MESA and Trading Market Cycles: Forecasting and Trading Strategies from the Creator of MESA / Edition 2 available in Hardcover
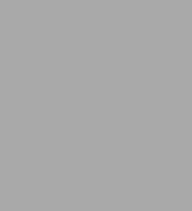
MESA and Trading Market Cycles: Forecasting and Trading Strategies from the Creator of MESA / Edition 2
- ISBN-10:
- 0471151963
- ISBN-13:
- 9780471151968
- Pub. Date:
- 02/25/2002
- Publisher:
- Wiley
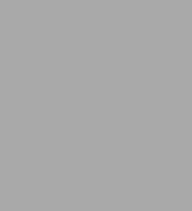
MESA and Trading Market Cycles: Forecasting and Trading Strategies from the Creator of MESA / Edition 2
Hardcover
Buy New
$90.00Overview
Product Details
ISBN-13: | 9780471151968 |
---|---|
Publisher: | Wiley |
Publication date: | 02/25/2002 |
Series: | Wiley Trading , #124 |
Edition description: | 2ND |
Pages: | 192 |
Product dimensions: | 6.26(w) x 9.39(h) x 0.82(d) |
About the Author
Read an Excerpt
MESA and Trading Market Cycles
Forecasting and Trading Strategies from the Creator of MESABy John F. Ehlers
John Wiley & Sons
ISBN: 0-471-15196-3Chapter One
WHY CYCLES EXIST IN THE MARKETTechnical analysis of the market is successful because the market is not always efficient. Discernible events that occur in chart patterns, such as double tops and Elliott waves, enable trading to be guided by technical analysis. Cycles are one of these discernible events that occur and are identifiable by direct measurement. Identification of cycles does not take a lifetime of experience or an expert system. Cycles can be measured directly, either by a simple system such as measuring the distance between successive lows or by a sophisticated computer software program such as MESA.
The fact that cycles exist does not imply that they exist all the time. Cycles come and go. External events sometimes dominate and obscure existing cycles. Experience shows that cycles useful for trading are present only about 15 to 30 percent of the time. This corresponds remarkably with J. M. Hurst's statement that "23% of all price motion is oscillatory in nature and semi-predictable." It is analogous to the problems of the trend-follower, who finds that the markets trend only a small percentage of the time.
Historical Perspective
Cyclic recurring processes observed in natural phenomena by humans since the earliest times have embedded the basic concepts used inmodern spectral estimation. Ancient civilizations were able to design calendars and time measures from their observations of the periodicities in the length of day, the length of the year, the seasonal changes, the phases of the moon, and the motion of the planets and stars. Pythagoras developed a relationship between the periodicity of musical notes produced by a fixed-tension string and a number representing the length of the string in the sixth century B.C. He believed that the essence of harmony was inherent in the numbers. Pythagoras extended the relationship to describe the harmonic motion of heavenly bodies, describing the motion as the "music of the spheres."
Sir Isaac Newton provided the mathematical basis for modern spectral analysis. In the seventeenth century, he discovered that sunlight passing through a glass prism expanded into a band of many colors. He determined that each color represented a particular wavelength of light and that the white light of the sun contained all wavelengths. He invented the word spectrum as a scientific term to describe the band of light colors.
Daniel Bernoulli developed the solution to the wave equation for the vibrating musical string in 1738. Later, in 1822, the French Engineer Jean-Baptiste-Joseph Fourier extended the wave equation results by asserting that any function could be represented as an infinite summation of sine and cosine terms. The mathematics of such representation has become known as harmonic analysis due to the harmonic relationship between the sine and cosine terms. Fourier transforms, the frequency description of time domain events (and vice versa), have been named in his honor.
Norbert Wiener provided the major turning point for the theory of spectral analysis in 1930, when he published his classic paper "Generalized Harmonic Analysis." Among his contributions were precise statistical definitions of autocorrelation and power spectral density for stationary random processes. The use of Fourier transforms, rather than the Fourier series of traditional harmonic analysis, enabled Wiener to define spectra in terms of a continuum of frequencies rather than as discrete harmonic frequencies.
