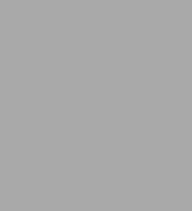
Remote Sensing of Landscapes with Spectral Images: A Physical Modeling Approach
386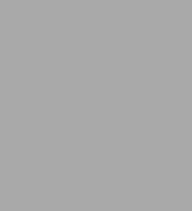
Remote Sensing of Landscapes with Spectral Images: A Physical Modeling Approach
386Paperback(Reprint)
-
SHIP THIS ITEMIn stock. Ships in 1-2 days.PICK UP IN STORE
Your local store may have stock of this item.
Available within 2 business hours
Related collections and offers
Overview
Product Details
ISBN-13: | 9781108462778 |
---|---|
Publisher: | Cambridge University Press |
Publication date: | 03/01/2018 |
Edition description: | Reprint |
Pages: | 386 |
Product dimensions: | 6.69(w) x 9.65(h) x 0.79(d) |
Read an Excerpt
Cambridge University Press
0521662214 - Remote Sensing of Landscapes with Spectral Images - A Physical Modeling Approach - by John B. Adams and Alan R. Gillespie
Excerpt
Chapter 1
Extracting information from spectral images
Image not available in HTML version |
North Cascades, Washington. Photograph by D. O’Callaghan
In this chapter |
Spectral information, when placed in a photo-interpretive context, is a powerful tool for understanding landscapes. We obtain the most reliable spectral information by invoking physical models that link image measurements with materials and processes on the ground. |
1.1 Introduction
Seeing is believing
Landscape |
A portion of the land that can be seen from a single vantage point. |
We are a visual species that relies heavily on images to understand the world around us. The ability of humans to extract information from landscapes through visual images has evolved over millions of years. Of necessity, our hunter–gatherer ancestors learned how to “read” landscapes to find shelter and resources and to avoiddanger. Today, with the increasing demands that are placed on the world’s ecosystems by a growing population, we find ourselves reading landscapes not only to find more resources and to cope with danger, but for signs of strain in our planet’s life-support systems. Ironically, though, our species evolved by paying the most attention to short-term events on local scales. Until now, we have had little need to develop the skills to notice decadal or longer changes or those changes that occur on regional or global scales (e.g., Ehrlich and Ehrlich, 2004). No wonder it is so hard to attract people’s attention to issues of environmental change, such as depletion of ground water, deforestation, expansion of deserts and retreat of glaciers. These, and many other changes, are largely ignored by the world’s increasingly urban population. Out of sight, out of mind.
Aerial photographs and satellite images now allow us to visualize landscapes in entirely new ways. We can see vast areas all at once. We can construct images that measure diverse properties of landscapes, and we can compare images taken at different times to discover changes. The power of these remote measurements lies in the fact that we can relate them to the familiar realm of what we see on the ground. Astonishingly, within a few decades humans have developed the means to visualize the world in ways that our ancestors never dreamed of. And just in time. Now, more than ever, we need to be able to see what is going on in a rapidly changing world where human influences are felt everywhere on the planet. Remote sensing is not just an interesting technical phenomenon, it has become an essential part of an urgent quest to understand our changing environment.
Pretty pictures are not enough
The issues of world resources and environmental change demand society’s best efforts, including our most perceptive and accurate assessments from remote sensing. However, in pursuing this goal, we need to be aware that there is an opposite side to “seeing is believing,” namely that it is easy to be misled by images. In remote sensing, it is all too common to accept images at face value without understanding how they were made and what they really show, especially when they are dramatic and esthetically pleasing. We all think that we know how to interpret images; after all, it comes naturally, right? Well, not really, at least in the case of remote sensing with modern instruments that operate beyond the range of human vision. Depending on our objectives, processing and analyzing spectral images can be a daunting task – one that often is left to technicians to do the hands-on computer work. In our experience, many, perhaps most, of the people who make use of spectral images do not have the time or inclination to analyze the data themselves, and many do not fully understand what happens inside the computer. Even skilled technicians are not immune. Let’s admit it: at heart we are all button-pushers. There is something seductive about clicking on an algorithm and seeing an image appear on the computer screen. With lots of choices of algorithms, it is tempting to try a few to see what happens. Of course, this leaves us vulnerable to generating erroneous or irreproducible interpretations when our real aim is to produce consistently reliable results that help us to solve problems in the field.
