Teaching biological concepts from which models can be developed, Richard McElreath and Robert Boyd introduce readers to many of the typical mathematical tools that are used to analyze evolutionary models and end each chapter with a set of problems that draw upon these techniques. Mathematical Models of Social Evolution equips behaviorists and evolutionary biologists with the mathematical knowledge to truly understand the models on which their research depends. Ultimately, McElreath and Boyd’s goal is to impart the fundamental concepts that underlie modern biological understandings of the evolution of behavior so that readers will be able to more fully appreciate journal articles and scientific literature, and start building models of their own.
Teaching biological concepts from which models can be developed, Richard McElreath and Robert Boyd introduce readers to many of the typical mathematical tools that are used to analyze evolutionary models and end each chapter with a set of problems that draw upon these techniques. Mathematical Models of Social Evolution equips behaviorists and evolutionary biologists with the mathematical knowledge to truly understand the models on which their research depends. Ultimately, McElreath and Boyd’s goal is to impart the fundamental concepts that underlie modern biological understandings of the evolution of behavior so that readers will be able to more fully appreciate journal articles and scientific literature, and start building models of their own.
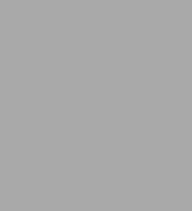
Mathematical Models of Social Evolution: A Guide for the Perplexed
432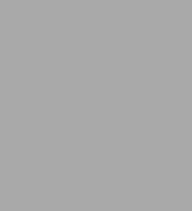
Mathematical Models of Social Evolution: A Guide for the Perplexed
432Hardcover
-
SHIP THIS ITEMTemporarily Out of Stock Online
-
PICK UP IN STORE
Your local store may have stock of this item.
Available within 2 business hours
Related collections and offers
Overview
Teaching biological concepts from which models can be developed, Richard McElreath and Robert Boyd introduce readers to many of the typical mathematical tools that are used to analyze evolutionary models and end each chapter with a set of problems that draw upon these techniques. Mathematical Models of Social Evolution equips behaviorists and evolutionary biologists with the mathematical knowledge to truly understand the models on which their research depends. Ultimately, McElreath and Boyd’s goal is to impart the fundamental concepts that underlie modern biological understandings of the evolution of behavior so that readers will be able to more fully appreciate journal articles and scientific literature, and start building models of their own.
Product Details
ISBN-13: | 9780226558264 |
---|---|
Publisher: | University of Chicago Press |
Publication date: | 03/15/2007 |
Pages: | 432 |
Product dimensions: | 6.00(w) x 9.00(h) x 1.10(d) |
About the Author
Read an Excerpt
Mathematical Models of Social Evolution A Guide for the Perplexed
By Richard McElreath Robert Boyd
The University of Chicago Press Copyright © 2007 The University of Chicago
All right reserved.
ISBN: 978-0-226-55827-1
Chapter One The Theoretician's Laboratory
Mathematical models and the tools used to analyze them constitute the theoretician's laboratory. Simple mathematical models are experiments aimed at understanding the causal relationships that drive important natural phenomena. Theoreticians in evolutionary biology use a variety of tools to study such models, divining their secrets to reveal how interactions that take place over long time spans shape the evolution of behavior. These models are almost always too simple to make accurate predictions or even accurately represent how any real behavior evolves. Nonetheless, they have proven to be extremely valuable because they help us understand processes too complex to grasp by verbal reasoning alone.
Consequently, evolutionary biology has been greatly influenced by the use of such "experiments." In order to read the primary literature in evolutionary biology or animal behavior, you have to be able to really understand mathematical models. Unfortunately, few programs adequately prepare even advanced graduate students to read and understand mathematical theory. Even when research is largely empirical, predictions are often derived from mathematical models, and students of animal behavior-be the animals humans or otherwise-are handicapped by not fully understanding the theory from which the empirical work derives. What makes it worse is that having a good education in mathematics or probability, while certainly helpful, isn't enough to understand evolutionary models. One needs to acquire a unique toolbox of ideas for dealing with formal population dynamics.
