Bayesian inference provides a simple and unified approach to data analysis, allowing experimenters to assign probabilities to competing hypotheses of interest, on the basis of the current state of knowledge. By incorporating relevant prior information, it can sometimes improve model parameter estimates by many orders of magnitude. This book provides a clear exposition of the underlying concepts with many worked examples and problem sets. It also discusses implementation, including an introduction to Markov chain Monte-Carlo integration and linear and nonlinear model fitting. Particularly extensive coverage of spectral analysis (detecting and measuring periodic signals) includes a self-contained introduction to Fourier and discrete Fourier methods. There is a chapter devoted to Bayesian inference with Poisson sampling, and three chapters on frequentist methods help to bridge the gap between the frequentist and Bayesian approaches. Supporting Mathematica® notebooks with solutions to selected problems, additional worked examples, and a Mathematica tutorial are available at www.cambridge.org/9780521150125.
"1137482941"
Bayesian Logical Data Analysis for the Physical Sciences: A Comparative Approach with Mathematica® Support
Bayesian inference provides a simple and unified approach to data analysis, allowing experimenters to assign probabilities to competing hypotheses of interest, on the basis of the current state of knowledge. By incorporating relevant prior information, it can sometimes improve model parameter estimates by many orders of magnitude. This book provides a clear exposition of the underlying concepts with many worked examples and problem sets. It also discusses implementation, including an introduction to Markov chain Monte-Carlo integration and linear and nonlinear model fitting. Particularly extensive coverage of spectral analysis (detecting and measuring periodic signals) includes a self-contained introduction to Fourier and discrete Fourier methods. There is a chapter devoted to Bayesian inference with Poisson sampling, and three chapters on frequentist methods help to bridge the gap between the frequentist and Bayesian approaches. Supporting Mathematica® notebooks with solutions to selected problems, additional worked examples, and a Mathematica tutorial are available at www.cambridge.org/9780521150125.
62.49
In Stock
5
1
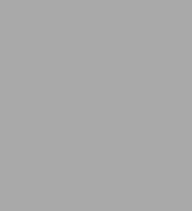
Bayesian Logical Data Analysis for the Physical Sciences: A Comparative Approach with Mathematica® Support
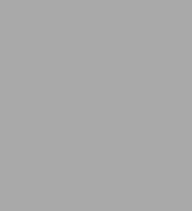
Bayesian Logical Data Analysis for the Physical Sciences: A Comparative Approach with Mathematica® Support
eBook
$62.49
$82.99
Save 25%
Current price is $62.49, Original price is $82.99. You Save 25%.
Related collections and offers
62.49
In Stock
Product Details
ISBN-13: | 9781107386006 |
---|---|
Publisher: | Cambridge University Press |
Publication date: | 04/14/2005 |
Sold by: | Barnes & Noble |
Format: | eBook |
File size: | 17 MB |
Note: | This product may take a few minutes to download. |
About the Author
From the B&N Reads Blog