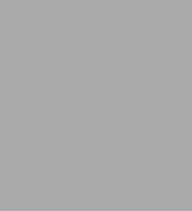
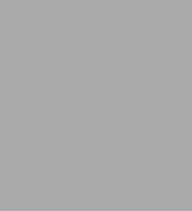
Paperback(5th ed.)
-
PICK UP IN STORECheck Availability at Nearby Stores
Available within 2 business hours
Related collections and offers
Overview
Microsoft Excel offers numerous possibilities for statistical analysis—and you don’t have to be a math wizard to unlock them. In Statistical Analysis with Excel For Dummies, fully updated for the 2021 version of Excel, you’ll hit the ground running with straightforward techniques and practical guidance to unlock the power of statistics in Excel.
Bypass unnecessary jargon and skip right to mastering formulas, functions, charts, probabilities, distributions, and correlations. Written for professionals and students without a background in statistics or math, you’ll learn to create, interpret, and translate statistics—and have fun doing it!
In this book you’ll find out how to:
- Understand, describe, and summarize any kind of data, from sports stats to sales figures
- Confidently draw conclusions from your analyses, make accurate predictions, and calculate correlations
- Model the probabilities of future outcomes based on past data
- Perform statistical analysis on any platform: Windows, Mac, or iPad
- Access additional resources and practice templates through Dummies.com
For anyone who’s ever wanted to unleash the full potential of statistical analysis in Excel—and impress your colleagues or classmates along the way—Statistical Analysis with Excel For Dummies walks you through the foundational concepts of analyzing statistics and the step-by-step methods you use to apply them.
Product Details
ISBN-13: | 9781119844549 |
---|---|
Publisher: | Wiley |
Publication date: | 01/19/2022 |
Edition description: | 5th ed. |
Pages: | 576 |
Sales rank: | 1,046,970 |
Product dimensions: | 7.30(w) x 9.10(h) x 1.30(d) |
About the Author
Read an Excerpt
Statistical Analysis with Excel For Dummies
By Joseph Schmuller
JOHN WILEY & SONS
ISBN: 0-7645-7594-5Chapter One
Evaluating Data in the Real World
In This Chapter
* Introducing statistical concepts
* Generalizing from samples to populations
* Getting into probability
* Making decisions
* Understanding important Excel fundamentals
The field of statistics is all about decision-making - decision-making based on groups of numbers. Statisticians constantly ask questions: What do the numbers tell us? What are the trends? What predictions can we make?
To answer these questions, statisticians have developed an impressive array of analytical tools. These tools help us to make sense of the mountains of data that are out there waiting for us to delve into, and to understand the numbers we generate in the course of our own work.
The Statistical (and Related) Notions You Just Have to Know
Because intensive calculation is often part and parcel of the statistician's toolset, many people have the misconception that statistics is about number crunching. Number crunching is just one small part of the path to sound decisions, however.
By shouldering the number-crunching load, software increases our speed of traveling down that path. Some software packages are specialized for statistical analysis and contain many of the tools that statisticians use. Although not marketed specifically as a statistical package, Excelprovides a number of these tools, which is the reason I wrote this book.
I said that number crunching is a small part of the path to sound decisions. The most important part is the concepts statisticians work with, and that's what I'll talk about for most of the rest of this chapter.
After that, I'll tell you about some important Excel fundamentals.
Samples and populations
On election night, TV commentators routinely predict the outcome of elections before the polls close. Most of the time they're right. How do they do that?
The trick is to interview a sample of voters after they cast their ballots. Assuming the voters tell the truth about whom they voted for, and assuming the sample truly represents the population, network analysts use the sample data to generalize to the population of voters.
This is the job of a statistician - to use the findings from a sample to make a decision about the population from which the sample comes. But sometimes those decisions don't turn out the way the numbers predicted. Flawed preelection polling led to the memorable picture of President Harry Truman holding up a copy of the Chicago Daily Tribune with the famous, but wrong, headline "Dewey Defeats Truman" after the 1948 election. Part of the statistician's job is to express how much confidence he or she has in the decision. Another election-related example speaks to the idea of confidence in a decision. Pre-election polls (again, assuming a representative sample of voters) tell you the percentage of sampled voters who prefer each candidate. The polling organization adds how accurate they believe the polls are. When you hear a newscaster say something like "accurate to within 3 percent," you're hearing a judgment about confidence.
