Structural Equation Modeling and Natural Systems available in Paperback
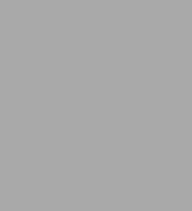
Structural Equation Modeling and Natural Systems
- ISBN-10:
- 0521546532
- ISBN-13:
- 9780521546539
- Pub. Date:
- 08/17/2006
- Publisher:
- Cambridge University Press
- ISBN-10:
- 0521546532
- ISBN-13:
- 9780521546539
- Pub. Date:
- 08/17/2006
- Publisher:
- Cambridge University Press
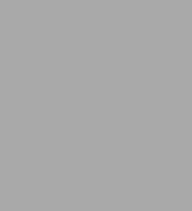
Structural Equation Modeling and Natural Systems
Paperback
Buy New
$77.99Buy Used
$45.72-
-
SHIP THIS ITEM
Temporarily Out of Stock Online
Please check back later for updated availability.
-
Overview
Product Details
ISBN-13: | 9780521546539 |
---|---|
Publisher: | Cambridge University Press |
Publication date: | 08/17/2006 |
Edition description: | New Edition |
Pages: | 378 |
Product dimensions: | 5.91(w) x 8.90(h) x 0.91(d) |
About the Author
Read an Excerpt
Cambridge University Press
0521837421 - Structural Equation Modeling and Natural Systems - by James B. Grace
Excerpt
PART I
A beginning
1
Introduction
The purpose and organization of this book
Structural equation modeling (SEM) represents both a different way of analyzing data, and a different way of doing science. A major theme of this book is that one of the factors that has limited the advance of ecological science has been the absence of methods for developing and evaluating multivariate theories. Understanding systems requires the capacity to examine simultaneous influences and responses. Conventional univariate analyses are typically limited to the examination of a single or at most a few processes at a time. Further, as will be illustrated in this book, characterizing interacting systems using univariate methods is commonly misleading and often inadequate. As I will argue in the final section of the book, conventional univariate hypothesis testing propagates a reliance on “theories of pieces” where one or two interacting processes are presumed to explain major characteristics of natural systems. Single-factor hypotheses seldom provide an adequate representation of system behavior. Worse still, such hypotheses are unable to be placed into a broader context or to evolve into more complex theories, regardless of the empirical evidence. Many of the simplistic theories that have occupiedecologists for so long seem irrelevant when we are faced with the task of predicting the responses of natural systems to environmental change. I believe ecologists have remained focused on univariate questions because we have lacked the scientific tools to ask and answer more complex questions.
Structural equation modeling offers a means of developing and evaluating ideas about complex (multivariate) relationships. It is this property that makes SEM of interest to the practitioner of science. As we shall see in this book, SEM has both tremendous flexibility and significant requirements for proper use. There are also some serious issues relating to how best to use SEM for the study of natural systems. Its mode of application in other fields, such as the social sciences where it has gained a widespread application, may or may not suit our needs. Further, ways of connecting structural equation models with the broader scientific process are needed if we are to gain the maximum impact from our models and analyses. All these issues need to be addressed if SEM is to be applied properly and is to have utility for advancing the study of natural systems.
Before I can discuss fully the potential contributions of SEM to the study of natural systems, we must first have a fairly clear understanding of the principles and practice of SEM. What is its history? What are the underlying statistical principles? How are results from SEM applications to be interpreted? What are the choices to be made and steps to be performed? After such questions have been addressed and the nature of SEM is clear, I will consider its broader significance for the study of natural systems.
To start us on common ground, I begin this chapter with a brief discussion of material that should be familiar to the majority of readers, classic univariate null hypothesis testing. This material will provide a point of comparison for explaining SEM. In this chapter, I will present only a brief and simplistic characterization of SEM, primarily from an historic perspective, while in Chapter 2, I will present an example from an application of SEM to give the reader a tangible illustration.
In Chapter 3, I begin to present some of the fundamental principles of structural equation models, emphasizing their reliance on the fundamental princi- ples of regression. This coverage of basic topics continues through Chapters 4 (latent variables) and 5 (estimation and model evaluation). In Chapter 6, I spend some time presenting a more advanced topic, composite variables, for the dual purposes of illustrating this important capability and also to help clarify the role of latent variables. Chapter 7 provides a very superficial overview of some of the more advanced capabilities of SEM.