John Tukey is the pioneer of modern empirical spectral analysis. In 1949 he provided the foundation for spectral estimation using correlation estimates produced from finite time sequences. Many of the terms of modern spectral estimation (such as aliasing, windowing, prewhitening, tapering, smoothing, and decimation) are attributed to Tukey. In 1965 he collaborated with Jim Cooley to describe an efficient algorithm for digital computation of the Fourier transform. This fast Fourier transform (FFT) unfortunately is not suitable for analysis of market data, as we will develop in later chapters.
The work of John Burg was the prime impetus for the current interest in high-resolution spectral estimation from limited time sequences. He described his high-resolution spectral estimate in terms of a maximum entropy formalism in his 1975 doctoral thesis and has been instrumental in the development of modeling approaches to high-resolution spectral estimation. Burg's approach was initially applied to the geophysical exploration for oil and gas through the analysis of seismic waves. The approach is also applicable for technical market analysis because it produces high-resolution spectral estimates using minimal data. This is important because the short-term market cycles are always shifting. Another benefit of the approach is that is maximally responsive to the selected data length and is not subject to distortions due to end effects at the ends of the data. The trading program, MESA, is an acronym for maximum entropy spectral analysis.
What Is a Cycle?
The dictionary definition of a cycle is that it is "an interval or space of time in which is completed one round of events or phenomena that recur regularly and in the same sequence." In the market, we consider a classic cycle exists when the price starts low, rises smoothly to a high over a length of time, and then smoothly falls back to the original price over the same length of time. The time required to complete the cycle is called the period of the cycle, or the cycle length.
Cycles certainly exist in the market. Many times they are justified on the basis of fundamental considerations. The clearest is the seasonal change for agricultural prices (lowest at harvest), or the decline in real estate prices in the winter. Television analysts are always talking about the rate of inflation being "seasonally adjusted" by the government. But the seasonal is a specific case of the cycle, always being 12 months. Other fundamentals-related cycles can originate from the 18-month cattle-breeding cycle or the monthly cold-storage report on pork bellies.
Business cycles are not as clear, but they exist. Business cycles vary with interest rates. The government sets objectives for economic growth based on its ability to hold inflation to reasonable levels. This growth is increased or decreased by adding or withdrawing funds from the economy and by changing the rate at which government lends money to banks. Easing of rates encourages business; tightening of rates inhibits it. Inevitably this process alternates, causing what we see as a business cycle. Although in practice this cycle may repeat in the same number of years, the exact repetition of the period is not necessary. The business cycle is limited on the upside by the amount of growth the government will allow (usually 3 percent) and on the downside by moderate negative growth (about ?1 percent), which indicates a recession. The range of the cycle from ?3 to ?1 percent is called its amplitude.
Components of the Market
Statisticians and economists have identified four important characteristics of price movement. All price forecasts and analyses deal with each of these elements:
1. A trend, or a tendency to move in one direction for a specified time period.
2. A seasonal factor, a pattern related to the calendar.
3. A cycle (other than seasonal) that may exist due to government action, the lag in starting up and winding down of business, or crop estimate announcements.
4. Other unaccountable price movement, often called noise.
Since points 2 and 3 are both cycles, it is clear that cycles are a significant and accepted part of all price movement.
When trading using cycles, one key question is the desired time span of the trade. At one extreme, the 54-year Kondratieff economic cycle (not without its critics) could be considered. A cattle rancher might prefer the 18-month breeding cycle, while a grain farmer probably hedges on the basis of the annual harvest. Speculators often work over a short (sometimes very short) time span.
Behavioral cycles in prices have been most popular in Elliott's wave theory and more recently in the works of Gann. But these methods have a large element of interpretation and subjectivity.
Short-term cycles can exist even within the definition of point 4, "noise." A casual glance at almost any bar chart shows, in retrospect, that short-term cycles ebb and flow. The ability to isolate and use market phenomena, such as cycles, is related to the awareness of its existence and the tools available. Many forecasting methods were not practical until the computer became popular. Now these methods can be used by nearly everyone. The philosophical foundation for these short-term cycles is derived from the random walk theory and is developed so you will feel more comfortable dealing with cycles within the constraints of point 4.
Random Walk
Randomness in the market results from a large number of traders exercising their prerogatives with different motivations of profit, loss, greed, fear, and entertainment; it is complicated by different perspectives of time. Market movement can therefore be analyzed in terms of random variables. One such analysis is the random walk. Imagine an atom of oxygen in a plastic box containing nothing but air. The path of this atom is erratic as it bounces from one molecule to another. Brownian motion is used to describe the way the atom moves. Its path is described as a three-dimensional random walk. Following such a random walk, the position of that atom is just as likely to be at any one location in that box as at any other.
