The Practice of Statistics for Business and Economics / Edition 4 available in Hardcover
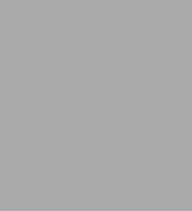
The Practice of Statistics for Business and Economics / Edition 4
- ISBN-10:
- 1464125643
- ISBN-13:
- 9781464125645
- Pub. Date:
- 11/15/2015
- Publisher:
- Freeman, W. H. & Company
- ISBN-10:
- 1464125643
- ISBN-13:
- 9781464125645
- Pub. Date:
- 11/15/2015
- Publisher:
- Freeman, W. H. & Company
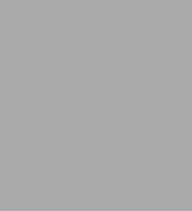
The Practice of Statistics for Business and Economics / Edition 4
Hardcover
Buy New
$194.11Buy Used
$210.19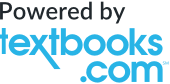
-
SHIP THIS ITEM— Not Eligible for Free Shipping
-
PICK UP IN STORECheck Availability at Nearby Stores
Available within 2 business hours
-
SHIP THIS ITEM
Temporarily Out of Stock Online
Please check back later for updated availability.
Overview
Product Details
ISBN-13: | 9781464125645 |
---|---|
Publisher: | Freeman, W. H. & Company |
Publication date: | 11/15/2015 |
Edition description: | Fourth Edition |
Pages: | 880 |
Product dimensions: | 7.90(w) x 10.10(h) x 1.40(d) |
About the Author
David S. Moore is Shanti S. Gupta Distinguished Professor of Statistics, Emeritus, at Purdue University and was 1998 president of the American Statistical Association. He received his A.B. from Princeton and his Ph.D. from Cornell, both in mathematics. He has written many research papers in statistical theory and served on the editorial boards of several major journals. Professor Moore is an elected fellow of the American Statistical Association and of the Institute of Mathematical Statistics and an elected member of the International Statistical Institute. He has served as program director for statistics and probability at the National Science Foundation. In recent years, Professor Moore has devoted his attention to the teaching of statistics. He was the content developer for the Annenberg/Corporation for Public Broadcasting college-level telecourse Against All Odds: Inside Statistics and for the series of video modules Statistics: Decisions through Data, intended to aid the teaching of statistics in schools. He is the author of influential articles on statistics education and of several leading texts. Professor Moore has served as president of the International Association for Statistical Education and has received the Mathematical Association of America’s national award for distinguished college or university teaching of mathematics.
George P. McCabe is the Associate Dean for Academic Affairs in the College of Science and a Professor of Statistics at Purdue University. In 1966, he received a B.S. degree in mathematics from Providence College, and in 1970 a Ph.D. in mathematical statistics from Columbia University. His entire professional career has been spent at Purdue with sabbaticals at Princeton, the Commonwealth Scientific and Industrial Research Organization in Melbourne (Australia); the University of Berne (Switzerland); the National Institute of Standards and Technology (Boulder, Colorado); and the National University of Ireland in Galway. Professor McCabe is an elected fellow of the American Association for the Advancement of Science and of the American Statistical Association; he was 1998 Chair of its section on Statistical Consulting. From 2008 to 2010, he served on the Institute of Medicine Committee on Nutrition Standards for the National School Lunch and Breakfast Programs. He has served on the editorial boards of several statistics journals, has consulted with many major corporations, and has testified as an expert witness on the use of statistics.
Professor McCabe’s research has focused on applications of statistics. Much of his recent work has been on problems of nutrition, including nutrient requirements, calcium metabolism, and bone health. He is author or coauthor of more than 160 publications in many different journals.
