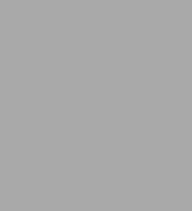
Inventing Money: The Story of Long-Term Capital Management and the Legends Behind It
288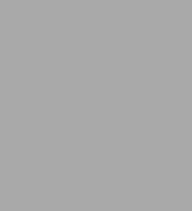
Inventing Money: The Story of Long-Term Capital Management and the Legends Behind It
288Paperback(Revised ed.)
-
SHIP THIS ITEMIn stock. Ships in 1-2 days.PICK UP IN STORE
Your local store may have stock of this item.
Available within 2 business hours
Related collections and offers
Overview
Product Details
ISBN-13: | 9780471498117 |
---|---|
Publisher: | Wiley |
Publication date: | 12/22/2000 |
Edition description: | Revised ed. |
Pages: | 288 |
Product dimensions: | 6.06(w) x 9.00(h) x 0.62(d) |
About the Author
In 1990, Dunbar decided to leave academia. He spent the next few years working in feature films and television, in a wide range of capacities. In 1996, after launching the television production company Flicker Films, a chance encounter with some old Harvard friends set him on a new path of finance and science writing, focusing on the derivatives industry. In 1998, he joined Risk magazine as technical editor.
Read an Excerpt
Chapter One
The Theory of Speculation
'I can calculate the motions of heavenly bodies,
but not the madness of people'
Isaac Newton, after losing £20,000 in the stock market.
A man was crossing a bridge over the Charles River from Boston to the Massachusetts Institute of Technology (MIT) in Cambridge. It was October 1968 and there was plenty going on to distract Fischer Black. Richard Nixon was on his way to winning the presidential election, and the country was bitterly divided over the Vietnam War, where almost a million US troops were in action. Earlier that year, Martin Luther King had been assassinated, a bitter blow to those living in Boston's black ghetto of Roxbury. All over the world that year, riot shields confronted student banners.
But Black had other things on his mind. A tall, quiet man with slicked-back hair and chunky spectacles, he wore a dark suit, in contrast to the long hair and afghan coats of the students leaving their classes. Black didn't notice the students; he was deep in thought as he entered the MIT economics faculty. He was there at the invitation of a young Canadian academic, Myron Scholes, who had recently joined the faculty from Chicago.
Aged 30, Black was earning a living at Boston consulting firm Arthur D. Little, trying to advise mutual funds on their stock market investments. But Black's heart wasn't in his work. Instead, he was seeking Scholes's help in pricing an obscure type of financial contract called an option. Black didn't yet know about the brainy youngstudent in the economics department, Robert C. Merton, who was interested in the same problem.
A talkative 27-year-old, Scholes showed his visitor into his office, and brought him a cup of coffee. He couldn't possibly know that the work he and Black were about to embark on would one day result in him and Merton shaking hands with the King of Sweden and accepting a Nobel Prize. He would have been insulted by the suggestion that shortly afterwards, their activities at a hedge fund called LTCM (Long-Term Capital Management) would paralyse the global financial system.
The two papers on the subject that the trio eventually published nearly five years later don't look too inspiring at first sight. Black and Scholes's opus was called 'On the pricing of options and other corporate liabilities' while Merton's paper rejoiced in the title of 'The rational theory of option pricing'. Turn a few pages, and mathematical equations start dancing before one's eyes.
Yet, although the ideas of Black, Scholes and Merton didn't have much immediate impact in 1973, they eventually would change the world, along with the lives of their authors. Options would become vitally important to finance, but there was more to it than that. Black, Scholes and Merton had invented what came to be known as financial engineering.
Just as the engineering of digital bits would eventually lead to the Internet, the mathematically driven engineering of stocks, bonds and other securities would create the modern trillion-dollar financial system. Unlike the Internet, or the space shuttle, or any other engineering achievement, this one is largely invisible. Only when a disaster strikes, as would happen to Merton and Scholes and their colleagues at LTCM in 1998, do people notice.
