DNA Methylation Microarrays: Experimental Design and Statistical Analysis / Edition 1 available in Paperback, eBook
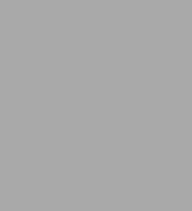
DNA Methylation Microarrays: Experimental Design and Statistical Analysis / Edition 1
- ISBN-10:
- 0367387409
- ISBN-13:
- 9780367387402
- Pub. Date:
- 10/21/2019
- Publisher:
- Taylor & Francis
- ISBN-10:
- 0367387409
- ISBN-13:
- 9780367387402
- Pub. Date:
- 10/21/2019
- Publisher:
- Taylor & Francis
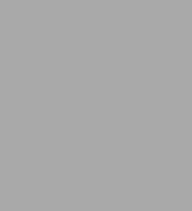
DNA Methylation Microarrays: Experimental Design and Statistical Analysis / Edition 1
Buy New
$84.99-
SHIP THIS ITEMIn stock. Ships in 3-7 days. Typically arrives in 3 weeks.PICK UP IN STORE
Your local store may have stock of this item.
Available within 2 business hours
Overview
After introducing basic statistics, the book describes wet-bench technologies that produce the data for analysis and explains how to preprocess the data to remove systematic artifacts resulting from measurement imperfections. It then explores differential methylation and genomic tiling arrays. Focusing on exploratory data analysis, the next several chapters show how cluster and network analyses can link the functions and roles of unannotated DNA elements with known ones. The book concludes by surveying the open source software (R and Bioconductor), public databases, and other online resources available for microarray research.
Requiring only limited knowledge of statistics and programming, this book helps readers gain a solid understanding of the methodological foundations of DNA microarray analysis.
Product Details
ISBN-13: | 9780367387402 |
---|---|
Publisher: | Taylor & Francis |
Publication date: | 10/21/2019 |
Pages: | 256 |
Product dimensions: | 6.12(w) x 9.19(h) x (d) |
About the Author
Table of Contents
1 Applied Statistics 1
1.1 Descriptive statistics 1
1.1.1 Frequency distribution 2
1.1.2 Central tendency and variability 2
1.1.3 Correlation 4
1.2 Inferential statistics 6
1.2.1 Probability distribution 6
1.2.2 Central limit theorem and normal distribution 7
1.2.3 Statistical hypothesis testing 7
1.2.4 Two-sample t-test 9
1.2.5 Nonparametric test 9
1.2.6 One-factor ANOVA and F-test 10
1.2.7 Simple linear regression 11
1.2.8 Chi-square test of contingency 13
1.2.9 Statistical power analysis 14
2 DNA Methylation Microarrays and Quality Control 17
2.1 DNA methylation microarrays 18
2.2 Workflow of methylome experiment 21
2.2.1 Restriction enzyme-based enrichment 21
2.2.2 Immunoprecipitation-based enrichment 21
2.3 Image analysis 23
2.4 Visualization of raw data 26
2.5 Reproducibility 26
2.5.1 Positive and negative controls by exogenous sequences 32
2.5.2 Intensity fold-change and p-value 32
2.5.3 DNA unmethylation profiling 33
2.5.4 Correlation of intensities between tiling arrays 33
3 Experimental Design 35
3.1 Goals of experiment 36
3.1.1 Class comparison and class prediction 36
3.1.2 Class discovery 36
3.2 Reference design 37
3.2.1 Dye swaps 39
3.3 Balanced block design 39
3.4 Loop design 41
3.5 Factorial design 42
3.6 Time course experimental design 47
3.7 How many samples/arrays are needed? 49
3.7.1 Biological versus technical replicates 49
3.7.2 Statistical power analysis 49
3.7.3 Pooling biological samples 55
3.8 Appendix 56
4 Data Normalization 59
4.1 Measure of methylation 59
4.2 The need for normalization 61
4.3 Strategy for normalization 62
4.4 Two-color CpG island microarray normalization 63
4.4.1 Global dependence of log methylation ratios 64
4.4.2 Dependence of log ratios on intensity 65
4.4.3 Dependence of log ratios on print-tips 67
4.4.4 Normalized Cy3- and Cy5-intensities 70
4.4.5 Between-array normalization 71
4.5 Oligonucleotide arrays normalization 72
4.5.1 Background correction: PM - MM? 72
4.5.2 Quantile normalization 73
4.5.3 Probeset summarization 75
4.6 Normalization using control sequences 76
4.7 Appendix 79
5 Significant Differential Methylation 81
5.1 Fold change 81
5.2 Linear model for log-ratios or log-intensities 84
5.2.1 Microarrays reference design or oligonucleotide chips 84
5.2.2 Sequence-specific dye effect in two-color microarrays 87
5.3 t-test for contrasts 88
5.4 F-test for joint contrasts 89
5.5 P-value adjustment for multiple testing 92
5.5.1 Bonferroni correction 92
5.5.2 False discovery rate 92
5.6 Modified t- and F-test 94
5.7 Significant variation within and between groups 95
5.7.1 Within-group variation 95
5.7.2 Between-group variation 96
5.8 Significant correlation with a co-variate 97
5.9 Permutation test for bisulfite sequence data 100
5.9.1 Euclidean distance 101
5.9.2 Entropy 102
5.10 Missing data values 103
5.11 Appendix 104
5.11.1 Factorial design 104
5.11.2 Time-course experiments 105
5.11.3 Balanced block design 106
