Topics in Structural VAR Econometrics
In recent years a growing interest in the structural V AR approach (SV AR) has followed the path-breaking works by Blanchard and Watson (1986), Bernanke (1986) and Sims (1986), especially in the U.S. applied macroeconometric literature. The approach can be used in two different, partially overlapping, directions: the interpretation of business cycle fluctuations of a small number of significant macroeconomic variables and the identification of the effects of different policies. SV AR literature shows a common feature: the attempt to "organise", in a "structural" theoretical sense, instantaneous correlations among the relevant variables. In non-structural V AR modelling, instead, correlations are normally hidden in the variance covariance matrix of the V AR model innovations. of independent V AR analysis tries to isolate ("identify") a set shocks by means of a number of meaningful theoretical restrictions. The shocks can be regarded as the ultimate source of shastic variation of the vector of variables which can all be seen as potentially endogenous. Looking at the development of SV AR literature we felt that it still lacked a formal general framework which could embrace the several types of models so far proposed for identification and estimation. This is the second edition of the book, which originally appeared as number 381 of the Springer series "Lecture notes in Economics of the first edition was Carlo and Mathematical Systems". The author Giannini.
"1002443810"
Topics in Structural VAR Econometrics
In recent years a growing interest in the structural V AR approach (SV AR) has followed the path-breaking works by Blanchard and Watson (1986), Bernanke (1986) and Sims (1986), especially in the U.S. applied macroeconometric literature. The approach can be used in two different, partially overlapping, directions: the interpretation of business cycle fluctuations of a small number of significant macroeconomic variables and the identification of the effects of different policies. SV AR literature shows a common feature: the attempt to "organise", in a "structural" theoretical sense, instantaneous correlations among the relevant variables. In non-structural V AR modelling, instead, correlations are normally hidden in the variance covariance matrix of the V AR model innovations. of independent V AR analysis tries to isolate ("identify") a set shocks by means of a number of meaningful theoretical restrictions. The shocks can be regarded as the ultimate source of shastic variation of the vector of variables which can all be seen as potentially endogenous. Looking at the development of SV AR literature we felt that it still lacked a formal general framework which could embrace the several types of models so far proposed for identification and estimation. This is the second edition of the book, which originally appeared as number 381 of the Springer series "Lecture notes in Economics of the first edition was Carlo and Mathematical Systems". The author Giannini.
109.99
In Stock
5
1
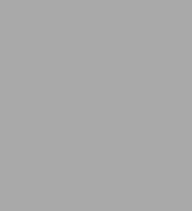
Topics in Structural VAR Econometrics
181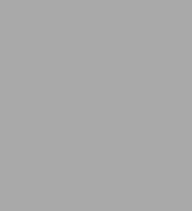
Topics in Structural VAR Econometrics
181Paperback(2nd ed. 1997. Softcover reprint of the original 2nd ed. 1997)
$109.99
109.99
In Stock
Product Details
ISBN-13: | 9783642644818 |
---|---|
Publisher: | Springer Berlin Heidelberg |
Publication date: | 09/18/2011 |
Edition description: | 2nd ed. 1997. Softcover reprint of the original 2nd ed. 1997 |
Pages: | 181 |
Product dimensions: | 5.90(w) x 9.00(h) x 0.50(d) |
From the B&N Reads Blog