Playing in the movie theatres in Autumn 1968 was the film by Stanley Kubrick, 2001: A Space Odyssey. The film opens with a stunning sequence where a prehistoric hominid hurls a bone spinning into the air, which becomes transformed into an orbiting space station. The story of finance is no different. When combined with mathematics and technology, the ancient urge to make money is amplified into a force of awesome power.
Addressing Congress after the LTCM débâcle, what did Federal Reserve Board chairman Alan Greenspan mean when he spoke of 'mathematical models of human behaviour'? We can be sure of one thing. Markets arrived long before mathematics did. In fact, they go back to the distant origins of human behaviour, millions of years ago on the sweltering African savannah. Before we discover what Black, Scholes and Merton actually did, it's worth taking a brief excursion back in time to find out more.
The origin of trading
In chimpanzee communities, individuals exchange gifts (such as fruit or sexual favours) within a group to cement alliances, and punish those who attempt to cheat on such mutually beneficial relationships. Anthropologists believe that early humans started trading in much the same way. The word they use to describe this behaviour is 'reciprocity' and our personal relationships work on this basis.
Helped by the gift of language, humans took trading far beyond the level of immediate family groups. Like chimps, humans have a xenophobic streak. Nevertheless, realising the advantage of specialising in the production of certain commodities, tribes that had once interacted purely to kill and enslave each other began exchanging goods through barter.
The ability to specialise gradually increased everyone's wealth, encouraged innovation, and the life of a hunter-gatherer slowly gave way to civilisation which brought advantages and disadvantages. Tribes that weren't interested in trading were either annihilated or forced to retreat to remote parts of the world where civilisations weren't viable. As agricultural economies replaced nomadic ones, one-to-one trading gave way to markets.
In a barter economy, cementing friendship is still a necessary part of exchanging goods. After all, if you needed to sell something, first you must look around for a partner who can offer you something worth while in return. Every barter trade is a unique, human event. It depends intimately on time and place, as well as the personalities and histories of those who took part. To make a living purely through barter, you need a network of friends and acquaintances whose needs you understand.
All this changed when people started using money, which was first developed in the agricultural states of the ancient Middle East. Money establishes the meaning of a 'fair' price. If a complex, tortuous negotiation could be summarised by a simple cash value, then it could be compared with other deals at different times and places. The value of this information was quickly realised by governments, who could use it to collect taxes, and pay their armies and bureaucrats. For this reason, the use of money was associated with an increase in state power.
That wasn't completely a bad thing. With state power came the rule of law. In the old barter economy, the only way to punish fraud or cheating was to seek out the cheat in person, and claim damages. If the cheat had returned to the safety of his village, this might be impossible. Once legal codes came into existence, plaintiffs could appeal to state authority to enforce agreements.
In time, confidence in the law increased so much that legal agreements such as bills of receipt or share certificates became readily exchangeable for cash. We would now call these bits of paper a form of risk management. In particular, shares were an important invention. Up to the seventeenth century, if you wanted to invest in a company, you had to join its owners in a partnership. If the company went bankrupt, you would be liable for its debts. But shares had something called 'limited liability', which protected investors from creditors. This very primitive form of financial engineering encouraged people to invest, and allowed economies to grow.
In a sense, the development of money and financial contracts was a democratic event. The knowledge of a fair price was hard to keep secret. Even in countries where prices are imposed by governments, such as communist states, 'real' prices soon emerge in the black market. Events with widespread economic impact, such as crop failures, are rapidly communicated to an entire population in the form of price changes, and can also lead quickly to political change.
The effect of money and law was to separate trading from friendship. The rule of law means that one no longer needs to build up a relationship of trust in order to trade. The priority becomes no longer to make a deal with the best person, but to obtain the best price. And the more impersonal things are, the better, in the sense that the best-price transaction is economically beneficial to everybody. Adam Smith was the first to comment on this:
It is not from the benevolence of the butcher, the brewer, or the baker, that we expect our dinner, but from their regard to their own interest ... This division of labour ... is not originally the effect of any human wisdom, which foresees and intends that general opulence to which it gives occasion. It is the necessary, though very slow and gradual, consequence of a certain propensity in human nature which has in view no such extensive utility; the propensity to truck, barter, and exchange one thing for another.