One of the best ways to improve the quality and quantity of information that we extract from spectral images is to apply physically based models that connect the spectroscopic and optical properties of known materials with remote measurements. Imaging and spectroscopy were joined in the 1980s for remote-sensing applications when it became technically feasible to acquire simultaneous images in hundreds of narrow wavelengths with spectral resolutions comparable to those of laboratory spectrometers. Spectroscopists were quick to use the new Airborne Imaging Spectrometer (AIS) and Airborne Visible and Infrared Imaging Spectrometer (AVIRIS) to identify materials remotely by the characteristic wavelengths of recognizable absorption bands (Goetz et al., 1985). Actually, though, it has always been possible to apply spectroscopy to spectral images – even to images that sample only a few, broad wavelength regions, such as those acquired by Landsat. Perhaps the best example is the widespread use of vegetation indices such as the normalized-difference vegetation index (NDVI), because these indices are firmly linked to the spectroscopic characteristics of green vegetation. Spectroscopy and physical models form a basis for interpreting all spectral images, regardless of the number and the resolution of the wavelengths sampled. We have powerful visual interpretive skills, and we have powerful analytical tools to interpret spectral data. The challenge is to combine the two in order best to extract information about landscapes.
1.2 Field studies and spectral images
Field work and image analysis |
Both involve sampling the landscape. In field work, a researcher can examine evidence at all scales. In images, the smallest sample of a landscape is determined by the size of the pixel footprint. |
Remotely sensed spectral images are a proxy for being in the field. True, there are important differences, such as not being able to sample materials, viewing outside of the visible range of the eye, and observing large areas at once. But there are important similarities between image analysis and field work. Spectral images and the field are data-rich. Skilled analysts, like field observers, can return again and again to an image and find new information each time. In contrast, as beginners, we are apt to turn away from an image after a few moments with the impression that there is not much there. With experience, however, we learn to ask questions of the data. “What do you want to know?” is equally relevant in the field and with images.
Thematic maps of land cover and land use are fundamental products of field studies (Chapter 7). By focusing on particular topics such as plant species, soils, or geology, field observers are able to sort through the complexities of the natural world to record, organize and interpret relevant information. To maintain focus, the observations and measurements are guided by questions and working hypotheses. Questions determine what to measure. Are there more trees on one part of a hill slope? Is the red soil younger than the brown one? Are the rocks in the distant outcrop the same as the ones we see here? Hypotheses are used to formulate testable ideas about processes that have shaped a landscape. Hypotheses provoke new questions. New questions lead to new observations, measurements and yet more hypotheses.
In the field, we do not expect that the answers to our questions will be revealed everywhere, or at a single spatial scale. We know from experience that critical information, perhaps the presence of a certain type of plant or the evidence for the relative ages of two rock types, is likely to be found in only a few places, and that an essential part of field work is assembling bits of specific, and perhaps scattered, information into a larger and more complete picture. This process is not amenable to a mechanical or automated approach, because the person doing the field work must continually evaluate what is important and what is not. Skilled field workers, therefore, continually make new discoveries and keep seeing a landscape in new ways. Because images are a proxy for being in the field, skilled image interpreters proceed in much the same way.
Color and pattern |
Color enriches our interpretations, but interpretations usually are based on spatial patterns and temporal change. |
Our day-to-day visual observations depend heavily on an ability to derive meaning from the shapes, sizes and textures of the objects and patterns in the world around us. We make relatively little use of spectral information. Color-blind people function quite normally in this visual world of ours, which is a reminder that, although the sensation of color enriches our lives and is essential in some instances, our visual experience is dominated by spatial, not spectral, information. It is natural that we bring this same emphasis to our interpretation of spectral images. Furthermore, most of our visual experience has been gained by viewing the world more-or-less horizontally from a few meters above the ground under ever-changing conditions of illumination. We do our best job of image interpretation with familiar subjects such as other people. Viewing the ground surface from aircraft or satellite altitudes introduces an unfamiliar perspective, significant changes in scale and resolution and, commonly, new subjects such as roof tops and the tops of forest canopies (Figure 1.1).
A change in perspective alters shading and shadowing clues, even to the extent of sometimes causing us to perceive topography as inverted. Nevertheless, with experience, we have learned to interpret patterns in images taken from overhead of extended areas of the land surface. An advantage still belongs to those who have experience in the field and in interpreting landscapes at different scales. For example, if we recognize a moraine from a long-vanished glacier in an image it is because we
Image not available in HTML version |
Figure 1.1. Image series taken north of Manaus, Brazil showing the primary forest at different perspectives, scales and resolutions: (a) from the ground; (b) from a 40-m research tower; (c) from an aircraft 200 m above the ground, showing the top of the tower.