Chapters 8 to 11 will emphasize examples of ecological applications to give the reader more of a sense of how the principles can be applied to natural systems. Throughout this section of material, I will contrast the kinds of results obtained from SEM with those obtained from the conventional scientific methods that have guided (and limited) the natural sciences up to this point. Experience suggests that such comparisons are often the most effective means of conveying the potential that SEM has to transform the study of natural systems. This section of chapters will include an illustration of the sustained application of SEM to an ecological problem, the understanding of patterns of plant diversity (in Chapter 10). In Chapter 11, I provide a summary of some cautions as well as a set of recommendations relating to the application of SEM so as to provide all of this practical advice in one place.
In the final section of the book (Chapters 12 and 13), it will be my purpose to give an overall view of the implications of applying SEM to the natural sciences. I will discuss from a philosophical point of view some of the things that I believe have limited the advance of ecological science, and how SEM can lead to a greater maturation of our theories and investigations. Included in this last section will be a discussion of how to integrate SEM into the broader scientific enterprise. Finally, an Appendix provides example applications that illustrate some of the mechanics and logic associated with SEM. The reader will be directed to these examples at appropriate places throughout the book.
An historic point of reference – univariate null hypothesis testing
In the latter half of the nineteenth century, the quantitative sciences began to shift from a deterministic viewpoint to one that recognized the need to address explicitly the concept of probability and error. The story is told by Salsburg (2001) that as scientists began to make more and more precise measurements, they discovered that deviations between calculations and observations became more noticeable, rather than less so. Gradually, the view of a “clockwork” universe in which outcomes are presumed to be deterministic has been replaced by one based on statistics and probabilities. As a result, for most sciences, the process of scientific inquiry has evolved to the point that explicit consideration must be given to issues of error and uncertainty.
Throughout the twentieth century, the acceptance and elaboration of statistical procedures steadily increased to the point that nowadays most scientists are taught basic and even advanced statistical methods as part of their core training. For many areas of inquiry, the very essence of the scientific method has come to require statistical design and analysis. Conclusions are only deemed acceptable if based on accepted statistical conventions (though what is deemed acceptable can vary among disciplines – and among individuals).
In the natural sciences, the fundamental statistical paradigm that is usually taught can be represented by the generalized univariate statistical formula
Display matter not available in HTML version |
Here, y1 refers to an observed set of responses, α1 represents an intercept for the population, Ⅹ refers to some set of independent variables, Γ represents some corresponding vector of coefficients (γs) that empirically link y1 to the elements in Ⅹ (a vector of x variables), and ζ1 represents random errors associated with the responses. Individual values of x and their associated γ1i values (γ1i is the effect of xi on y1) can represent a suite of factors, such as experimental treatments and their interactions, restrictions on randomization due to the experimental or sampling design (e.g., blocking effects), and uncontrolled covariates that influence responses.
Of course, the scientific method does not depend solely on the estimation of parameters, such as those implied by the above equation. It also involves a variety of procedures that are used to draw inferences about the parameter estimates. Associated with the univariate model there has long been a set of conventions and principles for null hypothesis testing that seek to determine whether parameter values are nonzero. These ideas and procedures can in large part be traced back to a combination of procedures and ideas developed by Ronald Fisher, Jerzy Neyman, and Egon Pearson (see Salsburg 2001 for an interesting historical account). The basic approach that is taught typically includes (1) the establishment of a null hypothesis (usually of no difference between groups) which is then compared to an alternative hypothesis, (2) the selection of a significance level (usually 0.05 or 0.01), (3) data collection involving some degree of random sampling, (4) calculation of estimates of means/intercepts and variances (as well as other statistical properties of the populations), and (5) determination of comparative test results and associated probability values. The values of the test statistics obtained are usually represented to be indicative of the probability that observed differences between groups may be due to chance rather than some systematic difference between populations (this is actually an oversimplification of the true interpretation of p-values). In the conventional null hypothesis testing procedure, priority is given to the null hypothesis, which is generally accepted unless there is convincing evidence to the contrary.