The theory of markets starts with Smith, the Scottish Enlightenment philosopher who first argued that greed was good. In The Wealth of Nations, published in the year of the American revolution, Smith paints a picture of ruddy-cheeked butchers, bakers and brewers who each put their own interests first, and yet create wealth collectively.
Back in 1776, this was a radical idea. Before the industrial revolution, markets were seen as a low-grade human activity, which often fell under the Devil's influence. Greed was evil it was a deadly sin and its appearance in economic affairs should be curbed by monarchs and religious institutions that answered to God. And where was the evidence that contracts like shares actually helped anybody?
In 1720, when Smith was only three-years old, this hostile view of free markets had been confirmed by the scandal of the South Sea Bubble. Founded by a Tory minister in 1711, the South Sea Company was intended to profit from trade with the Spanish colonies, but instead, used shareholders' money to pay off the national debt. To stoke public interest, the directors of the South Sea Company sold additional stock, which investors could use as security to borrow money to buy yet more stock, and even issued a press release to promote their efforts.
This pyramid investment scheme gathered momentum, and by early 1720 it had spawned dozens of imitators. On 1 January 1720, South Sea shares sold for £128. By 24 June, they reached £1050. However, the market crashed in September, and by December, the share price was back at £128. Thousands of investors were ruined. Banks that had lent money against the value of the stock went bankrupt.
Even Isaac Newton, basking in the glory of having discovered gravitation, lost £20,000 in the scheme a fortune in today's money. In the scandal's wake, chastened legislators passed a law forbidding new issues of stock without royal authority. Then, 55 years on, Smith was saying that all that control should be thrown out, leaving an 'invisible hand' to create human happiness.
To argue his case, Smith listed examples in which high taxes a key economic tool of autocratic governments had led to popular unrest. The American revolution was to provide a textbook example of Smith's argument. Unsurprisingly, the newly created United States took Smith's philosophy to its heart. And the bottom line was that the desire to make money was a natural law that should be allowed to run its course.
Smith's invisible hand has the air of science about it. Aren't Newton's laws of gravity an invisible hand guiding the planets? Notwithstanding Newton's sheepish comment at the head of the chapter, isn't it possible to write down a law of self-interest, that maximises wealth? Unfortunately, even if Smith had had the vision, the mathematical apparatus required even to attempt this wasn't yet available. Economic laws, if they indeed exist, must be approximate ones, and need statistics.
Of dogs and molecules
Ultimately, mathematics would find its way into finance, but statistics had to come first. Therefore, the road from Adam Smith to Black, Scholes and Merton takes us on a detour away from the markets and into the laboratories and lecture halls of Victorian science who first exploited statistics. For the biologists and the physicists of the nineteenth century were modern in the sense that, where their predecessors thought about observations, they thought about data.
Up to the end of the eighteenth century, biology largely consisted of either dissection (of plants, humans and animals) and taxonomy, or classification. Observation meant going to the tropics, finding new species to be classified, and taking a few samples home for the collection. The Victorian naturalists changed all that. Obsessive cataloguers and note-takers, they made the bold step of seeing plants, animals and people as populations rather than individuals.
Consider 100 dogs, picked at random. Although they might range widely in size and appearance, an eighteenth-century naturalist would have focused on what these dogs had in common, in terms of internal anatomy, as well as their taxonomic relation to other species such as wolves.
A nineteenth-century biologist would have made lists: weights, heights, lifespans, size of litter and so on. The next step would be to take this list, say for heights, and take the average, namely, add up all the heights measured and divide by the total number of dogs (in this case, 100). However, this average or mean height might not correspond to any particular dog in the sample. There is no such thing as an 'average dog', only a number representing a given collection of dogs.
But there is a further step which is crucial to this book. Although the average is useful, what about the variation in size and appearance? Our Victorian naturalist can do more with his data. He takes the average, subtracts it from each height on his list and squares the results. He then calculates the average of this new list, and finally takes the square root to give a number called the standard deviation.
The standard deviation tells us how spread out the data are. For example, if our dog sample consisted of 100 Labradors of the same age, the standard deviation of the height would be small. If it contained every breed from Chihuahuas to Great Danes, the standard deviation would be large. Like the mean, the standard deviation is clearly not intrinsic to any particular dog, but is a property of the group.
