 The Fama–MacBeth Approach 402
10.3 Illustration: Explaining Individual Wages 403
10.4 Dynamic Linear Models 405
10.4.1 An Autoregressive Panel Data Model 406
10.4.2 Dynamic Models with Exogenous Variables 411
10.4.3 Too Many Instruments 412
10.5 Illustration: Explaining Capital Structure 414
10.6 Panel Time Series 419
10.6.1 Heterogeneity 420
10.6.2 First Generation Panel Unit Root Tests 421
10.6.3 Second Generation Panel Unit Root Tests 424
10.6.4 Panel Cointegration Tests 425
10.7 Models with Limited Dependent Variables 426
10.7.1 Binary Choice Models 427
10.7.2 The Fixed Effects Logit Model 428
10.7.3 The Random Effects Probit Model 429
10.7.4 Tobit Models 431
10.7.5 Dynamics and the Problem of Initial Conditions 431
10.7.6 Semi-parametric Alternatives 433
10.8 Incomplete Panels and Selection Bias 433
10.8.1 Estimation with Randomly Missing Data 434
10.8.2 Selection Bias and Some Simple Tests 436
10.8.3 Estimation with Nonrandomly Missing Data 438
10.9 Pseudo Panels and Repeated Cross-sections 439
10.9.1 The Fixed Effects Model 440
10.9.2 An Instrumental Variables Interpretation 441
10.9.3 Dynamic Models 442
Wrap-up 444
Exercises 445
A Vectors and Matrices 450
A.1 Terminology 450
A.2 Matrix Manipulations 451
A.3 Properties of Matrices and Vectors 452
A.4 Inverse Matrices 453
A.5 Idempotent Matrices 454
A.6 Eigenvalues and Eigenvectors 454
A.7 Differentiation 455
A.8 Some Least Squares Manipulations 456
B Statistical and Distribution Theory 458
B.1 Discrete Random Variables 458
B.2 Continuous Random Variables 459
B.3 Expectations and Moments 460
B.4 Multivariate Distributions 461
B.5 Conditional Distributions 462
B.6 The Normal Distribution 463
B.7 Related Distributions 466
Bibliography 468
Index 488