Here's another example. Suppose you've been assigned to find the average reading speed of all fifth-grade children in the U.S., but you haven't got the time or the money to test them all. What would you do?
Your best bet is to take a sample of fifth-graders, measure their reading speeds (in words per minute), and calculate the average of the reading speeds in the sample. You can then use the sample average as an estimate of the population average.
Estimating the population average is one kind of inference that statisticians make from sample data. I discuss inference in more detail in the section "Inferential Statistics."
REMEMBER
Now for some terminology you have to know: Characteristics of a population (like the population average) are called parameters, and characteristics of a sample (like the sample average) are called statistics. When you confine your field of view to samples, your statistics are descriptive. When you broaden your horizons and concern yourself with populations, your statistics are inferential.
REMEMBER
Now for a notation convention you have to know: Statisticians use Greek letters ([mu], [alpha], [rho]) to stand for parameters, and English letters ([bar]x, s, r) to stand for statistics. Figure 1-1 summarizes the relationship between populations and samples, and parameters and statistics.
Variables: Dependent and independent
Simply put, a variable is something that can take on more than one value. (Something that can have only one value is called a constant.) Some variables you might be familiar with are today's temperature, the Dow Jones Industrial Average, your age, and the value of the dollar against the euro.
Statisticians care about two kinds of variables, independent and dependent. Each kind of variable crops up in any study or experiment, and statisticians assess the relationship between them.
For example, imagine a new way of teaching reading that's intended to increase the reading speed of fifth-graders. Before putting this new method into schools, it would be a good idea to test it. To do that, a researcher would randomly assign a sample of fifth-grade students to one of two groups: One group receives instruction via the new method; the other receives instruction via traditional methods. Before and after both groups receive instruction, the researcher measures the reading speeds of all the children in this study. What happens next? I'll get to that in the upcoming section entitled "Inferential Statistics: Testing Hypotheses."
For now, understand that the independent variable here is Method of Instruction. The two possible values of this variable are New and Traditional. The dependent variable is reading speed.
REMEMBER
In general, the idea is to try to find out if changes in the independent variable are associated with changes in the dependent variable.
REMEMBER
In the examples that appear throughout the book, I'll show you how to use Excel to calculate various characteristics of groups of scores. I'd like you to bear in mind that each time I show you a group of scores, I'm really talking about the values of a dependent variable.
Types of data
Data come in four kinds. When you work with a variable, the way you work with it depends on what kind of data it is.
The first variety is called nominal data. If a number is a piece of nominal data, it's just a name. Its value doesn't signify anything. A good example is the number on an athlete's jersey. It's just a way of identifying the athlete and distinguishing him or her from teammates. The number doesn't indicate the athlete's level of skill.
Next comes ordinal data. Ordinal data are all about order, and numbers begin to take on meaning over and above just being identifiers. A higher number indicates the presence of more of a particular attribute than a lower number. One example is Moh's Scale. Used since 1822, it's a scale whose values are 1 through 10. Mineralogists use this scale to rate the hardness of substances. Diamond, rated at 10, is the hardest. Talc, rated at 1, is the softest. A substance that has a given rating can scratch any substance that has a lower rating.
What's missing from Moh's Scale (and from all ordinal data) is the idea of equal intervals and equal differences. The difference between a hardness of 10 and a hardness of 8 is not the same as the difference between a hardness of 6 and a hardness of 4.
Interval data provides equal differences. Fahrenheit temperatures provide an example of interval data. The difference between 60 degrees and 70 degrees is the same as the difference between 80 degrees and 90 degrees.
Here's something that might surprise you about Fahrenheit temperatures: A temperature of 100 degrees is not twice as hot as a temperature of 50 degrees. For ratio statements (twice as much as, half as much as) to be valid, zero has to mean the complete absence of the attribute you're measuring. A temperature of 0 degrees F doesn't mean the absence of heat - it's just an arbitrary point on the Fahrenheit scale.