Such observations of variation in animals were seized upon by Charles Darwin in the Origin of Species as important evidence for evolution. By arguing that the mean and standard deviation were intrinsic qualities of every species, Darwin made two radical suggestions. Firstly, that these qualities were passed from parent to offspring by inheritance (the science of genetics would later explain how). Secondly, that a variation in some quality (such as beak size in Galapagos finches) could, over long periods of time, and if geographical conditions encouraged it, lead to completely new species through natural selection.
The Victorian hunger for data led to another, more subtle discovery. Recall our imaginary dog study. Suppose the naturalist constructs a chart with height plotted on the horizontal axis, and the number of times a particular range of heights was observed plotted on the vertical axis. Such charts, called distributions, tend to have a peak around the mean value.
As more and more data was used to produce distributions, a peculiar property was noticed. Whatever the actual data involved whether it measured animals, humans or plants the distribution took on a characteristic humped shape. In recognition of its importance, the curve has a special name: the Normal distribution. Called the Bell curve in the US because of its shape, the curve is also known as the Gaussian distribution. This last name derives from the German mathematician C.F. Gauss who observed the same distribution of errors in cartographers' distance measurements.
The Normal distribution is symmetrical about the mean, and the standard deviation measures the width of the central hump. Beyond this hump, on either side, the curve then flattens out and soon becomes close to zero.
But the most striking feature of the Normal distribution is its meaning; it is nature's evidence that something is completely random. We can see why this is so by trying to construct a Normal distribution from scratch. This construction starts out with the quintessential random event: a coin toss. Toss a coin a hundred times and note down the number of heads. This gives you one data point. Do it again and again and again. Eventually, if you have not run out of patience, you plot the distribution of heads, and it will look very much like the Normal distribution.
For mathematicians, the Normal distribution is very pure, and hence, a prized object. Aside from a vertical distance scale, its shape can be captured by only two numbers the mean and standard deviation. If a real-life data distribution fails to reflect the Normal distribution as the amount of data increase, statisticians are trained to smell a rat, and look for something non-random in the data.
The Normal distribution has another, very reassuring property. Recall our naturalist who measures the heights of 100 dogs. There may be some specific reason why the distribution of heights differs from Normal. For example, in the area of study, large dogs might run away before being measured. In other areas there may be different problems. But if the naturalist repeats the experiment at many locations, the distribution of the means will eventually look like the Normal distribution. Statisticians call this the 'Central Limit Theorem', and it seems to be saying that pure randomness always wins out in the end. The Normal distribution plays an important role in the LTCM story, as we shall see.
While randomness would play an important, but subtle role underpinning Darwin's theory of evolution, it became a central driving force in nineteenth-century physics. The idea that matter was made of atoms and molecules had been around since ancient Greece but for the first time, the idea could be tested. The goal was to explain the macroscopic properties of matter, such as heat flow and gas pressure, in terms of atomic behaviour.
With the tools of statistics at their disposal, theorists like James Clerk Maxwell in the 1860s were able to make intellectual leaps. Because no-one had ever seen a molecule (nor would they for another 100 years) Maxwell had to come up with a model. In the eighteenth century, the physicist Daniel Bernoulli had proposed that a gas inside a container consisted of countless tiny molecules, constantly bouncing off the walls and each other. Maxwell realised that this motion had to be random.
Maxwell started out by assuming that the gas was in equilibrium which means that on average, it wasn't gaining or losing energy to its surroundings. If the gas were in equilibrium, said Maxwell, the molecules' speeds in a given direction would have the Normal distribution, because of their randomness.
Clearly, the mean would have to be zero, because the molecules couldn't be moving anywhere on average in a closed container. The standard deviation was proportional to the temperature, and also the pressure exerted by the gas on the container walls. Without observing a single molecule, Maxwell had used statistics to explain the properties of gases.
Despite the elegance and power of Maxwell's 'kinetic' theory, many felt tricked by his statistical sleight of hand. These sceptics claimed that molecules were just imaginary constructs used by Maxwell, and his successor Boltzmann, to give the answers they wanted. The supporters of Maxwell's theory or the atomists, as they were known, needed to find some direct evidence. This came, in part, from a botanist called Robert Brown.
