Logic for Learning: Learning Comprehensible Theories from Structured Data
This book is concerned with the rich and fruitful interplay between the fields of computational logic and machine learning. The intended audience is senior undergraduates, graduate students, and researchers in either of those fields. For those in computational logic, no previous knowledge of machine learning is assumed and, for those in machine learning, no previous knowledge of computational logic is assumed. The logic used throughout the book is a higher-order one. Higher-order logic is already heavily used in some parts of computer science, for example, theoretical computer science, functional programming, and hardware verification, mainly because of its great expressive power. Similar motivations apply here as well: higher-order functions can have other functions as arguments and this capability can be exploited to provide abstractions for knowledge representation, methods for constructing predicates, and a foundation for logic-based computation. The book should be of interest to researchers in machine learning, espe cially those who study learning methods for structured data. Machine learn ing applications are becoming increasingly concerned with applications for which the individuals that are the subject of learning have complex structure. Typical applications include text learning for the World Wide Web and bioinformatics. Traditional methods for such applications usually involve the extraction of features to reduce the problem to one of attribute-value learning.
1117669974
Logic for Learning: Learning Comprehensible Theories from Structured Data
This book is concerned with the rich and fruitful interplay between the fields of computational logic and machine learning. The intended audience is senior undergraduates, graduate students, and researchers in either of those fields. For those in computational logic, no previous knowledge of machine learning is assumed and, for those in machine learning, no previous knowledge of computational logic is assumed. The logic used throughout the book is a higher-order one. Higher-order logic is already heavily used in some parts of computer science, for example, theoretical computer science, functional programming, and hardware verification, mainly because of its great expressive power. Similar motivations apply here as well: higher-order functions can have other functions as arguments and this capability can be exploited to provide abstractions for knowledge representation, methods for constructing predicates, and a foundation for logic-based computation. The book should be of interest to researchers in machine learning, espe cially those who study learning methods for structured data. Machine learn ing applications are becoming increasingly concerned with applications for which the individuals that are the subject of learning have complex structure. Typical applications include text learning for the World Wide Web and bioinformatics. Traditional methods for such applications usually involve the extraction of features to reduce the problem to one of attribute-value learning.
54.99
In Stock
5
1
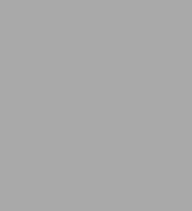
Logic for Learning: Learning Comprehensible Theories from Structured Data
257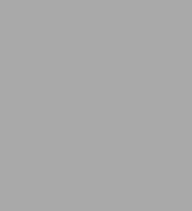
Logic for Learning: Learning Comprehensible Theories from Structured Data
257Hardcover(2003)
$54.99
54.99
In Stock
Product Details
ISBN-13: | 9783540420279 |
---|---|
Publisher: | Springer Berlin Heidelberg |
Publication date: | 09/17/2003 |
Series: | Cognitive Technologies |
Edition description: | 2003 |
Pages: | 257 |
Product dimensions: | 6.10(w) x 9.25(h) x 0.02(d) |
From the B&N Reads Blog