Layth C. Alwan in Associate Professor of Business Statistics and Operations Management, Sheldon B. Lubar School of Business, University of Wisconsin–Milwaukee. He received a B.A. in mathematics, B.S. in statistics, M.B.A., and PhD in business statistics/operations management, all from the University of Chicago, and an M.S. in computer science from DePaul University. Professor Alwan is an author of many research articles related to statistical process control and business forecasting. He has consulted for many leading companies on statistical issues related to quality, forecasting, and operations/supply chain management applications. On the teaching front, he is focused on engaging and motivating business students on how statistical thinking and data analysis methods have practical importance in business. He is the recipient of several teaching awards, including Business School Teacher of the Year and Executive M.B.A. Outstanding Teacher of the Year.
Bruce A. Craig is Professor of Statistics and Director of the Statistical Consulting Service at Purdue University. He received his B.S. in mathematics and economics from Washington University in St. Louis and his PhD in statistics from the University of Wisconsin–Madison. He is an active member of the American Statistical Association and was chair of its section on Statistical Consulting in 2009. He also is an active member of the Eastern North American Region of the International Biometrics Society and aws elected by the voting membership to the Regional Committee from 2003 to 2006. Professor Craig has served on the editorial board of several statistical journals and has been a member of several data and safety monitoring boards, including Purdue's IRB. Professor Craig's research interests focus on the development of novel statistical methodology to address research questions in the life sciences. Areas of current interest are protein structure determination, diagnostic testing, and animal abundance estimation. In 2005, he was named Purdue University Faculty Scholar.
Table of Contents
To Instructors: About This BookSaplingPlus for Statistics
To Students: What Is Statistics?
Index of Cases
Index of Data Tables
Beyond the Basics Index
About the Authors
CHAPTER 1 Examining Distributions
Introduction
1.1 Data
SECTION 1.1 SUMMARY
SECTION 1.1 EXERCISES
1.2 Displaying Distributions with Graphs
Categorical variables: bar graphs and pie charts
Quantitative variables: histograms
CASE 1.1 Treasury Bills
Quantitative variables: stemplots
Interpreting histograms and stemplots
Timeplots
SECTION 1.2 SUMMARY
SECTION 1.2 EXERCISES
1.3 Describing Distributions with Numbers
CASE 1.2 Time to Start a Business
Measuring center: the mean
Measuring center: the median
Comparing the mean and the median
Measuring spread: the quartiles
The five-number summary and boxplots
The 1.5 × IQR rule for outliers
Measuring spread: the standard deviation
Choosing measures of center and spread
BEYOND THE BASICS: Risk and return
SECTION 1.3 SUMMARY
SECTION 1.3 EXERCISES
1.4 Density Curves and the Normal Distributions
Density curves
The median and mean of a density curve
Normal distributions
The 68–95–99.7 rule
The standard Normal distribution
Normal distribution calculations
Using the standard Normal table
Inverse Normal calculations
Assessing the Normality of data
BEYOND THE BASICS: Density estimation
SECTION 1.4 SUMMARY
SECTION 1.4 EXERCISES
CHAPTER 1 REVIEW EXERCISES
CHAPTER 2 Examining Relationships
Introduction
2.1 Scatterplots
CASE 2.1 Education Expenditures and Population: Benchmarking
Interpreting scatterplots
The log transformation
Adding categorical variables to scatterplots
SECTION 2.1 SUMMARY
SECTION 2.1 EXERCISES
2.2 Correlation
The correlation r
Facts about correlation
SECTION 2.2 SUMMARY
SECTION 2.2 EXERCISES
2.3 Least-Squares Regression
The least-squares regression line
Facts about least-squares regression
Interpretation of r^2
Residuals
The distribution of the residuals
Influential observations
SECTION 2.3 SUMMARY
SECTION 2.3 EXERCISES
2.4 Cautions about Correlation and Regression
Extrapolation
Lurking variables
Correlation does not imply causation
BEYOND THE BASICS: Big Data
SECTION 2.4 SUMMARY
SECTION 2.4 EXERCISES
2.5 Data Analysis for Two-Way Tables
CASE 2.2 Does the Right Music Sell the Product?
