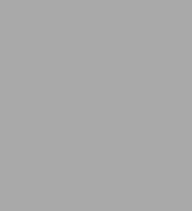
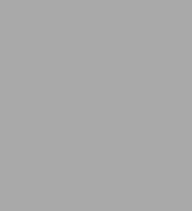
eBook
Available on Compatible NOOK devices, the free NOOK App and in My Digital Library.
Related collections and offers
Overview
Product Details
ISBN-13: | 9780486167657 |
---|---|
Publisher: | Dover Publications |
Publication date: | 01/24/2013 |
Series: | Dover Books on Mathematics |
Sold by: | Barnes & Noble |
Format: | eBook |
Pages: | 256 |
File size: | 11 MB |
Note: | This product may take a few minutes to download. |
Read an Excerpt
Mathematical Foundations of Quantum Statistics
By A.Y. Khinchin
Dover Publications, Inc.
Copyright © 1960 Graylock PressAll rights reserved.
ISBN: 978-0-486-16765-7
CHAPTER 1
PRELIMINARY CONCEPTS OF THE THEORY OF PROBABILITY
§1. Integral-valued random variables
This book is concerned with the rigorous and detailed mathematical bases of the most important formulas of quantum statistics. These are established with the help of the limit theorems of the theory of probability, since the question of limit theorems of some particular type arises in all cases. For a long time these theorems have been of interest to specialists and in recent years they have been developed significantly, particularly by mathematicians in the U. S. S. R. Nevertheless they are not, as a rule, discussed in textbooks and consequently are little known to a wide circle of scholars. (As an exception we may mention the book by von Mises.) Hence, in the present chapter we give both detailed formulations and complete proofs of the limit theorems which are necessary for our development. We assume only that the reader is acquainted with a general text such as Feller.
The type of limit theorem we need is distinguished by the following important specific characteristics:
1) We always consider random variables all of whose possible values are integers;
2) All the limit theorems of interest to us are of the local type, i.e., we always consider an asymptotic estimate of the probability that the sum of the random variables being studied assume some definite value;
3) We can limit ourselves to sums of mutually independent and identically distributed random variables that have finite moments up to the fifth order inclusive;
4) In all cases we must find not only an asymptotic formula, but also an accurate estimate of the error;
5) Finally, in addition to the one-dimensional limit theorems, we will be equally interested in multi-dimensional limit theorems of the same type (in particular, two-dimensional limit theorems).
Local limit theorems for integral-valued random variables attracted the attention of investigators a relatively long time ago, although much less effort was devoted to them than to theorems of the "integral" type. Thus, in the book by von Mises, one may find rather deep theorems of this latter type. However, a sufficiently general formulation of the problems of interest to us has been achieved only recently. In particular, the limit theorems of the type we require were first proved by B. V. Gnedenko and his students. Although they considered multi-dimensional problems, the fundamental direction of their investigations differs significantly in one way from that which we need: While Gnedenko and his students always sought more general conditions under which the fundamental limiting relationship is valid, we, as stated above, can confine ourselves to a very narrow class of initial distributions. On the other hand, we cannot be satisfied with deriving limiting relationships, but must estimate the resulting error, sometimes rather accurately. Thus, although Gnedenko's methods are completely adequate for our purpose, we need formulations of limit theorems which are somewhat different from those given by him and his co-workers. This is a further reason for our including a chapter containing detailed proofs of the limit theorems we require.
The random variables we must consider in this book always have only integers as their possible values. We call such random variables integral-valued. Evidently, the distribution law of the integral-valued random variable [xi] is completely determined by giving for each integer n the probability
[MATHEMATICAL EXPRESSION NOT REPRODUCIBLE IN ASCII],
that the variable [xi] take on the value n. In the future we shall say briefly that the variable [xi] obeys (is subject to) the law pn or is distributed according to the law pn.