In 1828, Brown published a paper entitled 'A Brief Account of Microscopical Observations Made in the Months of June, July and August 1827, on the Particles Contained in the Pollen of Plants'. Brown reported that when he peered down a microscope at pollen grains in water, he observed the grains in incessant, random motion. At first, Brown thought the grains might be bacteria, but soon proved that this was not the case. Brown went on to note the same jiggling of dust particles from a wide range of sources, ranging from London soot to a pulverised fragment of the Sphinx.
By the 1860s, atomists began suggesting that Brownian motion, as it came to be known, was the result of the grains being randomly buffeted by invisible water molecules. The jiggling increased with temperature the standard deviation of the molecules' speed, remember supporting this theory.
However, anti-atomists poured scorn on the idea, correctly pointing out that individual molecules were far too small to move a pollen grain on their own. The effect had to be statistical in nature. But how? What held the atomists back was the lack of a mathematical framework to express their idea.
The invisible man
In the last years of the nineteenth century, Frenchman Henri Poincaré was renowned as one of the world's leading mathematicians. Taking a deep interest in physics, Poincaré did groundbreaking work in optics, classical mechanics and relativity. But when he gave the opening address to a packed hall in Paris at the International Congress of Physics in 1900, he talked about Brownian motion:
Brown first thought that Brownian motion was a vital phenomenon, but soon he saw that inanimate bodies dance with no less ardour than the others; then he turned the matter over to the physicists ... We see under our eyes now motion transformed into heat by friction, now heat changed inversely into motion.
Across town, in the Sorbonne university, Poincaré's student, Louis Bachelier, was completing his doctoral dissertation. When he had suggested that Brownian motion would make a good thesis topic for Bachelier, Poincaré was handing him one of the plum problems of the day, and must have had high hopes for his young student. Bachelier in turn would have counted himself lucky to be part of the inner circle of Paris's star professor.
Here's what Bachelier did. The problem is how to reconcile two levels of randomness: the motion of a dust particle, which we can see, and the motion of trillions of molecules, which we can't see. Clearly, the scales of size are very different because the particle is so much bigger than the molecules, a lot of molecules have to gang up together in order to move the particle. It is like the battle between Gulliver and the Lilliputians in Swift's Gulliver's Travels.
The time-scales of particles and molecules are different as well. The Lilliputians do a lot of running around and arguing, but on average they manage to co-operate enough to move Gulliver. Likewise, molecules do a lot of scurrying around in between every move made by the dust particle. This fact allowed Bachelier to make his crucial step.
Imagine Robert Brown, peering down his microscope, making notes. As the particle moves this way and that, Brown notes whether it moved left or right, and the distance it covered. He then plots this information on a chart, showing distance on the horizontal axis, and the number of times that particular distance was observed on the vertical axis. As with the naturalist observing dogs, we have another example of a distribution.
At first sight, the shape of this distribution should depend on what the molecules are doing at a given time it should be different every time we do the experiment. But the difference in time-scales comes to the rescue. A move by the dust particle that Brown is capable of seeing in his microscope must be the sum of millions of tiny pushes given to it by molecules. These tiny pushes themselves each form a distribution, but when we add them together, the Central Limit Theorem means the visible movement of the dust particle always follows a Normal distribution.
In Swiftian terms, in the time it takes for Gulliver to move from one direction to the other, the Lilliputians have forgotten what they were doing, and act completely randomly. The only remnant of the molecules' behaviour, like a Cheshire Cat's grin, is in the standard deviation which describes how spread out the dust particles' distribution is.
You might think that over time, a particle would stay in the spot where it started out. After all, the Normal distribution tells us that a given movement to the left is as likely as the same move to the right in other words, it is symmetrical. But once the particle makes a large, rare move in one direction, its next move is likely to be small, because the Normal distribution is peaked around zero. This means that the particle slowly drifts away from its starting point, making what is known as a random walk.
Because of the drift, if you start out by clustering all the particles together, they will spread out over time. We see this happening every day: cigarette smoke spreads out and disappears in a room, while a drop of ink spreads out in a glass of water. These are examples of what are called diffusion processes.