Marginal distributions
Conditional distributions
Mosaic plots and software output
Simpson’s paradox
SECTION 2.5 SUMMARY
SECTION 2.5 EXERCISES
CHAPTER 2 REVIEW EXERCISES
CHAPTER 3 Producing Data
Introduction
3.1 Sources of Data
Anecdotal data
Available data
Sample surveys and experiments
Other sources and uses of data
SECTION 3.1 SUMMARY
SECTION 3.1 EXERCISES
3.2 Designing Samples
Simple random samples
Stratified samples
Multistage samples
Cautions about sample surveys
BEYOND THE BASICS: Capture-recapture sampling
SECTION 3.2 SUMMARY
SECTION 3.2 EXERCISES
3.3 Designing Experiments
Comparative experiments
Randomized comparative experiments
Completely randomized designs
How to randomize
The logic of randomized comparative experiments
Cautions about experimentation
Matched pairs designs
Block designs
SECTION 3.3 SUMMARY
SECTION 3.3 EXERCISES
3.4 Data Ethics
Institutional review boards
Informed consent
Confidentiality
Clinical trials
Behavioral and social science experiments
SECTION 3.4 SUMMARY
SECTION 3.4 EXERCISES
CHAPTER 3 REVIEW EXERCISES
CHAPTER 4 Probability: The Study of Randomness
Introduction
4.1 Randomness
The language of probability
Thinking about randomness and probability
SECTION 4.1 SUMMARY
SECTION 4.1 EXERCISES
4.2 Probability Models
Sample spaces
Probability rules
Assigning probabilities: Finite number of outcomes
CASE 4.1 Uncovering Fraud by Digital Analysis
Assigning probabilities: Equally likely outcomes
Independence and the multiplication rule
Applying the probability rules
SECTION 4.2 SUMMARY
SECTION 4.2 EXERCISES
4.3 General Probability Rules
General addition rules
Two-way table of counts to probabilities
Conditional probability
General multiplication rule
Tree diagrams
Bayes’ rule
Independence again
SECTION 4.3 SUMMARY
SECTION 4.3 EXERCISES
CHAPTER 4 REVIEW EXERCISES
CHAPTER 5 Random Variables and Probability Distributions
Introduction
5.1 Random Variables
Discrete random variables
CASE 5.1 Tracking Perishable Demand
Continuous random variables
SECTION 5.1 SUMMARY
SECTION 5.1 EXERCISES
5.2 Means and Variances of Random Variables
The mean of a random variable
Mean and the law of large numbers
Thinking about the law of large numbers
Rules for means
CASE 5.2 Portfolio Analysis
The variance of a random variable
Rules for variances and standard deviations
SECTION 5.2 SUMMARY
SECTION 5.2 EXERCISES
5.3 Common Discrete Distributions
Binomial distributions
The binomial distributions for sample counts
The binomial distributions for statistical sampling
CASE 5.3 Inspecting a Supplier’s Products
Finding binomial probabilities
Binomial formula
Binomial mean and standard deviation
Assessing the binomial assumptions with data
Poisson distributions
The Poisson setting
The Poisson model
Poisson approximation of the binomial
Assessing the Poisson assumptions with data
SECTION 5.3 SUMMARY
SECTION 5.3 EXERCISES
5.4 Common Continuous Distributions
Uniform distributions
Revisiting Normal distributions
Normal approximation for binomial distribution
The continuity correction
Normal approximation for Poisson distribution
Exponential distributions
SECTION 5.4 SUMMARY
SECTION 5.4 EXERCISES
CHAPTER 5 REVIEW EXERCISES
CHAPTER 6 Sampling Distributions
Introduction
6.1 Toward Statistical Inference
Sampling variability
Sampling distributions
Bias and variability in estimation
Sampling from large populations
Why randomize?