If the series
[MATHEMATICAL EXPRESSION NOT REPRODUCIBLE IN ASCII]
converges absolutely, its sum is called the mathematical expectation E]xi] of the variable [xi].(Sometimes, instead of mathematical expectation, the term "mean value" of the random variable V is used. We carefully avoid this terminology, since the term "mean value" has a completely different meaning in this book.) In general, given an absolutely convergent series
[MATHEMATICAL EXPRESSION NOT REPRODUCIBLE IN ASCII],
where (n) is an arbitrary real or complex function of the integral argument n, we call the sum of the series the mathematical expectation Ef ([xi]) of the random variable f ([xi]) In particular, the mathematical expectation E]xi]k of the variable [xi]k (if it exists) is called the moment of order k (kth moment) of the variable [xi]. The mathematical expectation E([xi] - E]xi])k of the variable ([xi] - E]xi])k (if it exists) is called the central moment of order k of the variable [xi]. The central moment of second order
[MATHEMATICAL EXPRESSION NOT REPRODUCIBLE IN ASCII]
(if it exists) is called the dispersion (variance) of the variable [xi] and is, along with the mathematical expectation E]xi], one of the most important characteristics of this variable. All the random variables we shall consider actually possess moments of arbitrary order k ≥ 0. However, we shall see that for the proof of the relevant limit theorems, it is sufficient to assume the existence of moments of only relatively low orders.
If [xi]' and [xi]" are integral-valued random variables obeying, respectively, the laws pn' and pn", then the sum [xi]' + [xi]" = [xi] is an integral-valued random variable. The distribution law pn of this sum, in addition to depending on the laws pn' and pn", also depends on the form of the mutual dependence of the variable [xi]' and [xi]". In particular, if these two variables are mutually independent, then the numbers pn are very simply expressed in terms of the numbers pn' and pn". Indeed, in order that [xi] = n, it is necessary and sufficient that [xi]' = k, [xi]" = n - k, where k is any integer. Therefore,
[MATHEMATICAL EXPRESSION NOT REPRODUCIBLE IN ASCII],
and in virtue of the mutual independence of the variables [xi]' and [xi]",
[MATHEMATICAL EXPRESSION NOT REPRODUCIBLE IN ASCII].
The last equation may be rewritten as
[MATHEMATICAL EXPRESSION NOT REPRODUCIBLE IN ASCII].
In the same fashion if we have s mutually independent integral-valued random variables
[xi](1), [xi](2), .., [xi](s)
with the corresponding distribution laws
[MATHEMATICAL EXPRESSION NOT REPRODUCIBLE IN ASCII],
then the distribution law pn of the sum
[MATHEMATICAL EXPRESSION NOT REPRODUCIBLE IN ASCII]
may be expressed as
(1)
[MATHEMATICAL EXPRESSION NOT REPRODUCIBLE IN ASCII],
where [MATHEMATICAL EXPRESSION NOT REPRODUCIBLE IN ASCII]; or, equivalently,
(2)
[MATHEMATICAL EXPRESSION NOT REPRODUCIBLE IN ASCII],
where [MATHEMATICAL EXPRESSION NOT REPRODUCIBLE IN ASCII]. The expression for the distribution of the sum of mutually independent random variables in terms of the distributions of the summands is called the rule of composition of these distributions. Thus, formulas (1) and (2) express the rule of composition of the distributions of an arbitrary number of integral-valued random variables.
It is known from the elementary theory of probability that the mathematical expectation of a sum of an arbitrary number of random variables is always equal to the sum of their mathematical expectations. If the summands are mutually independent, the analogous law holds true for the products. Finally, the dispersion of the sum is equal to the sum of the dispersions of the summands when the summands are pairwise mutually independent.