As economists, anthropologists, and others outside biology have been drawn to this body of theory over the last few decades, the problem has become more acute. Now social scientists of many flavors are struggling with mathematical theories they never encountered in their own fields. Often, we are told that someone has shown some result mathematically, and we will just have to accept it. Sometimes, this leads to uncritical adoption of theory. Other times, it leads to uncritical rejection. Neither is an acceptable state of affairs. Both kin selection (Chapter 3) and reciprocal altruism (Chapter 4), topics central to the evolution of social behavior, are cases where the gulf between theorists and consumers of theory is far too wide.
This book aims to help make the student or professional researcher in biology or the social sciences conversant in the language of the evolutionary theory of social behavior. It assumes that the student can do algebra and has had an introduction to calculus but maybe has forgotten much of it. It covers many of the basic topics: contests and conflicts, kin selection, reciprocity, signaling, multilevel selection, sex allocation, and sexual selection. At the same time, readers will be introduced to many of the typical mathematical tools that are used to analyze evolutionary models. At the end of each chapter, we provide a set of problems that elaborate upon the material in the chapter and allow the reader to practice the techniques we describe. The solutions to these problems are found at the end of the book. Those who work through the book and do all the problems should have a reasonable grasp on the fundamental concepts that underly modern biology's understanding of the evolution of behavior, be able to intelligently and critically read papers in journals like Animal Behaviour or Proceedings of the Royal Society, Series B, and with a little more practice maybe start building models of their own.
1.1 The structure of evolutionary theory
When Darwin left for his voyage around the world on the Beagle, he took with him the first volume of Charles Lyell's Principles of Geology. Somewhere in South America, he received the second volume by post. Lyell is famous for, among other things, never accepting Darwin's account of evolution by natural selection, apparently because of his religious beliefs. There is a sad irony here because Lyell's work played a crucial role in the development of Darwin's thinking. In fact, in some ways Lyell's principle of uniformitarianism is as central to Darwinism as is natural selection.
Before Lyell, it was common to explain the features of the earth's geology in terms of past catastrophes: floods, earthquakes, and other cataclysms. In contrast, Lyell tried to explain what he observed in terms of the cumulative action of processes that he could observe every day in the world around us-the sinking of lands, the building up of sediments, and so on. By appreciating the accumulated small effects of such processes over long time spans, great changes could be explained. Darwin took this idea and applied it to populations of organisms.
Darwin was a good naturalist and knew a lot about the everyday lives of plants and animals. They mate, they give birth, they move from one place to another, and they die. Darwin's great insight was to see that organisms vary and that the processes of their lives affect which types spread and which vanish. The key to explaining long-run change in nature was to apply Lyell's principle of uniformitarianism to populations. By keeping track of how the small events of everyday life change the composition of populations, we can explain great events over long time scales.
Biologists have been thinking this way ever since Darwin, but it is still news in some fields. Are people products of their societies or are societies products of people? The answer must be "both," but theory in the social sciences has tended to take one side or the other. In evolutionary models, this classical conflict between explanations at the level of the society (think Durkheimian social facts) and explanations at the level of individuals (think micro-economics) simply disappears. Population models allow explanation and real causation at both levels (and more than two levels) to exist seamlessly and meaningfully in one theory. We don't have to choose between atomistic and group-level explanations. Instead, one can build models about how groups of individuals can create population-level effects which then change individuals in powerful ways. This aspect of evolutionary theory gives it great power to explain the evolution of behavior in both people and other animals.
1.2 The utility of simple models
The models you will analyze in this chapter, and in fact every model we consider in this book, are much simpler than the real systems that they represent. The populations are infinite (or at least very large), the organisms that populate them have highly stylized trait inheritance and reproduction, the generations are discrete and nonoverlapping, stochastic effects are largely nonexistent, and gene interactions are wholly absent.
Simple evolutionary models never come close to capturing all the detail in any real species or situation. Instead, game theory trades specificity and detail for tractability and clarity. Models are like maps-they are most useful when they contain the details of interest and ignore others. A map of subways helps a person to navigate by subway. A map of streets helps on the surface. A map with both subways and streets would be too crowded and confusing for either task. A passage from Lewis Carroll's Sylvie and Bruno Concluded illustrates this point:
"What do you consider the largest map that would be really useful?"
"About six inches to the mile."
"Only six inches!" exclaimed Mein Herr. "We very soon got six yards to the mile. Then we tried a hundred yards to the mile. And then came the grandest idea of all! We actually made a map of the country, on the scale of a mile to the mile!"
"Have you used it much?" I enquired.