SECTION 6.1 SUMMARY
SECTION 6.1 EXERCISES
6.2 The Sampling Distribution of the Sample Mean
The mean and standard deviation of x
The central limit theorem
How large is large enough?
Two more facts
SECTION 6.2 SUMMARY
SECTION 6.2 EXERCISES
6.3 The Sampling Distribution of the Sample Proportion
Sample proportion mean and standard deviation
Normal approximation for proportions
SECTION 6.3 SUMMARY
SECTION 6.3 EXERCISES
CHAPTER 6 REVIEW EXERCISES
CHAPTER 7 Introduction to Inference
Introduction
Overview of inference
7.1 Estimating with Confidence
Statistical confidence
Confidence intervals
Confidence interval for a population mean
CASE 7.1 Bankruptcy Attorney Fees
How confidence intervals behave
Some cautions
SECTION 7.1 SUMMARY
SECTION 7.1 EXERCISES
7.2 Tests of Significance
The reasoning of significance tests
CASE 7.2 Fill the Bottles
Step 1: Stating the hypotheses
Step 2: Calculating the value of a test statistic
Step 3: Finding the P-value
Step 4: Stating a conclusion
Summary of the z test for one population mean
Two-sided significance tests and confidence intervals
Assessing significance with P-values versus critical values
SECTION 7.2 SUMMARY
SECTION 7.2 EXERCISES
7.3 Use and Abuse of Tests
Choosing a level of significance
Statistical significance does not imply practical significance
Statistical inference is not valid for all sets of data
Beware of searching for significance
SECTION 7.3 SUMMARY
SECTION 7.3 EXERCISES
7.4 Prediction Intervals
Concept of random deviations
Prediction of a single observation
SECTION 7.4 SUMMARY
SECTION 7.4 EXERCISES
CHAPTER 7 REVIEW EXERCISES
CHAPTER 8 Inference for Means
Introduction
8.1 Inference for the Mean of a Population t distributions
The one-sample t confidence interval
CASE 8.1 Battery Life of a Smartphone
The one-sample t test
Using software
Matched pairs t procedures
Robustness of the one-sample t procedures
Inference for non-Normal populations
BEYOND THE BASICS: The bootstrap
SECTION 8.1 SUMMARY
SECTION 8.1 EXERCISES
8.2 Comparing Two Means
The two-sample t statistic
The two-sample t confidence interval
The two-sample t significance test
Robustness of the two-sample procedures
Inference for small samples
The pooled two-sample t procedures
CASE 8.2 Active Versus Failed Retail Companies
SECTION 8.2 SUMMARY
SECTION 8.2 EXERCISES
8.3 Additional Topics on Inference
Sample size for confidence intervals
Power of a significance test
Inference as a decision
SECTION 8.3 SUMMARY
SECTION 8.3 EXERCISES
CHAPTER 8 REVIEW EXERCISES
CHAPTER 9 One-Way Analysis of Variance
Introduction
9.1 One-Way Analysis of Variance
The ANOVA setting
Comparing means
Revisiting the pooled two-sample t statistic
An overview of ANOVA
CASE 9.1 Tip of the Hat and Wag of the Finger?