For a particle moving at constant speed, in the absence of molecules, the distance travelled is proportional to the time taken. When the molecules force the particle to take a random walk, it turns out that the average distance the particle travels is proportional to the square root of the time it takes. If you can measure both these quantities, then you can unlock the properties of the molecules captured in the standard deviation. The paper that first showed how to do all this helped make its author famous, and clinched victory for the atomists. In a testament to its popularity, the paper is still in the top ten list of all physics citations from before 1912.
However, that paper was not written by Louis Bachelier. It was written by Albert Einstein in 1905. And while Bachelier did indeed discover the mathematics of Brownian motion in his 1900 thesis, the motion of dust particles was not mentioned once. The paper was about the Paris stock market.
Reading through the 70-page thesis, entitled 'The Theory of Speculation', Poincaré must have suspected a practical joke. He didn't make the connection with Brownian motion, and from contemporary accounts, Bachelier seems to have been strangely unassertive. It doesn't pay to be too original in the presence of a star professor, and by doing so, Bachelier killed his career stone dead. For the rest of his life, disowned by his mentor, Bachelier struggled to find teaching work.
What had Bachelier done? 120 years after Adam Smith, and 180 years after Isaac Newton gave up on trying to understand the 'madness of people', Bachelier struck out into the unknown. Instead of a dust particle, Bachelier thought of a stock price as being buffeted up and down, not by molecules but by the invisible moods of thousands of investors and traders. Here was Alan Greenspan's 'model of human behaviour', written down for the first time.
Bachelier's reasoning is no different from that used to model the jiggling of dust particles. A large company (such as a component of the Dow Jones Industrial Average) has millions of shares in circulation. With thousands of speculators buying and selling the shares in the course of a day, each trade gives a tiny 'push' to the share price.
When we add the 'pushes' together, by using that statistician's party trick, the Central Limit Theorem, the changes in price follow a Normal distribution. Like Lilliputians, the speculators' constant to-ing and fro-ing means that by the time they have made a difference, they can't remember why. All one needs to know about the speculators' behaviour gets wrapped up in the standard deviation.
We'll come back to Bachelier's vision many times in this book, so it is worth putting it in context. What started hundreds of thousands of years ago as individuals exchanging goods on the African savannah had turned into an emerging global economy by 1900, with a dozen national stock and commodity markets in operation around the world.
The fear and greed in the market had disturbed Isaac Newton in 1720, and Adam Smith was forced to defend the system as being good for humanity. Since then, Karl Marx had argued that markets served the needs only of capitalists exploiting the workers. Now Bachelier was telling the world that the human drama in the market could be reduced to a science related to the mathematics of tossing coins. Discarded as a curiosity, his work would remain forgotten for over fifty years.
What Bachelier didn't have was an ideology. Capitalism had plenty of supporters in 1900, but finance and the markets were still seen as an appendage. Over that ensuing 50-year period, Russia and China would violently reject the system. Even in the United States, Adam Smith's spiritual heartland, markets were blamed for causing the Great Depression in the 1930s.
The change in climate would come during the early years of the Cold War. An intellectual revolution set in motion at the University of Chicago would bring mathematics into the markets, and eventually turn finance into science. Set loose in the US economy, this new science would flourish, and eventually help win the Cold War. We will join this revolution through the eyes of a Canadian youth Myron Scholes.
The gurus of finance
Timmins, Ontario is about as close to the edge of the world as you can get. Located near Hudson Bay in the low-lying north-east of the Canadian province, the town owes its existence to the substantial gold deposits nearby. The average winter temperature of minus 15°C quoted by the local chamber of commerce leaves out a vicious wind-chill factor. Only a few hundred miles further north, the surrounding pine forests give way to tundra that stretches half-way to the North Pole.
Myron Scholes was born in Timmins on 1 July 1941. His father, Jesse, was a dentist, and had moved there several years earlier to take advantage of the local economy during the Depression. There was certainly no shortage of gold for fillings in Timmins.
Scholes's mother, Anne, was a strong character. With the help of her uncle, she opened a department store in Timmins, and expanded the business into a chain of similar outlets. When her uncle died suddenly of a heart attack in 1950, his surviving family wrested control of the business away from Anne, with the help of crafty local lawyers. As Scholes later laconically put it, this was his 'first exposure to agency and contracting problems'.