The last data type, ratio data, includes a meaningful zero point. For temperatures, the Kelvin scale gives us ratio data. One hundred degrees Kelvin is twice as hot as 50 degrees Kelvin. This is because the Kelvin zero point is absolute zero, where all molecular motion (the basis of heat) stops. Another example is a ruler. Eight inches is twice as long as 4 inches. A length of zero means a complete absence of length.
REMEMBER
Any of these types can form the basis for an independent variable or a dependent variable. The analytical tools you use depend on the type of data you're dealing with.
A little probability
When statisticians make decisions, they express their confidence about those decisions in terms of probability. They can never be certain about what they decide. They can only tell you how probable their conclusions are.
So what is probability? The best way to attack this is with a few examples. If you toss a coin, what's the probability that it comes up heads? Intuitively, you know that if the coin is fair, you have a 50-50 chance of heads and a 50-50 chance of tails. In terms of the kinds of numbers associated with probability, that's 1/2.
How about rolling a die (one member of a pair of dice)? What's the probability that you roll a 3? Hmmm ... a die has six faces and one of them is 3, so that ought to be 1/6, right? Right.
Here's one more. You have a standard deck of playing cards. You select one card at random. What's the probability that it's a club? Well, a deck of cards has four suits, so that answer is 1/4.
I think you're getting the picture. If you want to know the probability that an event occurs, figure out how many ways that event can happen and divide by the total number of events that can happen. In each of the three examples, the event we were interested in (head, 3, or club) only happens one way.
Things can get a bit more complicated. When you toss a die, what's the probability that you'll roll a 3 or a 4? Now you're talking about two ways the event you're interested in can occur, so that's (1 + 1)/6 = 2/6 = 1/3. What about the probability of rolling an even number? That has to be 2, 4, or 6, and the probability is (1 + 1 + 1)/6 = 3/6 = 1/2.
On to another kind of probability question. Suppose you roll a die and toss a coin at the same time. What's the probability you roll a 3 and the coin comes up heads? Consider all the possible events that could occur when you roll a die and toss a coin at the same time. Your outcome could be a head and 1-6, or a tail and 1-6. That's a total of 12 possibilities. The head-and-3 combination can only happen one way. So, the answer is 1/12.
In general the formula for the probability that a particular event occurs is
Pr(event) = Number of ways the event can occur/Total number of possible events
I began this section by saying that statisticians express their confidence about their decisions in terms of probability, which is really why I brought up this topic in the first place. This line of thinking leads us to conditional probability - the probability that an event occurs given that some other event occurs. For example, suppose I roll a die, take a look at it (so that you can't see it), and I tell you that I've rolled an even number. What's the probability that I've rolled a 2? Ordinarily, the probability of a 2 is 1/6, but I've narrowed the field. I've eliminated the three odd numbers (1, 3, and 5) as possibilities. In this case, only the three even numbers (2, 4, and 6) are possible, so now the probability of rolling a 2 is 1/3.
Exactly how does conditional probability play into statistical analysis? Read on.
Inferential Statistics: Testing Hypotheses
In advance of doing a study, a statistician draws up a tentative explanation - a hypothesis - as to why the data might come out a certain way. After the study is complete and the sample data are all tabulated, he or she faces the essential decision a statistician has to make - whether or not to reject the hypothesis.
That decision is wrapped in a conditional probability question: What's the probability of obtaining the data, given that this hypothesis is correct? Statistical analysis provides tools to calculate the probability. If the probability turns out to be low, the statistician rejects the hypothesis.
Here's an example. Suppose you're interested in whether or not a particular coin is fair - whether it has an equal chance of coming up heads or tails. To study this issue, you'd take the coin and toss it a number of times - say 100. These 100 tosses make up your sample data. Starting from the hypothesis that the coin is fair, you'd expect that the data in your sample of 100 tosses would show 50 heads and 50 tails.
If it turns out to be 99 heads and one tail, you'd undoubtedly reject the fair coin hypothesis. Why? The conditional probability of getting 99 heads and one tail given a fair coin is very low. Wait a second. The coin could still be fair and you just happened to get a 99-1 split, right? Absolutely. In fact, you never really know. You have to gather the sample data (the results from 100 tosses) and make a decision. Your decision might be right, or it might not.