The ANOVA model
Estimates of population parameters
Testing hypotheses in one-way ANOVA
The ANOVA table
The F test
Using software
BEYOND THE BASICS: Testing the equality of spread
SECTION 9.1 SUMMARY
SECTION 9.1 EXERCISES
9.2 Additional Comparisons of Group Means
Contrasts
CASE 9.2 Evaluation of a New Educational Product
Multiple comparisons
Simultaneous confidence intervals
Assessing the power of the ANOVA F test
SECTION 9.2 SUMMARY
SECTION 9.2 EXERCISES
CHAPTER 9 REVIEW EXERCISES
CHAPTER 10 Inference for Proportions
Introduction
10.1 Inference for a Single Proportion
CASE 10.1 Trends in the Workplace
Large-sample confidence interval for a single proportion
BEYOND THE BASICS: Plus four confidence interval for a single proportion
Significance test for a single proportion
Choosing a sample size for a confidence interval
CASE 10.2 Marketing Christmas Trees
Choosing a sample size for a significance test
SECTION 10.1 SUMMARY
SECTION 10.1 EXERCISES
10.2 Comparing Two Proportions
Large-sample confidence intervals for a difference in proportions
CASE 10.3 Social Media in the Supply Chain
BEYOND THE BASICS: Plus four confidence intervals for a difference in proportions
Significance tests
Choosing a sample size for two sample proportions
BEYOND THE BASICS: Relative risk
SECTION 10.2 SUMMARY
SECTION 10.2 EXERCISES
CHAPTER 10 REVIEW EXERCISES
CHAPTER 11 Inference for Categorical Data
Introduction
11.1 Inference for Two-Way Tables
Two-way tables
CASE 11.1 Are Flexible Companies More Competitive?
Describing relations in two-way tables
The null hypothesis: no association
Expected cell counts
The chi-square test
The chi-square test and the z test
Models for two-way tables
BEYOND THE BASICS: Meta-analysis
SECTION 11.1 SUMMARY
SECTION 11.1 EXERCISES
11.2 Goodness of Fit
SECTION 11.2 SUMMARY
SECTION 11.2 EXERCISES
CHAPTER 11 REVIEW EXERCISES
CHAPTER 12 Inference for Regression
Introduction
12.1 Inference about the Regression Model
Statistical model for simple linear regression
From data analysis to inference
CASE 12.1 The Relationship between Income and Education for Entrepreneurs
Estimating the regression parameters
Conditions for regression inference
Confidence intervals and significance tests
The word “regression”
Inference about correlation
SECTION 12.1 SUMMARY
SECTION 12.1 EXERCISES
12.2 Using the Regression Line
Confidence and prediction intervals
BEYOND THE BASICS: Nonlinear regression
SECTION 12.2 SUMMARY
SECTION 12.2 EXERCISES
12.3 Some Details of Regression Inference
Standard errors
Analysis of variance for regression
SECTION 12.3 SUMMARY
SECTION 12.3 EXERCISES
CHAPTER 12 REVIEW EXERCISES
CHAPTER 13 Multiple Regression
Introduction
13.1 Data Analysis for Multiple Regression
Using a linear model with multiple variables
CASE 13.1 The Inclusive Development Index (IDI)
Data for multiple regression
Preliminary data analysis for multiple regression
Estimating the multiple regression coefficients
Regression residuals
The regression standard error
SECTION 13.1 SUMMARY
SECTION 13.1 EXERCISES
13.2 Inference for Multiple Regression
Multiple linear regression model
CASE 13.2 Predicting Movie Revenue
Estimating the parameters of the model
Inference about the regression coefficients
Inference about prediction
ANOVA table for multiple regression
Squared multiple correlation R^2
Inference for a collection of regression coefficients
SECTION 13.2 SUMMARY
SECTION 13.2 EXERCISES
13.3 Multiple Regression Model Building
CASE 13.3 Prices of Homes
Models for curved relationships
Models with categorical explanatory variables
More elaborate models
Variable selection methods
BEYOND THE BASICS: Regression trees
SECTION 13.3 SUMMARY
SECTION 13.3 EXERCISES
CHAPTER 13 REVIEW EXERCISES
CHAPTER 14 Time Series Forecasting
Introduction
Overview of Time Series Forecasting
14.1 Assessing Time Series Behavior
CASE 14.1 Adidas Stock Price Returns
Random process model
Nonrandom processes
CASE 14.2 Amazon Sales
Runs test
Autocorrelation function
Forecasts of a random process
SECTION 14.1 SUMMARY
SECTION 14.1 EXERCISES
14.2 Random Walks
Price changes versus returns
Deterministic and stochastic trends
BEYOND THE BASICS: Dickey-Fuller tests
SECTION 14.2 SUMMARY
SECTION 14.2 EXERCISES
14.3 Basic Smoothing Models
Moving-average models
CASE 14.3 Lake Michigan Water Levels
Forecasting accuracy
Moving average and seasonal indexes
Exponential smoothing models
SECTION 14.3 SUMMARY
SECTION 14.3 EXERCISES
14.4 Regression-Based Forecasting Models
Modeling deterministic trends
Modeling seasonality
Residual checking
Modeling with lagged variables
BEYOND THE BASICS: ARCH models
SECTION 14.4 SUMMARY
SECTION 14.4 EXERCISES
CHAPTER 14 REVIEW EXERCISES
Tables T-1
Answers to Odd-Numbered Exercises S-1
Index I-1
The following optional Companion Chapters can be found online at www.macmillanhighered.com/psbe5e.