Image not available in HTML version |
Figure 1.2. Oblique aerial photograph of glacial moraine near Bishop, California (see also Figure 1.13). Sinuous, sub-parallel ridges are unsorted debris left behind after alpine glaciers retreated. Moraine is ∼1.5 km across. Photograph by D. Clark.
have seen a similar one before, or we have learned from some source what one looks like (Figure 1.2). If we have never heard of a moraine it is unlikely that we could recognize one, or even notice it. Apparently we compare what we see with many stored patterns (image or otherwise) in
Image not available in HTML version |
Figure 1.3. (a) Panchromatic photograph of a bowl of fruit. (b) Reflectivity spectra of items in the photograph.
the brain until a good match is found; however, when we interpret visual information we invoke a far more complicated process than matching and naming patterns. Interpretation explores the interrelations between patterns, and it involves a variety of judgments as to the significance of features in a broader context. A glaciologist, for example, can visualize the processes that produce moraines, and thus view all of the topography in an image from that perspective.
Spectral and spatial information
Our skills in spectral recognition are underdeveloped compared with our spatial and pattern-recognition abilities. Consider a bowl containing several different kinds of fruit (Figure 1.3). Most of us could recognize the familiar items in a black-and-white photograph by their shapes, sizes, and textures, but we would be less sure of our choices if we only could see swatches of color samples that matched the objects. And very few of us could identify them just using a plot of their spectral reflectance. Experience has taught us to be wary of relying on perceived colors to identify most objects. We know that different materials may have the same colors. Then too, the same material can have different colors, as is shown by fruits and vegetables that change as they ripen. Once we recognize something by shape, size and texture we can use color to gain further information. We tend to do the same thing with color images, that is, to rely heavily on the spatial information and to use the colors occasionally for help. Modern instruments have extended our visual spectral range by orders of magnitude. Until a few decades ago we never had “seen” the world at 1 µm or at 10 µm, nor at any other wavelengths outside the narrow range of about 0.4 to 0.7 µm. We have had to develop our experience from scratch in each new wavelength range, without the benefit of daily practice, to say nothing of millions of years of evolution.
Now that images are not limited to visible wavelengths, the overall availability of spectral information about landscapes has been enlarged enormously, and with it the potential for discriminating and identifying materials on the ground. Still, the typical approach to spectral images has continued to rely heavily on the more familiar spatial information. In addition, few users of remotely sensed images have had training in spectroscopy. As a result, standard, largely empirical methods for analyzing spectral images have evolved, and are the most widely used analytical tools in remote sensing today. Standard methods (Chapter 3) have been used successfully for a wide variety of applications; nevertheless, the spectral content of images has been, and continues to be under-utilized. To extract the maximum amount of information from spectral images we need to understand the physical connection between the data (encoded radiance) and materials on the ground, a topic that we discuss in Section 1.4.
1.3 Photo interpretation of spectral images
1.3.1 Image context
Image context |
The context of an image and the goals of the analyst are crucial factors in extracting useful information from images. |
Context is critically important to be able to interpret spectral images. A rich spatial context already exists for field investigators who want to make use of spectral images. Furthermore, field investigators are likely to approach remote-sensing data with objectives and questions in mind. Photo interpretation is an essential link between field observations and spectral analysis, because it places spectral measurements in a spatial context. For this reason, before unleashing the power of a spectral image-processing program, we should take time to do a careful reconnaissance using our photo-interpretation skills.
Photo interpretation, like learning to ride a bicycle, is not easily described in words. Besides having a strongly experiential component it requires practice. Many people think that they already are good photo interpreters, because they know how to interpret the everyday images formed by the eye–brain system. In the context of remote sensing, however, photo interpretation can be more difficult due to the unfamiliar perspective and scale. Textbooks on photo interpretation generally explain about cameras and photogrammetry, and then give examples of images where the reader either is told what they are seeing or is asked to render an interpretation. Textbook examples are a good way to build experience, but seldom are quite the same as the interpretive problems facing us in our own applications of remote sensing. One way to improve our photo-interpretation skills is to use an hypothesis-testing approach (Section 1.4). It also is helpful to understand the attributes of an image that govern visual perception.
1.3.2 Illumination and viewing geometry
Shadows and shading provide visual information that is needed to understand topography and texture, and being able to interpret topography and texture is important for discriminating and recognizing objects on the ground. To interpret patterns of shadows and shading in images correctly we need to be aware of how a scene is illuminated and viewed. Everyone knows from experience that the land surface varies in appearance during the day depending on Sun azimuth and elevation. Surfaces also vary in appearance depending on the direction from which they are viewed, for example, whether one is looking toward the Sun or away from it. When analyzing an image it is essential to know whether it was taken toward the nadir (straight down) or whether the view of the surface was oblique to the nadir direction. We also would like to know the following for the time the image was acquired:
The direction toward the Sun (azimuth).
