Mathematical Foundations of Information Theory
The first comprehensive introduction to information theory, this book places the work begun by Shannon and continued by McMillan, Feinstein, and Khinchin on a rigorous mathematical basis. For the first time, mathematicians, statisticians, physicists, cyberneticists, and communications engineers are offered a lucid, comprehensive introduction to this rapidly growing field.
In his first paper, Dr. Khinchin develops the concept of entropy in probability theory as a measure of uncertainty of a finite “scheme,” and discusses a simple application to coding theory. The second paper investigates the restrictions previously placed on the study of sources, channels, and codes and attempts “to give a complete, detailed proof of both … Shannon theorems, assuming any ergodic source and any stationary channel with a finite memory.”
Partial Contents: I. The Entropy Concept in Probability Theory — Entropy of Finite Schemes. The Uniqueness Theorem. Entropy of Markov chains. Application to Coding Theory. II. On the Fundamental Theorems of Information Theory — Two generalizations of Shannon’s inequality. Three inequalities of Feinstein. Concept of a source. Stationarity. Entropy. Ergodic sources. The E property. The martingale concept. Noise. Anticipation and memory. Connection of the channel to the source. Feinstein’s Fundamental Lemma. Coding. The first Shannon theorem. The second Shannon theorem.
"1103268985"
The first comprehensive introduction to information theory, this book places the work begun by Shannon and continued by McMillan, Feinstein, and Khinchin on a rigorous mathematical basis. For the first time, mathematicians, statisticians, physicists, cyberneticists, and communications engineers are offered a lucid, comprehensive introduction to this rapidly growing field.
In his first paper, Dr. Khinchin develops the concept of entropy in probability theory as a measure of uncertainty of a finite “scheme,” and discusses a simple application to coding theory. The second paper investigates the restrictions previously placed on the study of sources, channels, and codes and attempts “to give a complete, detailed proof of both … Shannon theorems, assuming any ergodic source and any stationary channel with a finite memory.”
Partial Contents: I. The Entropy Concept in Probability Theory — Entropy of Finite Schemes. The Uniqueness Theorem. Entropy of Markov chains. Application to Coding Theory. II. On the Fundamental Theorems of Information Theory — Two generalizations of Shannon’s inequality. Three inequalities of Feinstein. Concept of a source. Stationarity. Entropy. Ergodic sources. The E property. The martingale concept. Noise. Anticipation and memory. Connection of the channel to the source. Feinstein’s Fundamental Lemma. Coding. The first Shannon theorem. The second Shannon theorem.
Mathematical Foundations of Information Theory
The first comprehensive introduction to information theory, this book places the work begun by Shannon and continued by McMillan, Feinstein, and Khinchin on a rigorous mathematical basis. For the first time, mathematicians, statisticians, physicists, cyberneticists, and communications engineers are offered a lucid, comprehensive introduction to this rapidly growing field.
In his first paper, Dr. Khinchin develops the concept of entropy in probability theory as a measure of uncertainty of a finite “scheme,” and discusses a simple application to coding theory. The second paper investigates the restrictions previously placed on the study of sources, channels, and codes and attempts “to give a complete, detailed proof of both … Shannon theorems, assuming any ergodic source and any stationary channel with a finite memory.”
Partial Contents: I. The Entropy Concept in Probability Theory — Entropy of Finite Schemes. The Uniqueness Theorem. Entropy of Markov chains. Application to Coding Theory. II. On the Fundamental Theorems of Information Theory — Two generalizations of Shannon’s inequality. Three inequalities of Feinstein. Concept of a source. Stationarity. Entropy. Ergodic sources. The E property. The martingale concept. Noise. Anticipation and memory. Connection of the channel to the source. Feinstein’s Fundamental Lemma. Coding. The first Shannon theorem. The second Shannon theorem.
The first comprehensive introduction to information theory, this book places the work begun by Shannon and continued by McMillan, Feinstein, and Khinchin on a rigorous mathematical basis. For the first time, mathematicians, statisticians, physicists, cyberneticists, and communications engineers are offered a lucid, comprehensive introduction to this rapidly growing field.
In his first paper, Dr. Khinchin develops the concept of entropy in probability theory as a measure of uncertainty of a finite “scheme,” and discusses a simple application to coding theory. The second paper investigates the restrictions previously placed on the study of sources, channels, and codes and attempts “to give a complete, detailed proof of both … Shannon theorems, assuming any ergodic source and any stationary channel with a finite memory.”
Partial Contents: I. The Entropy Concept in Probability Theory — Entropy of Finite Schemes. The Uniqueness Theorem. Entropy of Markov chains. Application to Coding Theory. II. On the Fundamental Theorems of Information Theory — Two generalizations of Shannon’s inequality. Three inequalities of Feinstein. Concept of a source. Stationarity. Entropy. Ergodic sources. The E property. The martingale concept. Noise. Anticipation and memory. Connection of the channel to the source. Feinstein’s Fundamental Lemma. Coding. The first Shannon theorem. The second Shannon theorem.
10.95
In Stock
5
1
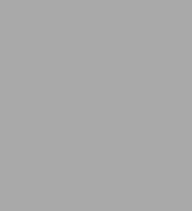
Mathematical Foundations of Information Theory
128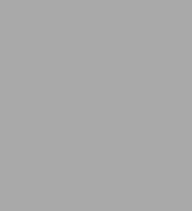
Mathematical Foundations of Information Theory
128
10.95
In Stock
Product Details
ISBN-13: | 9780486604343 |
---|---|
Publisher: | Dover Publications |
Publication date: | 06/01/1957 |
Series: | Dover Books on Mathematics Series |
Pages: | 128 |
Product dimensions: | 5.50(w) x 8.50(h) x (d) |
From the B&N Reads Blog