CHAPTER 15 Statistics for Quality: Control and Capability
Introduction
Quality overview
Systematic approach to process improvement
Process improvement toolkit
15.1 Statistical Process Control
SECTION 15.1 SUMMARY
SECTION 15.1 EXERCISES
15.2 Variable Control Charts
x and R charts
CASE 15.1 Turnaround Time for Lab Results
CASE 15.2 O-Ring Diameters
x and s charts
Assumptions underlying subgroup charts
Charts for individual observations
SECTION 15.2 SUMMARY
SECTION 15.2 EXERCISES
15.3 Process Capability Indices
SECTION 15.3 SUMMARY
SECTION 15.3 EXERCISES
15.4 Attribute Control Charts
Control charts for sample proportions
CASE 15.3 Reducing Absenteeism
Control charts for counts per unit of measure
SECTION 15.4 SUMMARY
SECTION 15.4 EXERCISES
CHAPTER 15 REVIEW EXERCISES
CHAPTER 16 Two-Way Analysis of Variance
Introduction
16.1 The Two-Way ANOVA Model
Advantages of two-way ANOVA
The two-way ANOVA model
Main effects and interactions
SECTION 16.1 SUMMARY
SECTION 16.1 EXERCISES
16.2 Inference for Two-Way ANOVA
The ANOVA table for two-way ANOVA
Carrying out a two-way ANOVA
CASE 16.1 Discounts and Expected Prices
CASE 16.2 Expected Prices, Continued
SECTION 16.2 SUMMARY
SECTION 16.2 EXERCISES
CHAPTER 16 REVIEW EXERCISES
CHAPTER 17 Nonparametric Tests
Introduction
17.1 The Wilcoxon Rank Sum Test
CASE 17.1 Price Discrimination?
The rank transformation
The Wilcoxon rank sum test
The Normal approximation
What hypotheses do the Wilcoxon test?
Ties
CASE 17.2 Consumer Perceptions of Food Safety
Rank versus t tests
SECTION 17.1 SUMMARY
SECTION 17.1 EXERCISES
17.2 The Wilcoxon Signed Rank Test
The Normal approximation
Ties
SECTION 17.2 SUMMARY
SECTION 17.2 EXERCISES
17.3 The Kruskal-Wallis Test
Hypotheses and assumptions
The Kruskal-Wallis test
SECTION 17.3 SUMMARY
SECTION 17.3 EXERCISES
CHAPTER 17 REVIEW EXERCISES
CHAPTER 18 Logistic Regression
Introduction
18.1 The Logistic Regression Model
CASE 18.1 Clothing Color and Tipping
Binomial distributions and odds
Model for logistic regression
Fitting and interpreting the logistic regression model
SECTION 18.1 SUMMARY
SECTION 18.1 EXERCISES
18.2 Inference for Logistic Regression
Examples of logistic regression analyses
SECTION 18.2 SUMMARY
SECTION 18.2 EXERCISES
18.3 Multiple Logistic Regression
SECTION 18.3 SUMMARY
SECTION 18.3 EXERCISES
CHAPTER 18 REVIEW EXERCISES