In the 1990s, a new type of learning algorithm was developed, based on results from statistical learning theory: the Support Vector Machine (SVM). This gave rise to a new class of theoretically elegant learning machines that use a central concept of SVMs—-kernels—for a number of learning tasks. Kernel machines provide a modular framework that can be adapted to different tasks and domains by the choice of the kernel function and the base algorithm. They are replacing neural networks in a variety of fields, including engineering, information retrieval, and bioinformatics.
Learning with Kernels provides an introduction to SVMs and related kernel methods. Although the book begins with the basics, it also includes the latest research. It provides all of the concepts necessary to enable a reader equipped with some basic mathematical knowledge to enter the world of machine learning using theoretically well-founded yet easy-to-use kernel algorithms and to understand and apply the powerful algorithms that have been developed over the last few years.
In the 1990s, a new type of learning algorithm was developed, based on results from statistical learning theory: the Support Vector Machine (SVM). This gave rise to a new class of theoretically elegant learning machines that use a central concept of SVMs—-kernels—for a number of learning tasks. Kernel machines provide a modular framework that can be adapted to different tasks and domains by the choice of the kernel function and the base algorithm. They are replacing neural networks in a variety of fields, including engineering, information retrieval, and bioinformatics.
Learning with Kernels provides an introduction to SVMs and related kernel methods. Although the book begins with the basics, it also includes the latest research. It provides all of the concepts necessary to enable a reader equipped with some basic mathematical knowledge to enter the world of machine learning using theoretically well-founded yet easy-to-use kernel algorithms and to understand and apply the powerful algorithms that have been developed over the last few years.
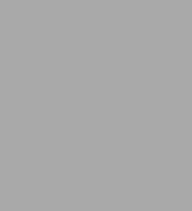
Learning with Kernels: Support Vector Machines, Regularization, Optimization, and Beyond
648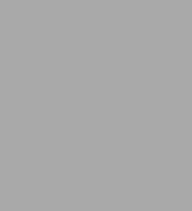
Learning with Kernels: Support Vector Machines, Regularization, Optimization, and Beyond
648Paperback(Reprint)
Product Details
ISBN-13: | 9780262536578 |
---|---|
Publisher: | MIT Press |
Publication date: | 06/05/2018 |
Series: | Adaptive Computation and Machine Learning series |
Edition description: | Reprint |
Pages: | 648 |
Product dimensions: | 8.00(w) x 10.00(h) x 1.06(d) |
Age Range: | 18 Years |