Learning Systems
A learning system can be defined as a system which can adapt its behaviour to become more effective at a particular task or set of tasks. It consists of an architecture with a set of variable parameters and an algorithm. Learning systems are useful in many fields, one of the major areas being in control and system identification. This work covers major aspects of learning systems: system architecture, choice of performance index and methods measuring error. Major learning algorithms are explained, including proofs of convergence. Artificial neural networks, which are an important class of learning systems and have been subject to rapidly increasing popularity, are discussed. Where appropriate, examples have been given to demonstrate the practical use of techniques developed in the text. System identification and control using multi-layer networks and CMAC (Cerebellar Model Articulation Controller) are also presented.
"1100828154"
Learning Systems
A learning system can be defined as a system which can adapt its behaviour to become more effective at a particular task or set of tasks. It consists of an architecture with a set of variable parameters and an algorithm. Learning systems are useful in many fields, one of the major areas being in control and system identification. This work covers major aspects of learning systems: system architecture, choice of performance index and methods measuring error. Major learning algorithms are explained, including proofs of convergence. Artificial neural networks, which are an important class of learning systems and have been subject to rapidly increasing popularity, are discussed. Where appropriate, examples have been given to demonstrate the practical use of techniques developed in the text. System identification and control using multi-layer networks and CMAC (Cerebellar Model Articulation Controller) are also presented.
54.99
In Stock
5
1
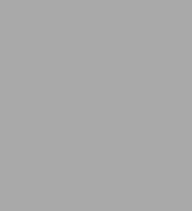
Learning Systems
119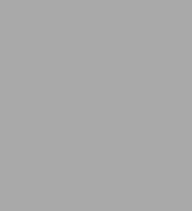
Learning Systems
119Paperback(1st Edition.)
$54.99
54.99
In Stock
Product Details
ISBN-13: | 9783540199960 |
---|---|
Publisher: | Springer London |
Publication date: | 11/22/1995 |
Edition description: | 1st Edition. |
Pages: | 119 |
Product dimensions: | 6.10(w) x 9.25(h) x 0.01(d) |
From the B&N Reads Blog