5.11.4 Loop design 107
6 High-Density Genomic Tiling Arrays 109
6.1 Normalization 110
6.1.1 Intra- and interarray normalization 110
6.1.2 Sequence-based probe effects 110
6.2 Wilcoxon test in a sliding window 112
6.2.1 Probe score or scan statistic 116
6.2.2 False positive rate 116
6.3 Boundaries of methylation regions 118
6.4 Multiscale analysis by wavelets 119
6.5 Unsupervised segmentation by hidden Markov model 121
6.6 Principal component analysis and biplot 125
7 Cluster Analysis 129
7.1 Measure of dissimilarity 129
7.2 Dimensionality reduction 130
7.3 Hierarchical clustering 133
7.3.1 Bottom-up approach 133
7.3.2 Top-down approach 136
7.4 K-means clustering 139
7.5 Model-based clustering 141
7.6 Quality of clustering 142
7.7 Statistically significance of clusters 144
7.8 Reproducibility of clusters 146
7.9 Repeated measurements 146
8 Statistical Classification 149
8.1 Feature selection 149
8.2 Discriminant function 152
8.2.1 Linear discriminant analysis 153
8.2.2 Diagonal linear discriminant analysis 154
8.3 K-nearest neighbor 154
8.4 Performance assessment 155
8.4.1 Leave-one-out cross validation 156
8.4.2 Receiver operating characteristic analysis 159
9 Interdependency Network of DNA Methylation 163
9.1 Graphs and networks 164
9.2 Partial correlation 164
9.3 Dependence networks from DNA methylation microarrays 165
9.4 Network analysis 168
9.4.1 Distribution of connectivities 169
9.4.2 Active epigenetically regulated loci 169
9.4.3 Correlation of connectivities 170
9.4.4 Modularity 171
10 Time Series Experiment 179
10.1 Regulatory networks from microarray data 181
10.2 Dynamic model of regulation 182
10.3 A penalized likelihood score for parsimonious model 182
10.4 Optimization by genetic algorithms 184
11 Online Annotations 187
11.1 Gene centric resources 187
11.1.1 GenBank: A nucleotide sequence database 187
11.1.2 UniGene: An organized view of transcriptomes 188
11.1.3 RefSeq: Reviews of sequences and annotations 188
11.1.4 PubMed: A bibliographic database of biomedical journals 189
11.1.5 dbSNP: Database for nucleotide sequence variation 190
11.1.6 OMIM: A directory of human genes and genetic disorders 190
11.1.7 Entrez Gene: A Web portal of genes 190
11.2 PubMeth: A cancer methylation database 192
11.3 Gene Ontology 192
11.4 Kyoto Encyclopedia of Genes and Genomes 195
11.5 UniProt/Swiss-P rot protein knowledgebase 196
11.6 The International HapMap Project 198
11.7 UCSC human genome browser 198
12 Public Microarray Data Repositories 205
12.1 Epigenetics Society 205
12.2 Microarray Gene Expression Data Society 206
12.3 Minimum Information about a Microarray Experiment 206
12.4 Public repositories for high-throughput arrays 208
12.4.1 Gene Expression Omnibus at NCBI 208
12.4.2 ArrayExpress at EBI 208
12.4.3 Center for Information Biology Gene Expression database at DDBJ 210
13 Open Source Software for Microarray Data Analysis 211
13.1 R: A language and environment for statistical computing and graphics 212
13.2 Bioconductor 212
13.2.1 Marray package 215
13.2.2 Affy package 215
13.2.3 Limma package 215
13.2.4 Stats package 215
13.2.5 TilingArray package 217
13.2.6 Ringo package 217
13.2.7 Cluster package 217
13.2.8 Class package 217
13.2.9 GeneNet package 217
13.2.10 Inetwork package 217
13.2.11 GOstats package 218
13.2.12 Annotate package 218
References 219
Index 225
What People are Saying About This
I found the book to be very informative and a timely introduction to the issues related to designing and analyzing array-based methylation experiments. … it provides a solid grounding and serves as a good reference book for any statistician venturing into this field.
—Sarah Bujac, Pharmaceutical Statistics, 2011, 10
…a useful presentation of four detailed, well-written parts concerning techniques in the analysis of high throughput epigenomic data … a consistent and self-contained overview on important fundamental and modern procedures used by researchers in biology, bioinformatics, experimental designs …The book is of great interest to research workers who use the above-mentioned procedures in experimental design and deep analysis of epigenomic data with sound statistics.
—Cryssoula Ganatsiou, Zentralblatt MATH 1172
…This book is a helpful guide for researchers and students with an interest in performing genomic studies using high-throughput microarrays. … A wide range of useful data analysis tools are covered … Other strengths throughout the book include the discussion of experimental design, the mention of software for certain analyses, and the inclusion of more advanced methods such as wavelets and genetic algorithms. … Overall, this book gives a nice summary of methods used for the analysis of hybridization-based microarray data. …
—Biometrics, March 2009