Many are aware of long-standing controversies over various aspects of conventional null hypothesis testing. Ronald Fisher, the founding father of much of the early development of modern statistics, as well as the originator of parametric p-values, was himself opposed to the use of fixed cutoffs for standardized hypothesis testing. In spite of this, the codified usage of null hypothesis testing based on predetermined and fixed p-values became entrenched in standardized statistical protocols (this is sometimes referred to as the Neyman–Pearson protocol). There has been a steady drumbeat of complaint about overreliance on null hypothesis tests ever since their introduction (e.g., see Taper and Lele 2004). In most areas of science, this seems to have done little to prevent the establishment of null hypothesis testing as the dominant framework. Perhaps one of the more conspicuous exceptions to this has been in the field of SEM, where priority has been placed on a-priori theory-based models for the past 35 years. The character of this model-based approach will become more apparent as we work through the material in this book. At this point, I wish only to make the point that SEMpractitioners long ago rejected the logical priority of null hypotheses, though the use of p-values continues to be one of the tools used in model evaluation.
It is perhaps useful to note that null hypothesis testing has recently been under attack from several quarters. Biologists have begun to argue more vigorously for a departure from reliance on null hypothesis testing (Anderson et al.2000). The lack of utility of null hypothesis testing has led to recommendations for the use of model selection procedures as an alternative basis for developing inferences. A tenacious effort to expose ecologists to this approach (e.g., Burnham and Anderson 2002) has begun to bring familiarity with these issues to many. At present, these efforts remain focused on univariate models and have not yet tapped into the substantial experiential base of SEM practitioners.
Bayesian methods for estimating parameters and probabilities (Congdon 2001, Gelman et al. 2004) also suggest alternatives to null hypothesis testing. While there are a number of different variants of the Bayesian procedure, the basic concept is that from a personal standpoint, the concept of probability is one that is based on the knowledge available to the investigator. In this framework, empirical evidence is used to update prior probabilities so as to generate posterior probability estimates. This form of probability assessment is preferred by many because it corresponds more directly to the intuitive meaning of probability as a measure of confidence in a result. As will be discussed in the final chapter, Bayesian approaches are now being considered for use in estimation and the evaluation of structural equation models.
What are structural equations?
Let us begin at the beginning. Directly stated, a single univariate equation such as
Display matter not available in HTML version |
representing the effect of a single x on y, can be said to be structural if there exists sufficient evidence from all available sources to support the interpretation that x1 has a causal effect on y1. If information does exist to support a cause and effect interpretation, the parameter γ11 provides an estimate of that effect.
There are certain points that should be made about the seemingly straightforward definition above. One is that the sort of information required to support a causal interpretation for a structural equation is basically the same as that required for a simple regression or an analysis of variance. Thus, we can see that the family of univariate equations represented by Eq. () can be classified as structural equations.
A difficulty for some may arise from the fact that our definition of structural includes the word causal. The average person who is neither a scientist nor a philosopher may be rather surprised to find that scientists and philosophers have historically had some difficulty with the concept of causation. Because of the unease some have with discussing causality, the relationships embodied in structural equations are sometimes referred to as dependencies instead of causes (e.g., “the values of y depend on the values of x”). Thus, an alternative definition that is sometimes seen for structural equations is that they represent statistical dependencies (or statistical associations) that are subject to causal interpretation. What is ultimately most important to realize is that, while the results of structural equation analyses are meant to be reflective of causal dependencies, it is not the statistical results per se that demonstrate causation. Rather, the case for making a causal interpretation depends primarily on prior experience and substantive knowledge.
There has existed over the years an ongoing discussion on the nature of causality and its relationship to structural equations. Perhaps one of the reasons structural equation modeling has been slow to be adopted by biologists has been the priority placed by Fisher on the adage that “correlation does not imply causation”. His emphasis was on manipulative experiments that sought to isolate causes and this approach remains a strong emphasis in biological research. One can see this ongoing debate as a recurring process in which from time to time some feel it wise to caution against overzealous inference of causes. These cautionary periods are typically followed by a general defense of the reasonableness of causal thinking. Fisher’s emphasis was on the development of rigorous experimental protocols designed to isolate individual causes. The emphasis of those who developed structural equation modeling has not been on isolating causes, but instead, on studying simultaneous influences. Both of these scientific goals have merit and, we can think of them as representing the study of individual processes versus the study of system responses.
Some clearly articulated insights into the nature of causality and the relationship to structural equations can be found in Wright (1921) and Bollen (1989), and some of these are described below. Recently, Pearl (2000, Causality) has addressed the issue of causation and structural equations at the level of fundamental logic. A distillation of some of these ideas as they relate to biology can be found in Shipley (2000).