Juries face this all the time. They have to decide among competing hypotheses that explain the evidence in a trial. (Think of the evidence as data.) One hypothesis is that the defendant is guilty. The other is that the defendant is not guilty. Jury members have to consider the evidence and, in effect, answer a conditional probability question: What's the probability of the evidence given that the defendant is not guilty? The answer to this question determines the verdict.
Null and alternative hypotheses
Consider once again that coin-tossing study I just mentioned. The sample data are the results from the 100 tosses. Before tossing the coin, you might start with the hypothesis that the coin is a fair one, so that you expect an equal number of heads and tails. This starting point is called the null hypothesis. The statistical notation for the null hypothesis is [H.sub.0]. According to this hypothesis, any heads-tails split in the data is consistent with a fair coin. Think of it as the idea that nothing in the results of the study is out of the ordinary.
An alternative hypothesis is possible - that the coin isn't a fair one, and it's loaded to produce an unequal number of heads and tails. This hypothesis says that any heads-tails split is consistent with an unfair coin. The alternative hypothesis is called, believe it or not, the alternative hypothesis. The statistical notation for the alternative hypothesis is [H.sub.1].
With the hypotheses in place, toss the coin 100 times and note the number of heads and tails. If the results are something like 90 heads and 10 tails, it's a good idea to reject [H.sub.0]. If the results are around 50 heads and 50 tails, don't reject [H.sub.0].
Similar ideas apply to the reading-speed example I gave earlier. One sample of children receives reading instruction under a new method designed to increase reading speed, the other learns via a traditional method. Measure the children's reading speeds before and after instruction, and tabulate the improvement for each child. The null hypothesis, [H.sub.0], is that one method isn't different from the other. If the improvements are greater with the new method than with the traditional method - so much greater that it's unlikely that the methods aren't different from one another - reject [H.sub.0]. If they're not, don't reject [H.sub.0].
REMEMBER
Notice that I didn't say "accept [H.sub.0]." The way the logic works, you never accept a hypothesis. You either reject [H.sub.0] or don't reject [H.sub.0].
Notice also that in the coin-tossing example I said around 50 heads and 50 tails. What does around mean? Also, I said if it's 90-10, reject [H.sub.0]. What about 85-15? 80-20? 70-30? Exactly how much different from 50-50 does the split have to be for you to reject [H.sub.0]? In the reading-speed example, how much greater does the improvement have to be to reject [H.sub.0]?
I won't answer these questions now. Statisticians have formulated decision rules for situations like this, and we'll explore those rules throughout the book.
Two types of error
Whenever you evaluate the data from a study and decide to reject [H.sub.0] or to not reject [H.sub.0], you can never be absolutely sure. You never really know what the true state of the world is. In the context of the coin-tossing example, that means you never know for certain if the coin is fair or not. All you can do is make a decision based on the sample data you gather. If you want to be certain about the coin, you'd have to have the data for the entire population of tosses - which means you'd have to keep tossing the coin until the end of time.
(Continues...)
Excerpted from Statistical Analysis with Excel For Dummies by Joseph Schmuller Excerpted by permission.
All rights reserved. No part of this excerpt may be reproduced or reprinted without permission in writing from the publisher.
Excerpts are provided by Dial-A-Book Inc. solely for the personal use of visitors to this web site.