We must repeatedly consider cases in which the basic element is not one random variable, but a family of several (two, three or more) mutually dependent integral-valued random variables [xi], η,.... For simplicity of notation we consider the case of a pair ([xi], η) of such variables. (All that is said for this case holds true with the corresponding obvious changes for any larger family of variables.) A pair of this type is sometimes called a (two-dimensional) random vector. The probability P ([xi] = l, η = m) of the simultaneous realization of the equations [xi] = l and η = m is denoted by plm. The set of numbers plm (- ∞ < l, m< ∞) forms the distribution law of the random pair (η, η). If pn and qm, respectively, denote the distribution laws of the variables and η, then evidently
(3)
[MATHEMATICAL EXPRESSION NOT REPRODUCIBLE IN ASCII].
Hence,
[MATHEMATICAL EXPRESSION NOT REPRODUCIBLE IN ASCII],
and analogously,
[MATHEMATICAL EXPRESSION NOT REPRODUCIBLE IN ASCII].
It is assumed that all these series converge absolutely.
If f ([xi], η) is an arbitrary real or complex function of the variables [xi] and ηITL, then the quantity
(4)
[MATHEMATICAL EXPRESSION NOT REPRODUCIBLE IN ASCII]
is called its mathematical expectation if the double series converges absolutely. In particular, from formulas (3) or (4) we obtain expressions for the dispersion of the variables [xi] and η in terms of the numbers plm:
[MATHEMATICAL EXPRESSION NOT REPRODUCIBLE IN ASCII].
The ratio
[MATHEMATICAL EXPRESSION NOT REPRODUCIBLE IN ASCII]
is called the correlation coefficient of the variables [xi] and η. The numerator of this ratio can be written in the form
[MATHEMATICAL EXPRESSION NOT REPRODUCIBLE IN ASCII].
From the random pairs ([xi]', η') and ([xi]", η") we may form the pair ([xi]' + [xi]", η' + [etea]") which is called the sum of the given pairs. In the same fashion, the sum of an arbitrary number of pairs can be defined. Let plm'., plm", plm denote the distributions of the pairs ([xi]', η'), ([xi]", η"), ([xi]' + [xi]", η' + η"), respectively. The numbers plm are, in general, not completely defined by specifying the numbers plm' and plm"; for this it is also necessary to know the dependence between the pairs ([xi]', η') and ([xi]", η"). In the most important case, when the latter pairs are mutually independent (i.e., when the values taken by the variables [xi]', η' do not depend on the law plm", and conversely) we easily obtain the rule of composition expressing the numbers plm in terms of the numbers plm' and plm". Moreover, we can obtain the rule of composition for the addition of an arbitrary number of (mutually independent) pairs. These formulas (which we shall not introduce here) are completely analogous to formulas (1) and (2), which were established above for the one-dimensional case, but are, of course, substantially more complicated than (1) and (2).
§2. Limit theorems
In the theory of probability, as in every mathematical theory of a natural science, such as theoretical mechanics, thermodynamics and many others, one tries to establish conformance to the most general principles. These principles would relate not only to the particular processes taking place in nature and in human practice, but would include the widest possible class of phenomena. For instance, the fundamental theorems of mechanics — the theorem of kinetic energy, the (Keplerian) theorem of areas, etc. — are not related to any special form of mechanical motion, but to an extremely wide class of such motions. In the same way, the fundamental propositions of the theory of probability (such as the law of large numbers) not only include special forms of mass phenomena, but include extremely wide classes of them. It can be said that the essence of mass phenomena is revealed in regularities of this type, i.e., those properties of these phenomena are revealed which are due to their mass character, but which depend in only a relatively slight manner on the individual nature of the objects composing the masses. For example, sums of random variables, the individual terms of which may be distributed according to any of a wide range of laws, obey the law of large numbers; neither the applicability nor the content of the law of large numbers depends upon these individual distribution laws (which must satisfy only certain very general requirements).