There are a number of arguments that have been made about the tendency for some scientists and philosophers to steer away from using causal language. As Pearl (2000) notes, one reason that some mathematicians have steered away from discussing causation may be the fact that the language of mathematical equations relies most commonly on the symbol “=” to describe the relationships between y and x. As a mathematical operator, the “=” symbol simply represents equality, not dependence; implying that if A = B then B = A. It is not clear to me, that the directional neutrality of the equals sign necessarily causes confusion, because the designation of y variables as dependent and x variables as independent does enable causal directionality to be explicitly specified. Thus, the equation y = x does not possess the criterion of reversibility. Nonetheless, for those who find the language of equations to be insufficiently precise with regard to causality, graphical methods are often preferred as an alternative mathematical language (as we will see below).
According to Pearl, another factor contributing to confusion about the concept of causation is that the word “cause” is not included in the language of probability theory, which is the official mathematical language of statistics. On page 138 he states, “statisticians, the arbiters of respectability, abhor assumptions that are not directly testable [via statistical assessment].”
In this same vein, I suspect that there is a degree to which scientists somehow associate the invocation of causation with a suggestion of “proof”. As Popper (1959) so persuasively stated, it is very difficult to prove anything to be true. Rather, science usually proceeds by falsifying hypotheses and accepting only those that remain.
Alternatively, for some, a statement of causal interpretation may imply “ultimate” causation. This is a reflection of mechanistic thinking that generally matches poorly with inference based on statistical relationships. Rather, such thinking reflects the Aristotelean tradition of determinism, which fails to adequately reflect probabilistic causation and the degree of uncertainty that is associated with measurement.
As we shall see, structural equation modeling does not start with a null hypothesis that is given priority. Rather, structural equation models are built upon the complete body of available knowledge. Models are only rejected if the observed data do not match expectations derived from the model. This “model-oriented” philosophy facilitates the invocation of causal interpretation because it automatically incorporates the a-priori knowledge available to the scientist. This forms the basis for cause and effect interpretations. Stated in a different way, if a researcher intends to draw interpretations about x having an effect on y using structural equations, they should be prepared to defend that supposition with something other than their statistical results.
Criteria for supporting an assumption of causal relationships in structural equations
A causal interpretation of a structural equation, such as y1 = ɑ1 + γ11x1 + ζ1(Eq. 1.2) can be supported if it can be assumed that a sufficient manipulation of x would result in a subsequent change in the values of y, independent of influences from any other factors (Pearl 2000). This criterion might be referred to as the “intervention outcome assumption”. One obvious set of conditions that would support such an assumption would be if previous manipulations had shown such a result. Thus, the assumption that plant growth would be affected by a manipulation of water supply can, under many circumstances, be supported by prior experience. How many times do we have to show through manipulations that plant growth can respond to watering before this effect comes to be expected? This is not to say that a response in plant growth would be expected for all possible additions of water supply. Rather, the assumption being made is that if (1) there is an observed correlation between water supply and plant growth, (2) the supply of additional water occurs prior to the growth response, (3) other independent influences on growth are not simultaneously changing, and (4) an interpretation of plant growth responding to water supply is consistent with known mechanisms, then a causal interpretation of the relationship is reasonable. Of course, when structural equations are used in conjunction with manipulative experiments, the criteria for establishing causality are automatically established if the manipulations are unambiguous.
We must recognize that absolute certainty (or even absolute confidence) is not a requirement for reporting scientific results. At times we may have a stronger or weaker case in support of a causal interpretation. A great deal will have to do with prior experience with the system under investigation. I will have more to say about this subject later as it pertains specifically to structural equation modeling. My position on this is basically consistent with Fisher’s ideas about hypothesis testing, we should not expect to rely on single studies to draw general conclusions. Rather, the process of establishing a confident interpretation comes from the process of investigation.
For now, it must suffice for us to recognize that any claim for a causal interpretation will, as always, have to be defended. At the risk of being repetitive, the requirements for supporting causal interpretations are not fundamentally different from the ones natural scientists already use. A useful discussion of criteria that can be used to evaluate the plausibility of claims based on statistical results can be found in Abelson (1995).
© Cambridge University Press