Table of Contents
Introduction 1About This Book 2
What’s New in This Edition 2
What’s New in Excel (Microsoft 365) 3
Foolish Assumptions 3
Icons Used in This Book 4
Where to Go from Here 5
Beyond This Book 5
Part 1: Getting Started With Statistical Analysis With Excel: A Marriage Made In Heaven 7
Chapter 1: Evaluating Data in the Real World 9
The Statistical (and Related) Notions You Just Have to Know 9
Samples and populations 10
Variables: Dependent and independent 11
Types of data 12
A little probability 13
Inferential Statistics: Testing Hypotheses 14
Null and alternative hypotheses 15
Two types of error 16
Some Excel Fundamentals 18
Autofilling cells 22
Referencing cells 25
Chapter 2: Understanding Excel’s Statistical Capabilities 29
Getting Started 30
Setting Up for Statistics 32
Worksheet functions 32
Quickly accessing statistical functions 36
Array functions 38
What’s in a name? An array of possibilities 41
Creating Your Own Array Formulas 50
Using data analysis tools 51
Additional data analysis tool packages 56
Accessing Commonly Used Functions 58
The New Analyze Data Tool 59
Data from Pictures! 60
Part 2: Describing Data 63
Chapter 3: Show-and-Tell: Graphing Data 65
Why Use Graphs? 65
Examining Some Fundamentals 67
Gauging Excel’s Graphics (Chartics?) Capabilities 68
Becoming a Columnist 69
Stacking the Columns 73
Slicing the Pie 74
A word from the wise 76
Drawing the Line 77
Adding a Spark 80
Passing the Bar 82
The Plot Thickens 84
Finding Another Use for the Scatter Chart 88
Chapter 4: Finding Your Center 91
Means: The Lore of Averages 91
Calculating the mean 92
AVERAGE and AVERAGEA 93
AVERAGEIF and AVERAGEIFS 95
TRIMMEAN 99
Other means to an end 100
Medians: Caught in the Middle 102
Finding the median 102
MEDIAN 103
Statistics à la Mode 104
Finding the mode 104
MODE.SNGL and MODE.MULT 104
Chapter 5: Deviating from the Average 107
Measuring Variation 108
Averaging squared deviations: Variance and how to calculate it 108
VAR.P and VARPA 111
Sample variance 113
VAR.S and VARA 114
Back to the Roots: Standard Deviation 114
Population standard deviation 115
STDEV.P and STDEVPA 115
Sample standard deviation 116
STDEV.S and STDEVA 116
The missing functions: STDEVIF and STDEVIFS 117
Related Functions 121
DEVSQ 121
Average deviation 122
AVEDEV 123
Chapter 6: Meeting Standards and Standings 125
Catching Some Z’s 126
Characteristics of z-scores 126
Bonds versus the Bambino 127
Exam scores 128
STANDARDIZE 128
Where Do You Stand? 131
RANK.EQ and RANK.AVG 131
LARGE and SMALL 133
PERCENTILE.INC and PERCENTILE.EXC 134
PERCENTRANK.INC and PERCENTRANK.EXC 137
Data analysis tool: Rank and Percentile 138
Chapter 7: Summarizing It All 141
Counting Out 141
COUNT, COUNTA, COUNTBLANK, COUNTIF, COUNTIFS 141
The Long and Short of It 144
MAX, MAXA, MIN, and MINA 144
Getting Esoteric 145
SKEW and SKEW.P 146
KURT 148
Tuning In the Frequency 150
FREQUENCY 150
Data analysis tool: Histogram 152
Can You Give Me a Description? 154
Data analysis tool: Descriptive Statistics 154
Be Quick About It! 156
Instant Statistics 159
Chapter 8: What’s Normal? 161
Hitting the Curve 161
Digging deeper 162
Parameters of a normal distribution 163
NORM.DIST 165
NORM.INV 167
A Distinguished Member of the Family 168
NORM.S.DIST 169
NORM.S.INV 170
PHI and GAUSS 170
Graphing a Standard Normal Distribution 171
Part 3: Drawing Conclusions From Data 173
Chapter 9: The Confidence Game: Estimation 175
Understanding Sampling Distributions 176
An EXTREMELY Important Idea: The Central Limit Theorem 177
(Approximately) simulating the Central Limit Theorem 178
The Limits of Confidence 183
Finding confidence limits for a mean 183
CONFIDENCE.NORM 186
Fit to a t 187
CONFIDENCE.T 188
Chapter 10: One-Sample Hypothesis Testing 189
Hypotheses, Tests, and Errors 190
Hypothesis Tests and Sampling Distributions 191
Catching Some Z’s Again 193
Z.TEST 196
t for One 197