Another form of the random walk is more appropriate for describing the motion of the market. This form is a two-dimensional random walk, called the "Drunkard's Walk." The two-dimensional structure is appropriate for the market because the prices can only go up or down in one dimension. The other dimension, time, can only move forward. These are similar to the way a drunkard's walk is described.
Diffusion Equation
The Drunkard's Walk is formulated by allowing the drunkard to step to either the right or left randomly with each step forward. To ensure randomness, the decision to step right or left is made on the outcome of a coin toss from a fair coin. If the coin turns up heads, the drunk steps to the right. If the coin turns up tails, the drunk steps to the left. Viewed from above, we see the random path the drunk has followed. Figure 1.1 shows a computer-generated path using the Drunkard's Walk rules. We can write a differential equation for this path because the rate change of time is related to the rate change of position in two dimensions.
Differential equations are used to describe relationships due to variations. For example, velocity is the change of distance with respect to time, such as miles per hour. Written as a differential equation, velocity is expressed as
V = dx/dt
so that the equation shows that velocity is the change of distance with respect to time. Think of the d in the equation as meaning the difference. Similarly, acceleration is the change of velocity with respect to time. The equation for acceleration becomes
a = dV/dt
Since velocity is the change of distance with respect to time, we can think of acceleration as being the second rate change of distance with respect to time. Now the equation for acceleration can be written as
a = dV/dt = [d.sup.2]x/d[t.sup.2]
Mathematicians use these formats when writing differential equations.
Writing out the Drunkard's Walk problem, the differential equation is
dP/dt = D * [d.sup.2]P/d[x.sup.2]
where P = the position in time and space D = the diffusion constant
This relatively famous differential equation (among mathematicians, at least) is known as the diffusion equation. In words, this equation states that the change of position with respect to time is proportional to the second rate change of position with respect to space. It describes many natural phenomena (e.g., the way heat travels up a silver spoon when it's placed in a hot cup of coffee). A better analogy to the way the market works is that the diffusion equation can describe the plume of smoke coming from a smokestack. Figure 1.2 shows 100 overlaid computer-generated Drunkard's Walk paths. Using some imagination, you can picture Figure 1.2 as a plume of smoke.
Picture this plume of smoke in a gentle breeze. The plume is roughly conical, widening with greater distance from the smokestack. The plume is bent in the direction of the breeze. The diffusion equation describes the position of a single smoke particle, and you see the random position of all the particles in macrospect. Due to the random nature of the variable, the best estimate of the position you can make for any particle is the average position of the plume. There clearly are no cycles involved. Relating the smoke plume to the market, the general direction can be determined by averaging the random price. This, of course, is the moving average. It identifies the trend as surely as you can see the bending of the smoke plume in the breeze. Several centuries ago Gauss proved that such an average is the best estimator for a truly random variable. Note that the estimation, or prediction, degrades with distance from the origin just as the smoke plume widens as it leaves the smokestack. For this reason, moving average predictors for the market trend to degrade rapidly, as do most forecasts.
Telegrapher's Equation
Let's revisit the mathematical formulation of the Drunkard's Walk problem. This time, the result of the coin flip will determine whether the drunk takes the next step in the same direction as the previous one or whether he reverses his direction. This makes the random variable his momentum rather than direction. Figure 1.3 shows a computer-generated Drunkard's Walk path formed using momentum as the random variable. Mathematicians call this the Continuous-Time Random Walk, or CTRW. In this case, the random variable is his momentum rather than his direction. We have altered the way his position changes as a function of time. When we now express his position as a differential equation, we obtain
[d.sup.2]P/d[t.sup.2] + (1/T) * dP/dt = C * [d.sup.2]P/d[x.sup.2]
where T and ITLITL are constants.
This is also a famous equation.
Continues...
Excerpted from MESA and Trading Market Cycles by John F. Ehlers Excerpted by permission.
All rights reserved. No part of this excerpt may be reproduced or reprinted without permission in writing from the publisher.
Excerpts are provided by Dial-A-Book Inc. solely for the personal use of visitors to this web site.