Robust Methods and Asymptotic Theory in Nonlinear Econometrics
This Lecture Note deals with asymptotic properties, i.e. weak and strong consistency and asymptotic normality, of parameter estimators of nonlinear regression models and nonlinear structural equations under various assumptions on the distribution of the data. The estimation methods involved are nonlinear least squares estimation (NLLSE), nonlinear robust M-estimation (NLRME) and non linear weighted robust M-estimation (NLWRME) for the regression case and nonlinear two-stage least squares estimation (NL2SLSE) and a new method called minimum information estimation (MIE) for the case of structural equations. The asymptotic properties of the NLLSE and the two robust M-estimation methods are derived from further elaborations of results of Jennrich. Special attention is payed to the comparison of the asymptotic efficiency of NLLSE and NLRME. It is shown that if the tails of the error distribution are fatter than those of the normal distribution NLRME is more efficient than NLLSE. The NLWRME method is appropriate if the distributions of both the errors and the regressors have fat tails. This study also improves and extends the NL2SLSE theory of Amemiya. The method involved is a variant of the instrumental variables method, requiring at least as many instrumental variables as parameters to be estimated. The new MIE method requires less instrumental variables. Asymptotic normality can be derived by employing only one instrumental variable and consistency can even be proved with out using any instrumental variables at all.
"1021131799"
Robust Methods and Asymptotic Theory in Nonlinear Econometrics
This Lecture Note deals with asymptotic properties, i.e. weak and strong consistency and asymptotic normality, of parameter estimators of nonlinear regression models and nonlinear structural equations under various assumptions on the distribution of the data. The estimation methods involved are nonlinear least squares estimation (NLLSE), nonlinear robust M-estimation (NLRME) and non linear weighted robust M-estimation (NLWRME) for the regression case and nonlinear two-stage least squares estimation (NL2SLSE) and a new method called minimum information estimation (MIE) for the case of structural equations. The asymptotic properties of the NLLSE and the two robust M-estimation methods are derived from further elaborations of results of Jennrich. Special attention is payed to the comparison of the asymptotic efficiency of NLLSE and NLRME. It is shown that if the tails of the error distribution are fatter than those of the normal distribution NLRME is more efficient than NLLSE. The NLWRME method is appropriate if the distributions of both the errors and the regressors have fat tails. This study also improves and extends the NL2SLSE theory of Amemiya. The method involved is a variant of the instrumental variables method, requiring at least as many instrumental variables as parameters to be estimated. The new MIE method requires less instrumental variables. Asymptotic normality can be derived by employing only one instrumental variable and consistency can even be proved with out using any instrumental variables at all.
54.99
In Stock
5
1
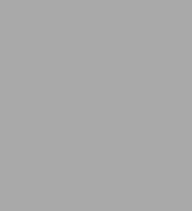
Robust Methods and Asymptotic Theory in Nonlinear Econometrics
198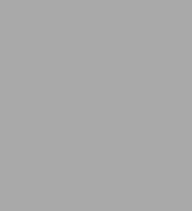
Robust Methods and Asymptotic Theory in Nonlinear Econometrics
198Paperback(Softcover reprint of the original 1st ed. 1981)
$54.99
54.99
In Stock
Product Details
ISBN-13: | 9783540108382 |
---|---|
Publisher: | Springer Berlin Heidelberg |
Publication date: | 07/29/1981 |
Series: | Lecture Notes in Economics and Mathematical Systems , #192 |
Edition description: | Softcover reprint of the original 1st ed. 1981 |
Pages: | 198 |
Product dimensions: | 6.69(w) x 9.61(h) x 0.02(d) |
From the B&N Reads Blog