T.DIST, T.DIST.RT, and T.DIST.2T 198
T.INV and T.INV.2T 200
Visualizing a t-Distribution 201
Testing a Variance 203
CHISQ.DIST and CHISQ.DIST.RT 205
CHISQ.INV and CHISQ.INV.RT 206
Visualizing a Chi-Square Distribution 208
Chapter 11: Two-Sample Hypothesis Testing 211
Hypotheses Built for Two 211
Sampling Distributions Revisited 212
Applying the Central Limit Theorem 213
Z’s once more 215
Data analysis tool: z-Test: Two Sample for Means 216
t for Two 219
Like peas in a pod: Equal variances 220
Like p’s and q’s: Unequal variances 221
T.TEST 222
Data analysis tool: t-Test: Two Sample 223
A Matched Set: Hypothesis Testing for Paired Samples 227
T.TEST for matched samples 228
Data analysis tool: t-Test: Paired Two Sample for Means 230
t-tests on the iPad with StatPlus 232
Testing Two Variances 235
Using F in conjunction with t 237
F.TEST 238
F.DIST and F.DIST.RT 240
F.INV and F.INV.RT 241
Data analysis tool: F-test: Two Sample for Variances 242
Visualizing the F-Distribution 244
Chapter 12: Testing More Than Two Samples 247
Testing More than Two 247
A thorny problem 248
A solution 249
Meaningful relationships 253
After the F-test 254
Data analysis tool: Anova: Single Factor 258
Comparing the means 260
Another Kind of Hypothesis, Another Kind of Test 262
Working with repeated measures ANOVA 262
Getting trendy 264
Data analysis tool: Anova: Two-Factor Without Replication 268
Analyzing trend 271
ANOVA on the iPad 272
ANOVA on the iPad: Another Way 274
Repeated Measures ANOVA on the iPad 277
Chapter 13: Slightly More Complicated Testing 281
Cracking the Combinations 281
Breaking down the variances 282
Data analysis tool: Anova: Two-Factor Without Replication 284
Cracking the Combinations Again 286
Rows and columns 286
Interactions 287
The analysis 288
Data analysis tool: Anova: Two-Factor With Replication 289
Two Kinds of Variables — at Once 292
Using Excel with a Mixed Design 293
Graphing the Results 298
After the ANOVA 300
Two-Factor ANOVA on the iPad 300
Chapter 14: Regression: Linear and Multiple 303
The Plot of Scatter 303
Graphing a line 305
Regression: What a Line! 307
Using regression for forecasting 309
Variation around the regression line 309
Testing hypotheses about regression 311
Worksheet Functions for Regression 317
SLOPE, INTERCEPT, STEYX 318
FORECAST.LINEAR 319
Array function: TREND 319
Array function: LINEST 323
Data Analysis Tool: Regression 325
Working with tabled output 327
Opting for graphical output 329
Juggling Many Relationships at Once: Multiple Regression 330
Excel Tools for Multiple Regression 331
TREND revisited 331
LINEST revisited 333
Regression data analysis tool revisited 336
Regression Analysis on the iPad 338
Chapter 15: Correlation: The Rise and Fall of Relationships 341
Scatterplots Again 341
Understanding Correlation 342
Correlation and Regression 345
Testing Hypotheses about Correlation 347
Is a correlation coefficient greater than zero? 348
Do two correlation coefficients differ? 349
Worksheet Functions for Correlation 350
CORREL and PEARSON 350
RSQ 351
COVARIANCE.P and COVARIANCE.S 352
Data Analysis Tool: Correlation 353
Tabled output 354
Multiple correlation 355
Partial correlation 356
Semipartial correlation 357
Data Analysis Tool: Covariance 358
Using Excel to Test Hypotheses about Correlation 358
Worksheet functions: FISHER, FISHERINV 359
Correlation Analysis on the iPad 360
Chapter 16: It’s About Time 363
A Series and Its Components 363
A Moving Experience 364
Lining up the trend 365
Data analysis tool: Moving Average 365
How to Be a Smoothie, Exponentially 368
One-Click Forecasting 369
Working with Time Series on the iPad 374
Chapter 17: Nonparametric Statistics 379
Independent Samples 380
Two samples: Mann-Whitney U test 380
More than two samples: Kruskal-Wallis one-way ANOVA 382
Matched Samples 383
Two samples: Wilcoxon matched-pairs signed ranks 384
More than two samples: Friedman two-way ANOVA 386