One of the most important parts of the theory of probability — the theory of limit theorems — was developed because of this desire to establish general principles of a type which includes the widest possible class of real phenomena. In a very large number of cases — in particular, in the simplest problems which arose initially — the mass character of the phenomenon being studied was taken into account mathematically by investigating sums of a very large number of random variables (more or less equally significant and mutually dependent or independent). Thus, in the theory of measurement errors (one of the first applications of the theory of probability) we study the error actually incurred in performing a measurement; this error is usually the sum of a large number of individual errors caused by very different factors. The law of large numbers is concerned with just such sums of a large number of random variables. In the XVIIIth century, De Moivre and Laplace showed that in some of the simplest cases the sums of large numbers of mutually independent random variables, after proper normalization, were subject to distribution laws which approach the so-called "normal" law as the number of terms approaches infinity. The "density" of this law is given by the function
[MATHEMATICAL EXPRESSION NOT REPRODUCIBLE IN ASCII].
This was the first of the limit theorems of the theory of probability, the so-called theorem of De Moivre and Laplace. It is now studied in all courses on the theory of probability. This theorem includes only an extremely narrow class of cases, the so-called Bernoulli trials, where each term has as its possible values only the numbers 0 and 1, and the probabilities of these values are the same for all terms. However, as was stated by Laplace, the causes, due to which distribution laws of sums in the case of Bernoulli trials have a tendency to approach the normal law, have a character so general that there is every reason to suppose that the theorem of De Moivre and Laplace is merely a special case of some much more general principle. Laplace attempted to find the basis for this tendency to the normal law for a wider class of situations. However, neither he nor his contemporaries made significant progress in this direction, partly because the methods of mathematical analysis known at that time were inadequate for this purpose. The first method, by which it was possible to prove that the limit theorem is a general principle governing the behavior of sums of a large number of mutually independent random variables, was not formulated until the middle of the XIXth century by P. L. Chebyshev, the great Russian scholar. It is well-known that the first general conception of the law of large numbers is due to him. In general, the desire to establish principles of wide validity, which is common to every natural science and of which we spoke at the beginning of the present section, was noticeable in the theory of probability only after the investigations of Chebyshev.
Chebyshev tried to formulate a general limit theorem during almost all of his scientific life. He finally found a suitable formulation, but did not succeed in proving the theorem itself. The proof was completed shortly after Chebyshev's death by his student and successor A. A. Markov. However, several years before the work of Markov, A. M. Liapunov, who was also a student of Chebyshev, proved the limit theorem under extremely general conditions by a different method, which more closely resembles the contemporary proof.
(Continues...)
Excerpted from Mathematical Foundations of Quantum Statistics by A.Y. Khinchin. Copyright © 1960 Graylock Press. Excerpted by permission of Dover Publications, Inc..
All rights reserved. No part of this excerpt may be reproduced or reprinted without permission in writing from the publisher.
Excerpts are provided by Dial-A-Book Inc. solely for the personal use of visitors to this web site.
Table of Contents
PrefaceINTRODUCTIONCHAPTER I. PRELIMINARY CONCEPTS OF THE THEORY OF PROBABILITY
CHAPTER II. PRELIMINARY CONCEPTS OF QUANTUM MECHANICS
CHAPTER III. GENERAL PRINCIPLES OF QUANTUM STATISTICS
CHAPTER IV. THE FOUNDATIONS OF THE STATISTICS OF PHOTONS
CHAPTER V. FOUNDATIONS OF THE STATISTICS OF MATERIAL PARTICLES
CHAPTER VI. THERMODYNAMIC CONCLUSIONS
Supplement I. THE STATISTICS OF HETEROGENEOUS SYSTEMSSupplement I I. THE DISTRIBUTION OF A COMPONENT AND ITS ENERGY Supplement III. THE PRINCIPLE OF CANONICAL AVERAGINGSupplement IV. THE REDUCTION TO A ONE-DIMENSIONAL PROBLEM IN THE CASE OF COMPLETE STATISTICSSupplement V. SOME GENERAL THEOREMS OF STATISTICAL PHYSICSSupplement VI. SYMMETRIC FUNCTIONS ON MULTI-DIMENSIONAL SURFACESReferencesIndex