Fast data ingestion, serving, and analytics in the Hadoop ecosystem have forced developers and architects to choose solutions using the least common denominator—either fast analytics at the cost of slow data ingestion or fast data ingestion at the cost of slow analytics. There is an answer to this problem. With the Apache Kudu column-oriented data store, you can easily perform fast analytics on fast data. This practical guide shows you how.
Begun as an internal project at Cloudera, Kudu is an open source solution compatible with many data processing frameworks in the Hadoop environment. In this book, current and former solutions professionals from Cloudera provide use cases, examples, best practices, and sample code to help you get up to speed with Kudu.
- Explore Kudu’s high-level design, including how it spreads data across servers
- Fully administer a Kudu cluster, enable security, and add or remove nodes
- Learn Kudu’s client-side APIs, including how to integrate Apache Impala, Spark, and other frameworks for data manipulation
- Examine Kudu’s schema design, including basic concepts and primitives necessary to make your project successful
- Explore case studies for using Kudu for real-time IoT analytics, predictive modeling, and in combination with another storage engine
Fast data ingestion, serving, and analytics in the Hadoop ecosystem have forced developers and architects to choose solutions using the least common denominator—either fast analytics at the cost of slow data ingestion or fast data ingestion at the cost of slow analytics. There is an answer to this problem. With the Apache Kudu column-oriented data store, you can easily perform fast analytics on fast data. This practical guide shows you how.
Begun as an internal project at Cloudera, Kudu is an open source solution compatible with many data processing frameworks in the Hadoop environment. In this book, current and former solutions professionals from Cloudera provide use cases, examples, best practices, and sample code to help you get up to speed with Kudu.
- Explore Kudu’s high-level design, including how it spreads data across servers
- Fully administer a Kudu cluster, enable security, and add or remove nodes
- Learn Kudu’s client-side APIs, including how to integrate Apache Impala, Spark, and other frameworks for data manipulation
- Examine Kudu’s schema design, including basic concepts and primitives necessary to make your project successful
- Explore case studies for using Kudu for real-time IoT analytics, predictive modeling, and in combination with another storage engine
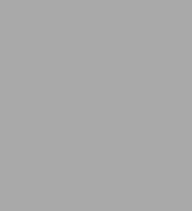
Getting Started with Kudu: Perform Fast Analytics on Fast Data
156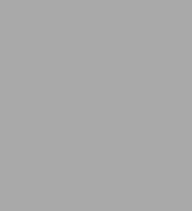
Getting Started with Kudu: Perform Fast Analytics on Fast Data
156eBook
Related collections and offers
Product Details
ISBN-13: | 9781491980200 |
---|---|
Publisher: | O'Reilly Media, Incorporated |
Publication date: | 07/09/2018 |
Sold by: | Barnes & Noble |
Format: | eBook |
Pages: | 156 |
File size: | 5 MB |