Furious at the betrayal, Anne made the family move 500 miles south to the town of Hamilton where her brother ran a publishing and promotion business. Jesse Scholes had to abandon his dentistry practice and retrain as an orthodontist. Resigned to being a housewife, Anne now focused on her son.
Short-sighted and unco-ordinated, Scholes may have lacked ability in ice hockey or other Canadian sporting traditions. However, he was studious, had a head for figures and most importantly, got on with people. At high school he became treasurer of countless school clubs, making himself too useful to be bullied.
Meanwhile, Anne Scholes plotted out for him the business career in which she had failed herself. The plan was that Myron would join his uncle's publishing business after university. Feeling a close bond to his mother, Scholes dutifully worked for his uncle during school holidays, and even tried gambling, not to enjoy himself, but 'to understand probabilities and risks'. When her son reached fifteen, Anne opened a brokerage account for him, so he could learn about the stock market.
Then tragedy struck the family a second time. Diagnosed with terminal cancer, Mrs Scholes would never see the results of her investment. She died three days after Scholes's sixteenth birthday. The bereavement nearly floored Scholes. Shortly after the funeral, he developed scars on the corneas of both eyes, making him virtually blind.
For Scholes, reading a newspaper let alone a textbook was now a slow, painful experience. Years later, he managed to see the ailment in a positive light: 'I learned to think abstractly and conceptualise the solution to problems.' Leaving Hamilton was now out of the question, so Scholes enrolled at the local college, McMaster University, after which he would join the family business.
Living at home and commuting to class, Scholes worked hard. Jesse took over Anne's role in funding his brokerage account. Mapped out by his dead mother, Scholes's life now seemed on autopilot. However, half-blind as he was on the outside, Scholes was changing on the inside. 'Out of necessity, I became a good listener', he commented later.
Studying economics, Scholes listened especially carefully to a Professor McIver, who had got an economics PhD from the University of Chicago. According to McIver, Chicago was the place to be. Here were professors such as Milton Friedman and George Stigler, a new wave of free-marketeers who were revolutionising the subject. Here too, was a chance to escape from Canadian provincial life and live in a vast metropolis. Scholes was hooked, and when Chicago offered him a graduate school place in economics, he decided that his uncle's business could wait a little longer.
So what had been happening in Chicago? For the economists who arrived there in the 1940s and 1950s, their defining experience had been the trauma of the Great Depression. In particular, the Wall Street crash of October 1929 had shaken up everyone's ideas about investment. Until then, investing in stocks was like shopping. You would browse through the supermarket until you found a few select brands or stocks that you liked. Then you would stick with them for years, earning dividends while your investment grew in value.
1929 blew this cosy theory out of the water. Favourite brands of stock collapsed like dominoes along with the entire market. The companies that didn't go out of business completely, took years to regain their pre-1929 price and pay dividends again. Clearly stocks were risky. In an echo of the South Sea Bubble, laws were passed during the 1930s forbidding them as investments.
What happened next mirrored the changes in biology between the eighteenth and nineteenth centuries. Rather than trying to dissect individual companies, to see if they were 'good' or 'bad' investments, the new generation of self-styled financial economists started looking at pure data.
To see how radical this approach was, imagine investing in a modern company, Microsoft. The traditional approach would be to investigate Microsoft's products (for anyone using a personal computer, it's hard not to). Then you read its annual and quarterly earnings reports, to see how much money it is making, and how much it pays to shareholders. You might also read some interviews with Microsoft's CEO, Bill Gates, to see what his strategy was. At the end of all this, you make a decision whether or not to buy Microsoft shares.
The new generation would put all that carefully gathered information in the garbage can. Instead, they would sharpen their pencils, and focus on one thing: Microsoft's daily share price. They would gather a year's worth of prices and look at them using statistics. A single price perhaps from the end of the year no longer means anything. The new generation would define 'Microsoft' as a collection of share prices, measured one after the other, just as the nineteenth-century naturalist defined the species of dogs as a population of individuals.