"It has never been spread out, yet," said Mein Herr: "The farmers objected: they said it would cover the whole country, and shut out the sunlight! So now we use the country itself, as its own map, and I assure you it does nearly as well."
Game-theoretic models are simple maps for understanding the consequences of a small number of key assumptions. Despite their deliberate myopia, and perhaps as a result of it, they have proven quite useful in a number of fields, especially in the study of social behavior. Their simplicity limits their power, but it also allows them to do things for us that the real world cannot. For example, we can fit them in our pockets.
The models we will explore in this book are only as complicated as they must be to capture the structure of their problems. They always omit factors which are known to matter in the real world. Such omissions are intentional. It is accepted practice to control for variables in psychology or economics experiments, variables which are known matter in many important situations. But ignoring some (most!) variables allows experimenters to examine the effects of other variables in isolation. Simple models are much the same. They are not meant to be replicas of the real world, but rather to help us understand it, one piece at a time.
Simple models can aid our understanding of the world in several ways.
Existence proofs. There is usually a very large number of possible accounts of any particular biological or social phenomenon. And since much of the data needed to evaluate these accounts are lost or impossible to collect, it can be challenging to narrow down the field of possibilities. But models which formalize these accounts tell us which are internally consistent and when conclusions follow from their premises. With purely verbal arguments about evolutionary processes, it is all too often the case that our conclusions do not follow from our assumptions. Unaided reasoning about the mass effects of many weak forces operating over many generations has proved to be hazardous. Formalizing our arguments helps us understand which stories are possible explanations. They provide proof that some candidate set of processes could explain the observations of interest.
Aid communication. Formal models are much easier to present and explain. In almost every field in which theory has been important-philosophy, the natural sciences, economics-formal systems for communicating and inspecting theory have arisen. This is partly for the reasons just explained, but formal models are also important because they give us the ability to clearly communicate what we mean. The looseness of verbal models sometimes allows scholars to argue for years about their implications. Such wrangling is much rarer in mathematical disciplines because you have to precisely specify how every piece of the theory relates to every other piece. There is comfort in vagueness, and formal theory allows for little comfort. Sometimes, being forced to spell out an idea in detail is enough to make the formal exercise worth our time.
Counterintuitive results. Simple models often lead to surprising results. If such models always told us what we thought they would, there would be little point in constructing them. Instead, as any practicing theorist can tell you, models often surprise us, producing effects and interactions we hadn't imagined prior to constructing the model. These unanticipated effects lead to additional theory construction and data collection. They take our work in directions we would have missed had we stuck to verbal reasoning, and they help us to understand features of the system of study that were previously mysterious.
Prediction. Simple formal models can be used to make predictions about natural phenomena. In most cases, these predictions are qualitative. We might expect more or less of some effect as we increase the real-world analogue of a particular parameter of the model. For example, a model of voter turnout might predict that turnout increases in times of national crisis. The precise amount of increase per amount of crisis is very hard to predict, as deciding how to measure "crisis" on a quantitative scale seems like a life's work in itself. Even so, the model may provide a useful prediction of the direction of change. These sorts of predictions are sometimes called comparative statics. And even very simple models can tell us whether some effect should be linear, exponential, or some other functional form. All too often, scientists construct theories which imply highly nonlinear effects, yet they analyze their data with linear regression and analysis of variance. Allowing our models of the actual phenomenon to make predictions of our data can yield much more analytic power. In a few cases, such as sex allocation, models can make very precise quantitative predictions that can be applied to data.
But keep in mind that even when direct prediction from a model seems unlikely, models can direct our attention to things in the real world we should be measuring but had previously ignored. New data will then lead us to revise our models, leading in turn to more data collection. The relationship between models and data is not a one-way street. Data inspire and challenge theories just as often as theories inspire data collection. Or at least they should.
1.3 Why not just simulate?
There is a growing number of modelers who know very little about analytic methods. Instead, these researchers focus on computer simulations of complex systems. When computers were slow and memory was tight, simulation was not a realistic option. Without analytic methods, it would have taken years to simulate even moderately complex systems. With the rocketing ascent of computer speed and plummeting price of hardware, it has become increasingly easy to simulate very complex systems. This makes it tempting to give up on analytic methods, since most people find them difficult to learn and to understand.
There are several reasons why simulations are poor substitutes for analytic models.
