Researchers in the social sciences are faced with complex data sets in which they have relatively small samples and many variables (high dimensional data). Unlike the various technical guides currently on the market, Applied Regularization Methods for the Social Sciences provides and overview of a variety of models alongside clear examples of hands-on application. Each chapter in this book covers a specific application of regularization techniques with a user-friendly technical description, followed by examples that provide a thorough demonstration of the methods in action.
"1140172292"
Key Features:
- Description of regularization methods in a user friendly and easy to read manner
- Inclusion of regularization-based approaches for a variety of statistical analyses commonly used in the social sciences, including both univariate and multivariate models
- Fully developed extended examples using multiple software packages, including R, SAS, and SPSS
- Website containing all datasets and software scripts used in the examples
- Inclusion of both frequentist and Bayesian regularization approaches
- Application exercises for each chapter that instructors could use in class, and independent researchers could use to practice what they have learned from the book
Applied Regularization Methods for the Social Sciences
Researchers in the social sciences are faced with complex data sets in which they have relatively small samples and many variables (high dimensional data). Unlike the various technical guides currently on the market, Applied Regularization Methods for the Social Sciences provides and overview of a variety of models alongside clear examples of hands-on application. Each chapter in this book covers a specific application of regularization techniques with a user-friendly technical description, followed by examples that provide a thorough demonstration of the methods in action.
Key Features:
- Description of regularization methods in a user friendly and easy to read manner
- Inclusion of regularization-based approaches for a variety of statistical analyses commonly used in the social sciences, including both univariate and multivariate models
- Fully developed extended examples using multiple software packages, including R, SAS, and SPSS
- Website containing all datasets and software scripts used in the examples
- Inclusion of both frequentist and Bayesian regularization approaches
- Application exercises for each chapter that instructors could use in class, and independent researchers could use to practice what they have learned from the book
59.99
In Stock
5
1
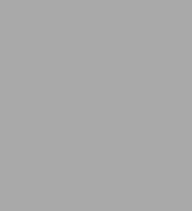
Applied Regularization Methods for the Social Sciences
305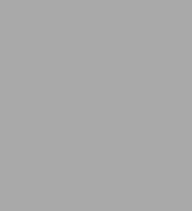
Applied Regularization Methods for the Social Sciences
305
59.99
In Stock
Product Details
ISBN-13: | 9781032209470 |
---|---|
Publisher: | CRC Press |
Publication date: | 05/27/2024 |
Series: | Chapman & Hall/CRC Statistics in the Social and Behavioral Sciences |
Pages: | 305 |
Product dimensions: | 6.12(w) x 9.19(h) x (d) |
About the Author
From the B&N Reads Blog