With a share price measured in dollars, pounds or whatever the financial economists weren't quite ready to go to work. They needed a way to compare different stocks. For example, if the price of Microsoft rises by $5, that means something different from IBM rising by the same amount. If one of these companies pays out a dividend, that means something different again. To get round this problem, the early financial economists used the concept of returns the percentage increase in the value of an investment after a day, a month and so on. Dividends were included as part of the return.
Like the heights of dogs in our earlier example, daily returns can be averaged, and that says something meaningful. The fact that Microsoft had an annual return of 114 per cent in 1998, while for Netscape the figure was 149 per cent, clearly tells us that Netscape was a better investment. Perhaps it's enough to stop there, sell Microsoft and buy Netscape. Many investors do just that.
However, it is the standard deviation the Cheshire Cat grin of Bachelier's speculators that we have forgotten about. Known as volatility in finance, the standard deviation of price returns tells us how the returns are spread out around the average. And that tells us something about risk. For example, Netscape has a higher volatility than Microsoft, suggesting that it is a riskier investment.
It was in Chicago, in 1952, that a graduate student named Harry Markowitz first brought this idea into finance. A tetchy and unsocial man, Markowitz made few friends among the Chicago economics faculty, and was nearly denied his PhD by a hostile thesis committee. But Markowitz's idea would change finance.
In order to say something useful about investment, Markowitz considered a portfolio, or group, of stocks. It's straightforward to calculate the average return and volatility of each individual stock. But what about the whole portfolio? To deal with this Markowitz looked at a statistical measure, the correlation, of how much each stock moved in line with the others.
Correlation takes us into new territory. Rather than being a property of a single collection of data, it provides a link between two separate distributions. As we shall see later in the LTCM story, it is one of the most subtle and treacherous tools in statistics. But it worked a miracle for Markowitz.
To see how, imagine a portfolio with two risky stocks: an ice cream company and an umbrella company. Ice cream sells when the weather is sunny, and umbrellas sell when it is rainy. The first company does well when the other does badly, and vice-versa. In the language of statistics, we say that their returns have negative correlation.
Now think about the total value of the portfolio. Day by day, this value is the sum of the two stocks. The volatility of each stock tells us how much its return fluctuates around the mean. However, when ice cream moves up, umbrellas move down at least on average. Because of this, the portfolio value hardly fluctuates at all, or in other words, its volatility is low.
Low volatility means low risk. By not putting all our eggs in one basket, we have made a safer investment. As Markowitz would put it, the risk has been diversified away. Fifty years on, this idea seems like no more than common sense. Back in 1952, it seemed revolutionary.
In his thesis, Markowitz suggested that his correlation trick could be used to build portfolios. All one needed to do, he said, was maximise the total return, at the same time as minimising the volatility, or risk. Markowitz dubbed a collection of stocks having this property as an 'efficient portfolio'.
It was this insight that earned Markowitz a Nobel prize years later. Back in the 1950s, however, there was a problem: how to handle the data needed to come up with useful results. First of all, one might calculate the annual return and volatility for a single stock. In one year, there are 252 trading days, which means 252 individual numbers daily returns needed to obtain the result.
Now consider a portfolio consisting of the Dow Jones Industrial Average, which contains 30 stocks. We now calculate using our 252 daily returns, and repeat this 30 times. That means handling 7560 numbers. For 30 stocks, there are a total of 420 correlations we need to calculate. To do this, a total of 106,000 numbers must be obtained and added together.
Today, desktop computers can do this in less than a second. In the 1950s and 1960s, the primitive machines available would require hours or even days. This bottleneck had two consequences. Firstly, it made technology a force in finance, and second, it made the younger generation who knew how to get the most out of these early computers especially valuable.
In the summer of 1963, completing his first year at the University of Chicago, Myron Scholes made one of the smartest decisions of his life. Despite having no experience, he pressured the dean of the economics department into giving him a summer job programming the department's
(Continues...)
Table of Contents
Preface to the Paperback Edition.Acknowledgements.
Introduction.
The Theory of Speculation.
The Science of Fear and Greed.
Trading in Time.
The Garden of Forking Paths.
The Warning.
The Dream Team.
Out of Control.
The Song of a Martingale.
Aftermath.
Sources and Further Reading.
Index.