Equations talk. Equations-given the proper training-really do speak to you. They provide intuitions about the reasons an evolutionary system behaves as it does, and these reasons can be read from the expressions that define the dynamics and resting states of the system. Analytic models therefore tell us things that we must infer, often with great difficulty, from simulation results. Analytic models can provide proofs, while simulations provide only a collection of examples.
Sensitivity analysis. It is difficult to explore the sensitivity of simulations to changes in parameter values. Parameters are quantities that specify assumptions of the model for a given run of the simulation-things like population size, mutation rate, and the value of a resource. In analytic models, the effects of these changes can be read directly from equations or by using various analytic techniques. In simulations, there are no analogous expressions. Instead, the analyst has to run a large number of simulations, varying the parameters in all combinations. For a small number of parameters, this may not be so bad. But let's assume a model has four parameters of interest, each of which has only 10 interesting values. Then we require [10.sup.4] simulations. If there are any stochastic effects in the model, we will need maybe 100 or 1000 or 10,000 times as many.
To see how time-consuming this can be, suppose we have a fairly fast computer that can complete a thorough single run of a simulation in 1 second. This may seem like a long time to run a simulation, but a nondeterministic, agent-based simulation can take a long time to run out enough generations to get a good picture of any steady state or dynamic cycles. Now, we will need [10.sup.4] = 10,000 different combinations of parameter values to entirely map out the parameter space, at the given intervals. Suppose we require 1000 simulations at each combination, to reliably estimate the mean outcome in each case. Now we are looking at [10.sup.7] simulations. At 1 second each, that's almost 116 days of computer time. No doubt computers will one day be fast enough to do 100 or more such simulations per second, but until then computer time is a major constraint.
In practice, people often ignore large portions of the parameter space, and so, many fewer simulations may be needed. There are often obvious and good reasons to do so. But even when large portions of the parameter space can be safely neglected, managing and interpreting the large amounts of data generated from the rest of the combinations can be a giant project, and this data-management problem will remain no matter how fast computers become in the future. Technology cannot save us here. When simple analytic methods can produce the same results, simulation should be avoided, both for economy and sanity.
(Continues...)
Excerpted from Mathematical Models of Social Evolution by Richard McElreath Robert Boyd Copyright © 2007 by The University of Chicago. Excerpted by permission.
All rights reserved. No part of this excerpt may be reproduced or reprinted without permission in writing from the publisher.
Excerpts are provided by Dial-A-Book Inc. solely for the personal use of visitors to this web site.
Table of Contents
Preface: How to use this book1 Theoretician’s Laboratory
1.1 Structure of evolutionary theory
1.2 The utility of simple models
1.3 Why not just simulate?
1.4 A model of viability selection
1.5 Determining long-term consequences
1.6 Non-genetic replication
2 Animal Conflict
2.1 The Hawk-Dove game
2.2 Retaliation
2.3 Continuous stable strategies
2.4 Ownership
2.5 Resource holding power
2.6 Sequential play
3 Altruism & Inclusive Fitness
3.1 The prisoner’s dilemma
3.2 Positive assortment
3.3 Common descent and inclusive fitness
3.4 Rediscovering Hamilton’s rule
3.5 Justifying Hamilton’s rule
3.6 Using Hamilton’s rule
4 Reciprocity
4.1 The Axelrod-Hamilton model
4.2 Mutants and mistakes
4.3 Partner Choice
4.4 Indirect Reciprocity
4.5 Reciprocity & Collective Action
5 Animal Communication
5.1 Costly signaling theory
5.2 Cheap honest signals
5.3 Signaling and altruism
5.4 Social learning
6 Selection Among Groups
6.1 Three views of selection
6.2 Deriving the Price equation
6.3 Selection within & between groups
6.4 Dispersal
7 Sex Allocation
7.1 Fisher’s theory of sex allocation
7.2 Reproductive value & sex ratio
7.3 Using the Shaw-Mohler Theorem
7.4 Biased sex ratios
7.5 Breaking the Eigen Barrier
8 Sexual Selection
8.1 Quantitative genetic models
8.2 Fisher’s runaway process
8.3 Costly choice & sensory bias
8.4 Good genes and sexy sons
A Facts About Derivatives
B Facts About Random Variables
C Binomial Expectations
D Numerical Solution
E Solutions to Problems
Bibliography
Index