More than two samples: Cochran’s Q 387
Correlation: Spearman’s rS 389
A Heads-Up 391
Part 4: Probability 393
Chapter 18: Introducing Probability 395
What Is Probability? 395
Experiments, trials, events, and sample spaces 396
Sample spaces and probability 396
Compound Events 397
Union and intersection 397
Intersection, again 398
Conditional Probability 399
Working with the probabilities 400
The foundation of hypothesis testing 400
Large Sample Spaces 400
Permutations 401
Combinations 402
Worksheet Functions 403
FACT 403
PERMUT and PERMUTIONA 403
COMBIN and COMBINA 404
Random Variables: Discrete and Continuous 405
Probability Distributions and Density Functions 405
The Binomial Distribution 407
Worksheet Functions 409
BINOM.DIST and BINOM.DIST.RANGE 409
NEGBINOM.DIST 411
Hypothesis Testing with the Binomial Distribution 412
BINOM.INV 413
More on hypothesis testing 414
The Hypergeometric Distribution 415
HYPGEOM.DIST 416
Chapter 19: More on Probability 419
Discovering Beta 419
BETA.DIST 421
BETA.INV 423
Poisson 424
POISSON.DIST 425
Working with Gamma 427
The gamma function and GAMMA 427
The gamma distribution and GAMMA.DIST 428
GAMMA.INV 430
Exponential 431
EXPON.DIST 431
Chapter 20: Using Probability: Modeling and Simulation 433
Modeling a Distribution 434
Plunging into the Poisson distribution 434
Visualizing the Poisson distribution 435
Working with the Poisson distribution 436
Using POISSON.DIST again 437
Testing the model’s fit 437
A word about CHISQ.TEST 440
Playing ball with a model 441
A Simulating Discussion 444
Taking a chance: The Monte Carlo method 444
Loading the dice 444
Data analysis tool: Random Number Generation 445
Simulating the Central limit Theorem 448
Simulating a business 452
Chapter 21: Estimating Probability: Logistic Regression 457
Working Your Way Through Logistic Regression 458
Mining with XLMiner 460
Part 5: The Part of Tens 465
Chapter 22: Ten (12, Actually) Statistical and Graphical Tips and Traps 467
Significant Doesn’t Always Mean Important 467
Trying to Not Reject a Null Hypothesis Has a Number of Implications 468
Regression Isn’t Always Linear 468
Extrapolating Beyond a Sample Scatterplot Is a Bad Idea 469
Examine the Variability Around a Regression Line 469
A Sample Can Be Too Large 470
Consumers: Know Your Axes 470
Graphing a Categorical Variable as a Quantitative Variable Is Just Plain Wrong 471
Whenever Appropriate, Include Variability in Your Graph 472
Be Careful When Relating Statistics Textbook Concepts to Excel 472
It’s Always a Good Idea to Use Named Ranges in Excel 472
Statistical Analysis with Excel on the iPad Is Pretty Good! 473
Chapter 23: Ten Topics (Thirteen, Actually) That Just Don’t Fit Elsewhere 475
Graphing the Standard Error of the Mean 475
Probabilities and Distributions 479
PROB 479
WEIBULL.DIST 479
Drawing Samples 480
Testing Independence: The True Use of CHISQ.TEST 481
Logarithmica Esoterica 484
What is a logarithm? 484
What is e? 486
LOGNORM.DIST 489
LOGNORM.INV 490
Array Function: LOGEST 491
Array Function: GROWTH 494
The logs of Gamma 497
Sorting Data 498
Part 6: Appendices 501
Appendix A: When Your Data Live Elsewhere 503
Appendix B: Tips for Teachers (and Learners) 507
Augmenting Analyses Is a Good Thing 507
Understanding ANOVA 508
Revisiting regression 510
Simulating Data Is Also a Good Thing 512
When All You Have Is a Graph 514
Appendix C: More on Excel Graphics 515
Tasting the Bubbly 515
Taking Stock 516
Scratching the Surface 518
On the Radar 519
Growing a Treemap and Bursting Some Sun 520
Building a Histogram 521
Ordering Columns: Pareto 522
Of Boxes and Whiskers 523
3D Maps 524
Filled Maps 527
Appendix D: The Analysis of Covariance 529
Covariance: A Closer Look 529
Why You Analyze Covariance 530
How You Analyze Covariance 531
ANCOVA in Excel 532
Method 1: ANOVA 533
Method 2: Regression 537
After the ANCOVA 540
And One More Thing 542
Index 545