Research on interior-point methods (IPMs) has dominated the field of mathematical programming for the last two decades. Two contrasting approaches in the analysis and implementation of IPMs are the so-called small-update and large-update methods, although, until now, there has been a notorious gap between the theory and practical performance of these two strategies. This book comes close to bridging that gap, presenting a new framework for the theory of primal-dual IPMs based on the notion of the self-regularity of a function.
The authors deal with linear optimization, nonlinear complementarity problems, semidefinite optimization, and second-order conic optimization problems. The framework also covers large classes of linear complementarity problems and convex optimization. The algorithm considered can be interpreted as a path-following method or a potential reduction method. Starting from a primal-dual strictly feasible point, the algorithm chooses a search direction defined by some Newton-type system derived from the self-regular proximity. The iterate is then updated, with the iterates staying in a certain neighborhood of the central path until an approximate solution to the problem is found. By extensively exploring some intriguing properties of self-regular functions, the authors establish that the complexity of large-update IPMs can come arbitrarily close to the best known iteration bounds of IPMs.
Researchers and postgraduate students in all areas of linear and nonlinear optimization will find this book an important and invaluable aid to their work.
Research on interior-point methods (IPMs) has dominated the field of mathematical programming for the last two decades. Two contrasting approaches in the analysis and implementation of IPMs are the so-called small-update and large-update methods, although, until now, there has been a notorious gap between the theory and practical performance of these two strategies. This book comes close to bridging that gap, presenting a new framework for the theory of primal-dual IPMs based on the notion of the self-regularity of a function.
The authors deal with linear optimization, nonlinear complementarity problems, semidefinite optimization, and second-order conic optimization problems. The framework also covers large classes of linear complementarity problems and convex optimization. The algorithm considered can be interpreted as a path-following method or a potential reduction method. Starting from a primal-dual strictly feasible point, the algorithm chooses a search direction defined by some Newton-type system derived from the self-regular proximity. The iterate is then updated, with the iterates staying in a certain neighborhood of the central path until an approximate solution to the problem is found. By extensively exploring some intriguing properties of self-regular functions, the authors establish that the complexity of large-update IPMs can come arbitrarily close to the best known iteration bounds of IPMs.
Researchers and postgraduate students in all areas of linear and nonlinear optimization will find this book an important and invaluable aid to their work.
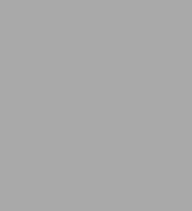
Self-Regularity: A New Paradigm for Primal-Dual Interior-Point Algorithms
208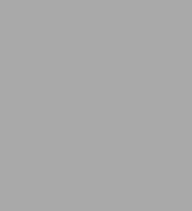
Self-Regularity: A New Paradigm for Primal-Dual Interior-Point Algorithms
208Product Details
ISBN-13: | 9781400825134 |
---|---|
Publisher: | Princeton University Press |
Publication date: | 01/10/2009 |
Series: | Princeton Series in Applied Mathematics , #22 |
Sold by: | Barnes & Noble |
Format: | eBook |
Pages: | 208 |
File size: | 16 MB |
Note: | This product may take a few minutes to download. |