The zenith angle (the angle between the direction to the Sun and the normal to the mean global surface).
The Sun-elevation angle (the complement of the zenith angle, providing the orientation of the mean surface of the imaged area is coincident with the mean global surface).
The angular field of view.
Information on Sun azimuth and elevation generally accompany images that are standard products of remote-sensing surveys and programs. Otherwise, the angles can be computed from an ephemeris if the location, date and time are known. The angular field of view of an image depends on the configuration of the sensor system, and, along with the altitude at the time the data were taken, determines the field of view of the image. For public-domain satellites this information is published. The illumination and viewing geometries are relatively simple for nadir-viewing satellites (Figure 1.4); however, these parameters can vary substantially for some satellites, planetary spacecraft and aircraft cameras that view surfaces obliquely.
1.3.3 Shadows and shading
Shadows, shading and inverted topography
By knowing the Sun azimuth we can predict the orientation of shadows that are cast by topographic or other features. The relationship of
Image not available in HTML version |
Figure 1.4. Sketch defining zenith angle, Sun-elevation angle, nadir view and oblique view relative to the mean global surface. Notice that these angles are not measured relative to local topographic surfaces. (a) Moderate zenith angle. This is the most common geometry for remote-sensing images. (b) Large zenith angle, low Sun-elevation angle. Images show strong shadowing, even by low objects, but shadows obstruct view of much of the surface. (c) Zero zenith angle. When the Sun and the observer are close to the same angular position, objects on the ground block the observer’s view of shadows. Sketches are in the “principal plane” that includes the Sun, ground and observer. For simplicity, the angular field of view of the observer or camera is assumed to be zero; and “rays” from the Sun are considered to be parallel.
Image not available in HTML version |
Figure 1.5. Image that may cause the topography to appear inverted when the page is illuminated from the top. Nilosyrtis, Mars. Mars Global Surveyor; by permission of NASA/JPL/MSSS. Rotate the page 180 degrees and look again.
shadows to the objects that cast them is perfectly obvious in many images. The eye–brain system is so practiced at making sense out of shadows and shading that we take the ability for granted. But the same ability plays tricks on us sometimes by inverting topography (Figure 1.5). The main reason for inversion is that we are accustomed to seeing landscapes illuminated from above – a natural consequence of where solar illumination comes from when we are standing on the ground. However, a satellite view of a landscape toward the nadir need not obey this convention. For example, on a winter morning in the northern hemisphere a landscape will be illuminated from the southeast, and, if the image, as is customary, is oriented with north at the top, the illumination will come from the bottom right. For some, the eye–brain system insists that the illumination must actually be coming from the top of the image and, if so, the ridges must then be valleys and vice versa. Sometimes it is possible to change this perception of inversion by looking at the image while keeping in mind the correct Sun azimuth. A more powerful remedy in the case of hard copy is to rotate the image 180°. Obviously, rotation is more difficult with a computer monitor, and analysts have been known to resort to the indignity of inverting themselves. Whatever method we use to solve this problem, it is essential for further analysis of the image to able to see the topography correctly.
Shadows, exposed and hidden
Brightness and lightness |
We use the term “brightness” for illumination sources (e.g., Sun, light bulbs and computer screens); and the term “lightness” to describe reflecting surfaces (e.g., Earth, Moon and hardcopy images). |
Many of the widely used images of Earth (e.g., Landsat, SPOT, air photos) are acquired in mid morning. It is the time when shadows cast by topography and roughness elements are not too long and not too short for optimum photo interpretation of most scenes. (Morning images avoid the clouds that build during the day in many areas, and, therefore are more popular than afternoon images.) Everyone knows that shadows are prominent in images that are taken near sunrise or sunset when the Sun is low in the sky. It is less widely recognized that significant shadowing occurs anytime that the Sun grazes a local surface such as a hillside. Spatially resolved shadows often reveal subtle features of topography and roughness that otherwise would go unnoticed, because small objects can cast large shadows. Low-Sun satellite images of Earth are not familiar to most image analysts, but they are more common for moons and planets. A drawback of low-Sun images is that at extremely low Sun elevations shadows may obscure a significant portion of the surface, resulting in a loss of information. However, when a sensor is directly in line with the surface and the Sun, shadows disappear, because they are hidden behind the objects that cast them. This is the so-called “zero-phase angle” geometry at which images appear “washed out,” because it is difficult or impossible to see topography and roughness (Figures 1.6 and 1.7). Although these conditions make photo interpretation difficult, they are optimum for measuring the spectral properties of surface materials, precisely because the confusing effects of shadows and shading are minimized.
Lightness gradients can occur from one side of an image to another as a result of variations in shadowing that are caused by changes in
© Cambridge University Press