Elastic Stack, previously known as the ELK stack, is a log analysis solution that helps users ingest, process, and analyze search data effectively. With the addition of machine learning, a key commercial feature, the Elastic Stack makes this process even more efficient. This updated second edition of Machine Learning with the Elastic Stack provides a comprehensive overview of Elastic Stack's machine learning features for both time series data analysis as well as for classification, regression, and outlier detection.
The book starts by explaining machine learning concepts in an intuitive way. You'll then perform time series analysis on different types of data, such as log files, network flows, application metrics, and financial data. As you progress through the chapters, you'll deploy machine learning within Elastic Stack for logging, security, and metrics. Finally, you'll discover how data frame analysis opens up a whole new set of use cases that machine learning can help you with.
By the end of this Elastic Stack book, you'll have hands-on machine learning and Elastic Stack experience, along with the knowledge you need to incorporate machine learning in your distributed search and data analysis platform.
Elastic Stack, previously known as the ELK stack, is a log analysis solution that helps users ingest, process, and analyze search data effectively. With the addition of machine learning, a key commercial feature, the Elastic Stack makes this process even more efficient. This updated second edition of Machine Learning with the Elastic Stack provides a comprehensive overview of Elastic Stack's machine learning features for both time series data analysis as well as for classification, regression, and outlier detection.
The book starts by explaining machine learning concepts in an intuitive way. You'll then perform time series analysis on different types of data, such as log files, network flows, application metrics, and financial data. As you progress through the chapters, you'll deploy machine learning within Elastic Stack for logging, security, and metrics. Finally, you'll discover how data frame analysis opens up a whole new set of use cases that machine learning can help you with.
By the end of this Elastic Stack book, you'll have hands-on machine learning and Elastic Stack experience, along with the knowledge you need to incorporate machine learning in your distributed search and data analysis platform.
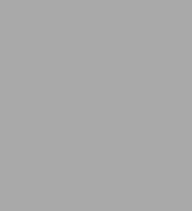
Machine Learning with the Elastic Stack: Gain valuable insights from your data with Elastic Stack's machine learning features
450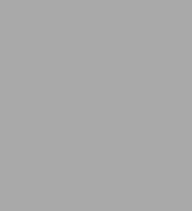
Machine Learning with the Elastic Stack: Gain valuable insights from your data with Elastic Stack's machine learning features
450Product Details
ISBN-13: | 9781801078467 |
---|---|
Publisher: | Packt Publishing |
Publication date: | 05/31/2021 |
Sold by: | Barnes & Noble |
Format: | eBook |
Pages: | 450 |
File size: | 31 MB |
Note: | This product may take a few minutes to download. |